MTr-Net:A multipath fusion network based on 2.5D for medical image segmentation.
2023 IEEE International Conference on Bioinformatics and Biomedicine (BIBM)(2023)
摘要
Accurate segmentation of organs and tumours from medical images is to diagnose and treat diseases more accurately. Many organs, such as the representative pancreas and spleen, have blurred boundaries, small size, large variance, and inter-class imbalance. 2.5D methods based on 2D networks are currently widely used in various areas of medical image segmentation. However, 2D network-based methods are still unable to truly balance the contextual residual difference information. Therefore, we propose a new 2.5D multi-path Transformer fusion network(MTr-Net) that combines Z-axis information from 3D networks to address organs and tumours boundary deformation, while balancing contextual residual information. A fusion of two different methods is proposed to further refine the segmentation results of organs and tumours. Our method is evaluated on four widely accepted public datasets of pancreas and tumour, spleen, and skin lesions, which contain different imaging modalities. Specifically, these include the NIH public dataset, the MSD Pancreas public dataset, the MSD Spleen dataset and the ISIC2018 dataset. The algorithm performs well on all the above datasets. Our source codes will be released at https://github.com/graduation37289/MTr-net, once the manuscript is accepted for publication.
更多查看译文
关键词
Segmentation of organs and tumors,2.5D Methodology,Multipath Networks,Two fusion methods
AI 理解论文
溯源树
样例
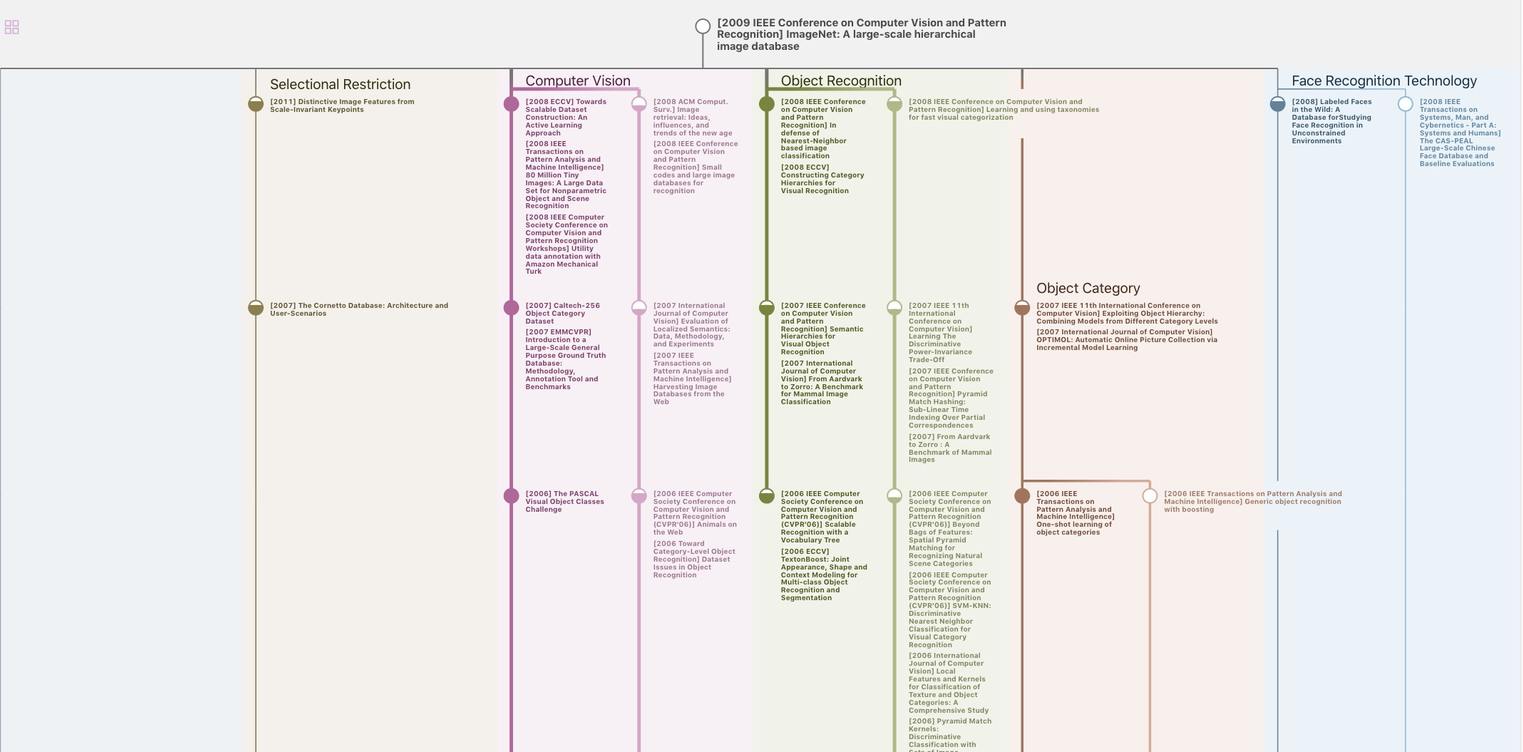
生成溯源树,研究论文发展脉络
Chat Paper
正在生成论文摘要