Assessing Tree-Based Phenotype Prediction on the UK Biobank.
2023 IEEE International Conference on Bioinformatics and Biomedicine (BIBM)(2023)
摘要
Precision medicine relies on the ability to identify associations between genomic data and its phenotypic expression in order to provide personalized predictions. Phenotype prediction using statistical models trained on large-scale genomic and phenotypic data is a critical research area at the intersection of machine learning and genomics. Current genotype-to-phenotype models, such as polygenic risk scores, only account for linear relationships, and the use of nonlinear methods is still partially unexplored. In this work, we evaluate the prediction accuracy and scalability of nine nonlinear decision tree-based algorithms, including ensembling and boosting mechanisms, and compare them to linear prediction models. We assess the prediction performance for 24 anthropometric and disease-related phenotypes present in the UK Biobank. By using random feature selection, we explore how accuracy and computational time vary for each method as a function of the number of genetic variants selected. Our results show that tree-based methods, especially gradientboosted trees, can offer superior predictions with computational times comparable to those of linear methods. Thus, models able to capture nonlinear relationships between genotypes and phenotypes merit consideration for integration in upcoming computational systems for personalized medicine.
更多查看译文
关键词
Bioinformatics,Phenotype Prediction,Precision Medicine,Machine Learning,Tree-based Methods
AI 理解论文
溯源树
样例
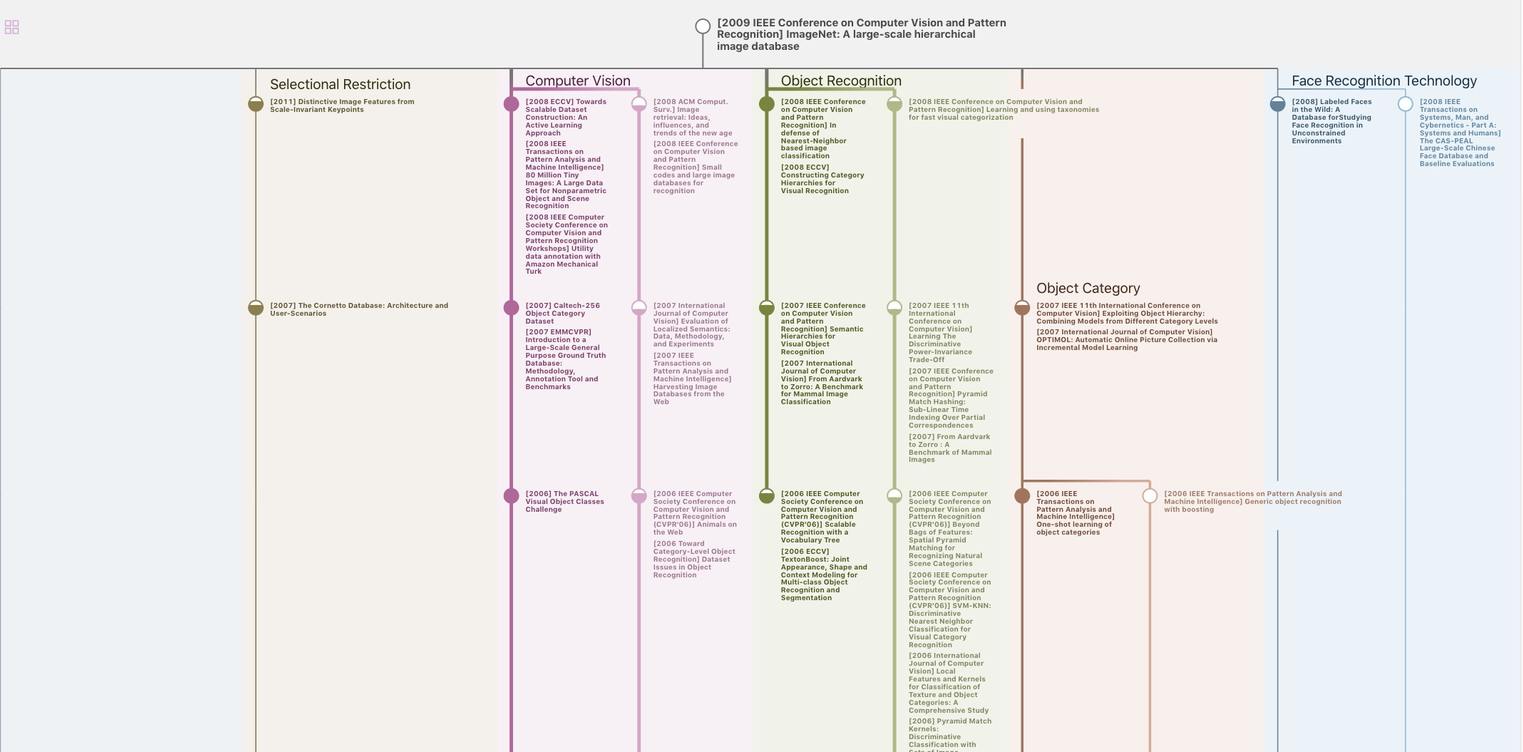
生成溯源树,研究论文发展脉络
Chat Paper
正在生成论文摘要