MKGSAGE: A Computational Framework via Multiple Kernel Fusion on GraphSAGE for Inferring Potential Disease-Related Microbes.
2023 IEEE International Conference on Bioinformatics and Biomedicine (BIBM)(2023)
摘要
Microbes play a crucial role within the human body and are closely associated with the occurrence and development of numerous diseases. Studies have shown that disruptions in the composition and functionality of microbes can lead to immune system imbalances, inflammatory responses, and subsequently impact human health. Therefore, developing computational models to discover the potential connections between microbes and diseases is currently a hot topic. In this paper, a computational framework based on the multiple kernel fusion of graph embedding with sampling and aggregation (GraphSAGE) and dual Laplace regularized least squares called MKGSAGE is proposed for predicting potential links between microbe and disease. First, multiple layers embedding features of microbe and disease are learned from the initial input features by GraphSAGE. The kernel matrices are then calculated separately for each layer based on the Gaussian interaction profile (GIP). Furthermore, the multiple kernel fusion method is proposed for fusing kernel matrices of each layer and the initial similarity matrix. Dual Laplacian regularized least squares are finally applied for potential microbe-disease association prediction. Compared with six state-of-the-art methods on the HMDAD dataset, 5-fold cross-validations show that MKGSAGE performs best. In addition, case studies on asthma and inflammatory bowel disease further validate the effectiveness of MKGSAGE on discovering novel microbe-disease associations.
更多查看译文
关键词
Microbe-disease associations,Graph sample and aggregate,Multiple kernel fusion,Dual Laplacian regularized least squares
AI 理解论文
溯源树
样例
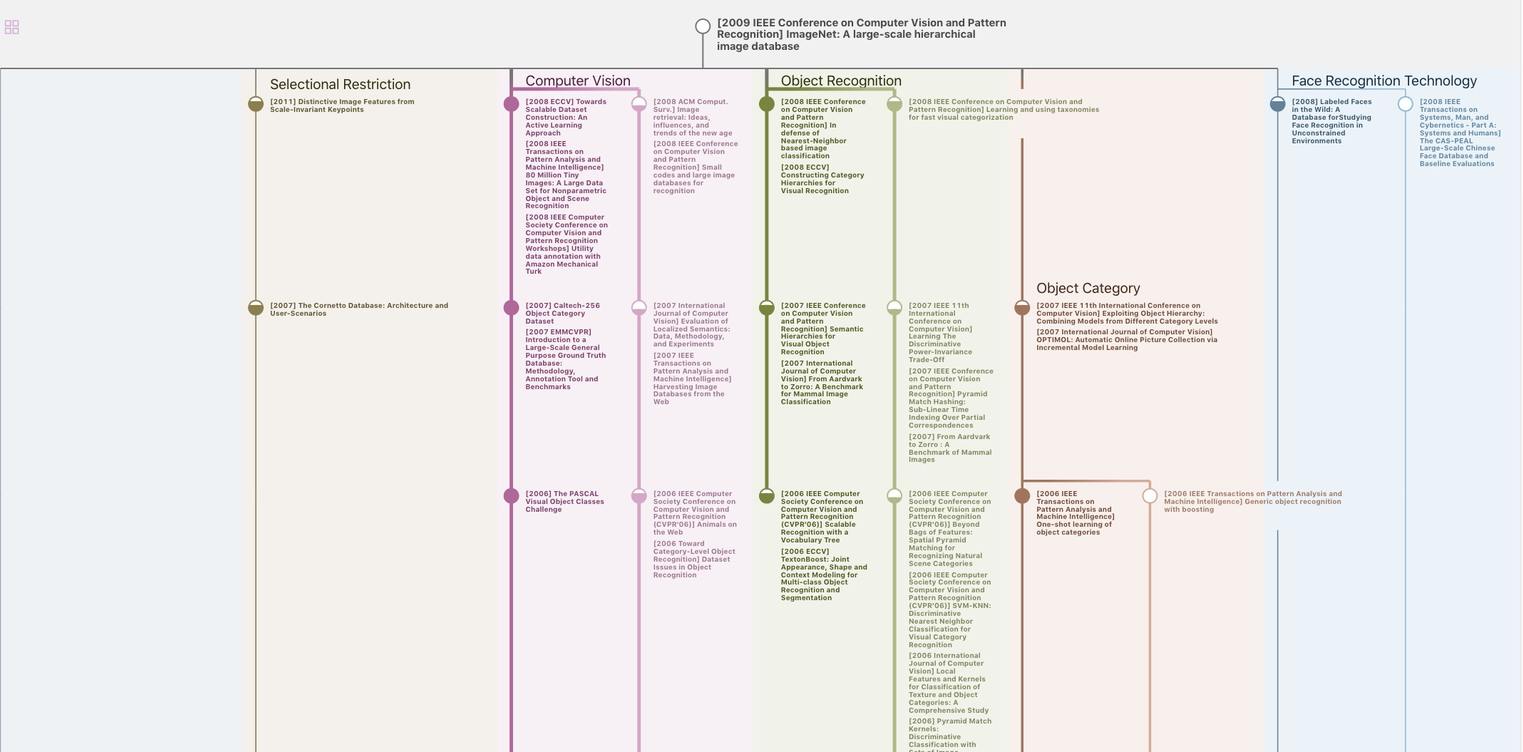
生成溯源树,研究论文发展脉络
Chat Paper
正在生成论文摘要