Mr. Clean: An Ensemble of Data Cleaning Algorithms for Increased Data Retention.
2023 IEEE International Conference on Bioinformatics and Biomedicine (BIBM)(2023)
Abstract
Handling missing data is a critical issue in nearly all analytical fields. Techniques that address this problem are generally categorized as either imputation or deletion algorithms. Imputation techniques replace missing data based on observed values and an assumed relationship, which can lead to biases in the imputed values and analysis results. Deletion programs remove missing data by deleting the corresponding sample or feature. This manuscript focuses on partial deletion, where some missing data is allowed, but samples and features with excessive missing data are removed. By intelligently selecting which rows and columns to delete, more valid data can be retained than with tradition deletion techniques. We developed three new algorithms for partial deletion: a greedy algorithm and two mathematical optimization programs. We compare these methods against the DataRetainer, Auto-miss, list-wise, and feature-wise programs, using several real-world data sets and a range of allowed missingness values. Our Greedy algorithm outperforms or ties existing algorithms in terms of run time and valid elements kept in nearly all scenarios. Our mathematical optimization programs further increase the number of valid elements kept, but require additional computational costs. These programs will allow researchers to retain more of their precious data thereby strengthening downstream analyses.
MoreTranslated text
Key words
Data Cleaning,data deletion,imputation
AI Read Science
Must-Reading Tree
Example
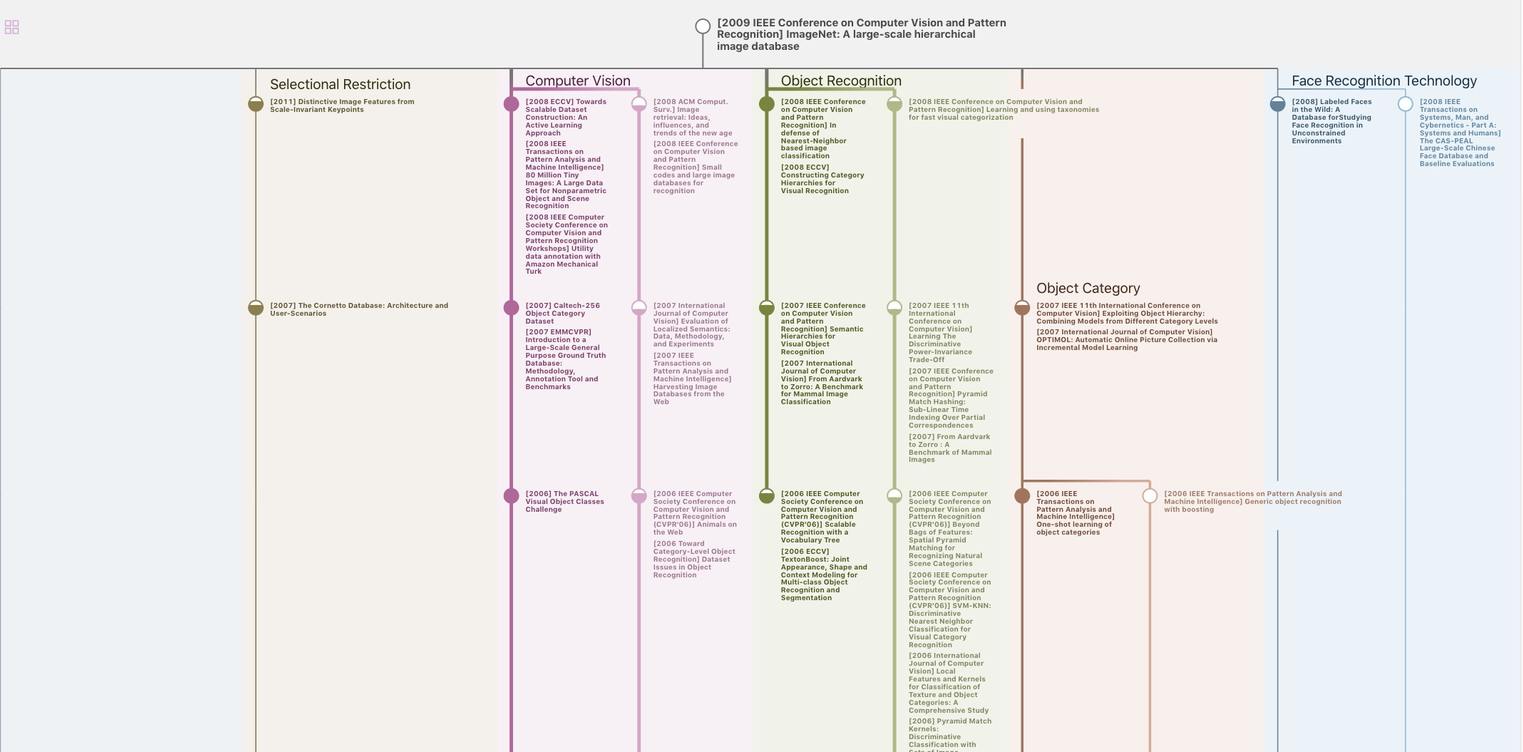
Generate MRT to find the research sequence of this paper
Chat Paper
Summary is being generated by the instructions you defined