ChatICD: Prompt Learning for Few-shot ICD Coding through ChatGPT.
2023 IEEE International Conference on Bioinformatics and Biomedicine (BIBM)(2023)
摘要
Automated International Classification of Diseases (ICD) coding involves the automated assignment of diverse disease codes to clinical medical texts. It is considered as a multi-label classification task. Because most ICD codes are rare, the imbalanced distribution and small sample size issue make this task challenging. Inspired by the recent success of ChatGPT and prompt-based fine-tuning, this study proposes a model called ChatICD to address the issue of few-shot ICD coding. First, ChatGPT for data augumentation rephrases the descriptions of ICD codes into multiple samples. Then, ChatICD fine-tunes the pretrained model by generating prompt templates and label mapping words. We conduct an evaluation of ChatICD on benchmark datasets, namely MIMIC-III-50 and MIMIC-III-rare50. On the few-shot ICD coding task of MIMIC-III-rare50, ChatICD achieves macro-F1 and micro-F1 of 35.8% and 38.2% respectively, which is a 5.4% and 5.6% improvement over the current best model.
更多查看译文
关键词
ICD Coding,Few-shot Learning,ChatGPT,Prompt-based Fine-tuning
AI 理解论文
溯源树
样例
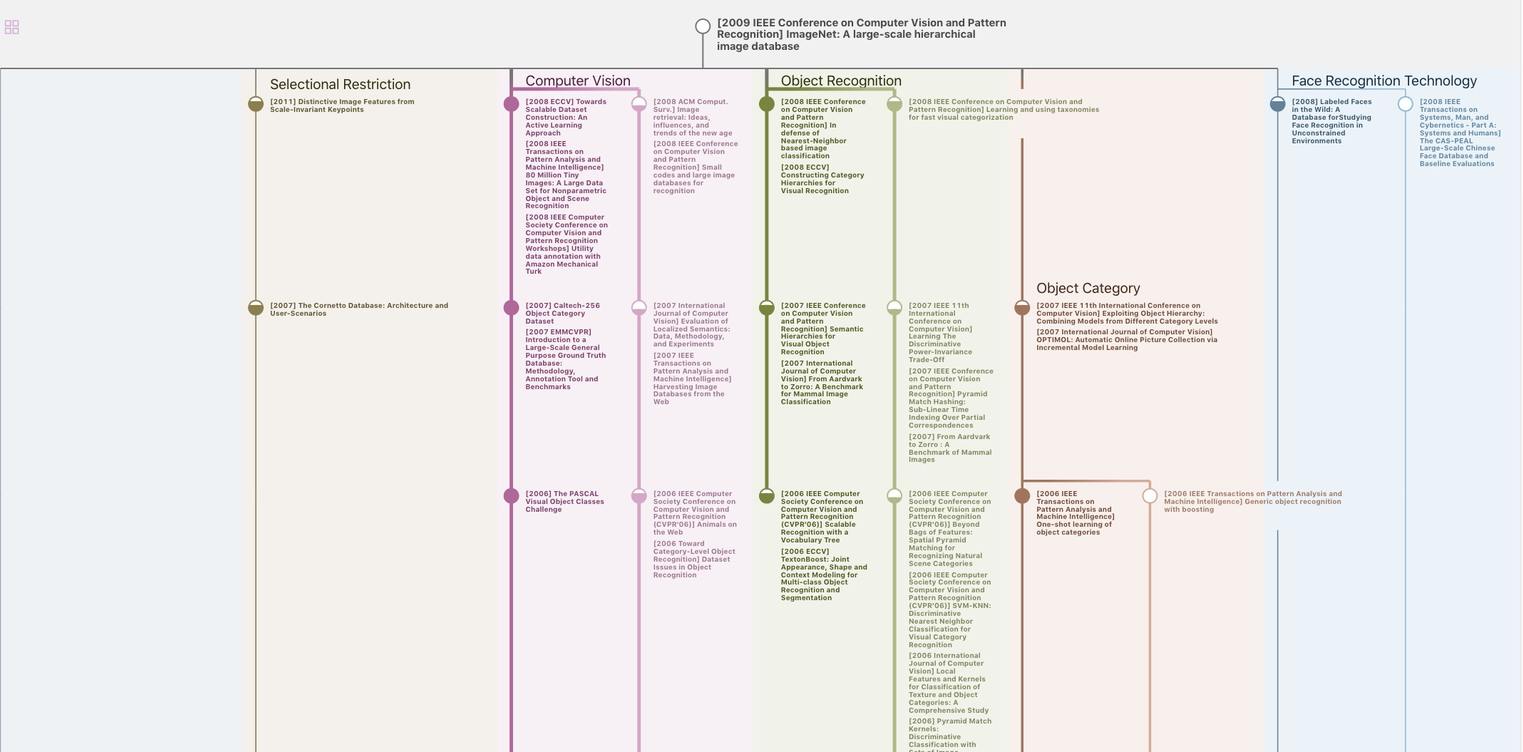
生成溯源树,研究论文发展脉络
Chat Paper
正在生成论文摘要