MGDTI: Graph Transformer with Meta-Learning for Drug-Target Interaction Prediction.
2023 IEEE International Conference on Bioinformatics and Biomedicine (BIBM)(2023)
摘要
Drug-target interaction (DTI) prediction is of great importance for drug discovery and development. With the rapid development of biological and chemical technologies, computational methods for DTI prediction are becoming a promising strategy. However, there are few methods which explore solving the cold-start problem in DTI prediction scenarios due to most of existing methods require modeling under the existing interaction that can’t effectively capture information from new drugs and new targets which have few interactions in existing literature. In this paper, we propose a graph transformer method based on meta-learning named MGDTI to fill the gap. In particular, we employ drug-drug similarity and target-target similarity as additional information for network to mitigate the scarcity of interactions. Besides, we trained our model via meta-learning to be adaptive to cold-start tasks. Moreover, we introduced graph transformer to prevent over-smoothing by capturing long-range dependencies. Comparison results on the benchmark dataset demonstrate that our proposed MGDTI is effective in the DTI prediction.
更多查看译文
关键词
drug-target interaction prediction,meta-learning,graph transformer
AI 理解论文
溯源树
样例
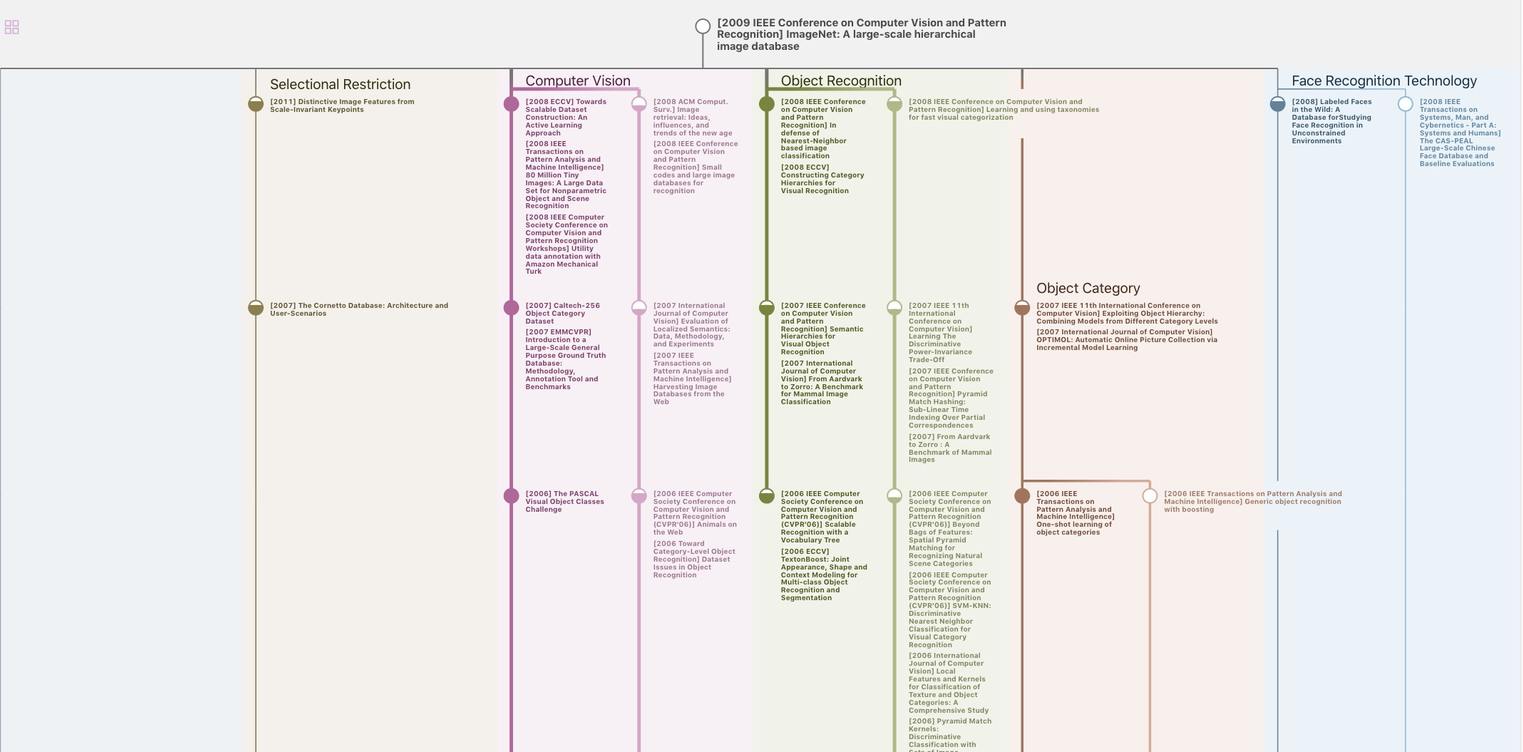
生成溯源树,研究论文发展脉络
Chat Paper
正在生成论文摘要