DARE: Sequence-Structure Dual-Aware Encoder for RNA-Protein Binding Prediction.
2023 IEEE International Conference on Bioinformatics and Biomedicine (BIBM)(2023)
摘要
Predicting RNA-protein binding sites helps to explore the mechanisms of the interaction between RNA and proteins. Numerous deep learning methods have been applied to predict RNA-protein binding sites. Some of these methods use only sequence information for prediction which could lose information about the topology. And there may be a loss of important information if the secondary structure features are simply represented as one-hot matrices. Furthermore, existing deep learning methods are usually based on convolutional neural networks for feature extraction, which tend to focus on local features. As for the information of the whole sequence, existing methods usually ignore global features. Therefore, we propose a novel deep learning model called DARE for RNA-protein binding sites prediction using both sequence and secondary structure information of RNA. DARE employs the secondary structure feature extraction module to capture the features of the RNA secondary structure and learn the topological information. Therefore, we design a local feature extraction module and a global feature integration module to capture the whole information of RNA. Thus we can achieve the purpose of complementary information. Extensive experiments demonstrate that DARE outperforms baselines. Our analysis of the case study further confirm the effectiveness of DARE.
更多查看译文
关键词
RNA-proteins binding prediction,RNA secondary structure,Transformer
AI 理解论文
溯源树
样例
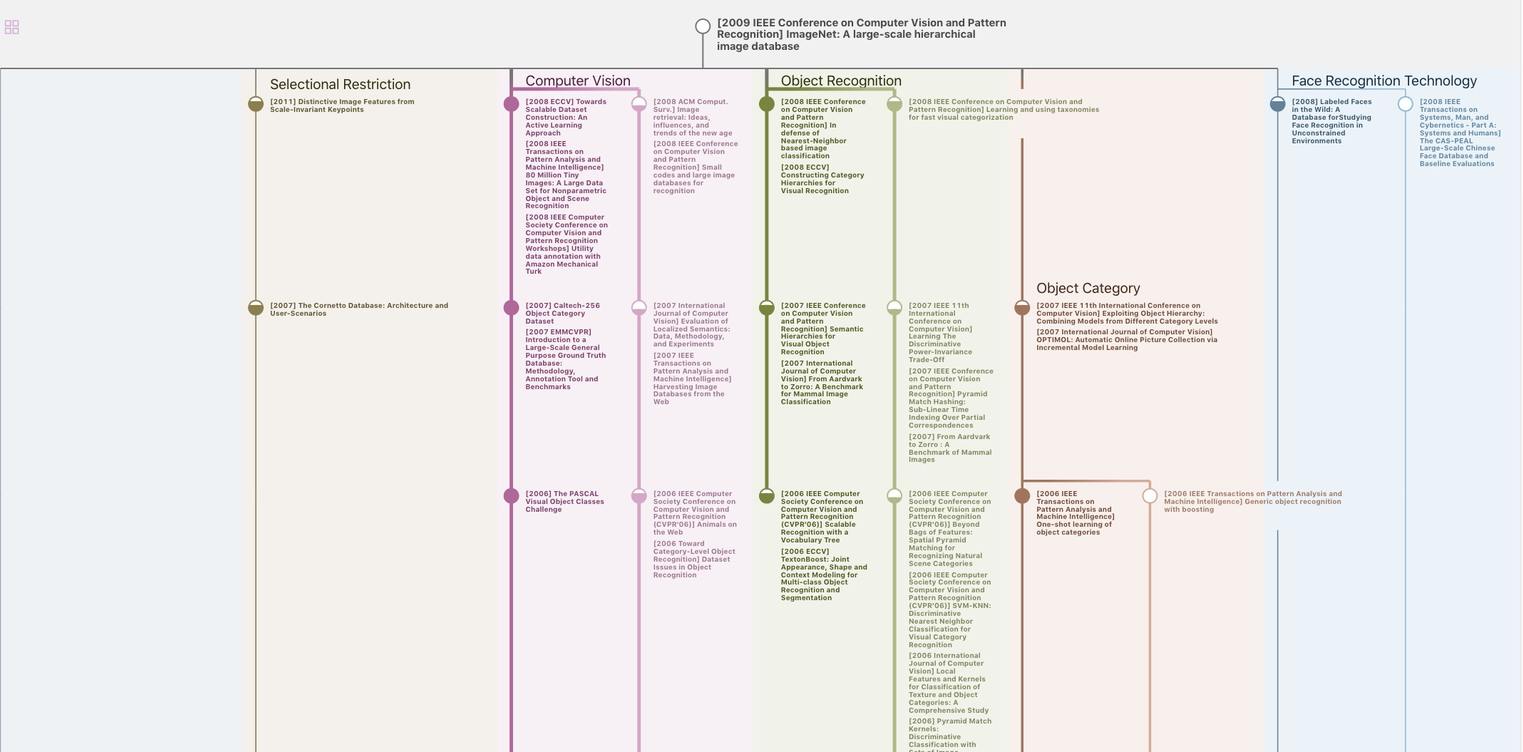
生成溯源树,研究论文发展脉络
Chat Paper
正在生成论文摘要