Enhancing Reproducibility in Precipitate Analysis: A FAIR Approach with Automated Dark-Field Transmission Electron Microscope Image Processing
Integrating Materials and Manufacturing Innovation(2024)
Abstract
High-strength aluminum alloys used in aerospace and automotive applications obtain their strength through precipitation hardening. Achieving the desired mechanical properties requires precise control over the nanometer-sized precipitates. However, the microstructure of these alloys changes over time due to aging, leading to a deterioration in strength. Typically, the size, number, and distribution of precipitates for a quantitative assessment of microstructural changes are determined by manual analysis, which is subjective and time-consuming. In our work, we introduce a progressive and automatable approach that enables a more efficient, objective, and reproducible analysis of precipitates. The method involves several sequential steps using an image repository containing dark-field transmission electron microscopy (DF-TEM) images depicting various aging states of an aluminum alloy. During the process, precipitation contours are generated and quantitatively evaluated, and the results are comprehensibly transferred into semantic data structures. The use and deployment of Jupyter Notebooks, along with the beneficial implementation of Semantic Web technologies, significantly enhances the reproducibility and comparability of the findings. This work serves as an exemplar of FAIR image and research data management.
MoreTranslated text
Key words
Automated image analysis,FAIR research data management,Reproducibility,Microstructural changes
AI Read Science
Must-Reading Tree
Example
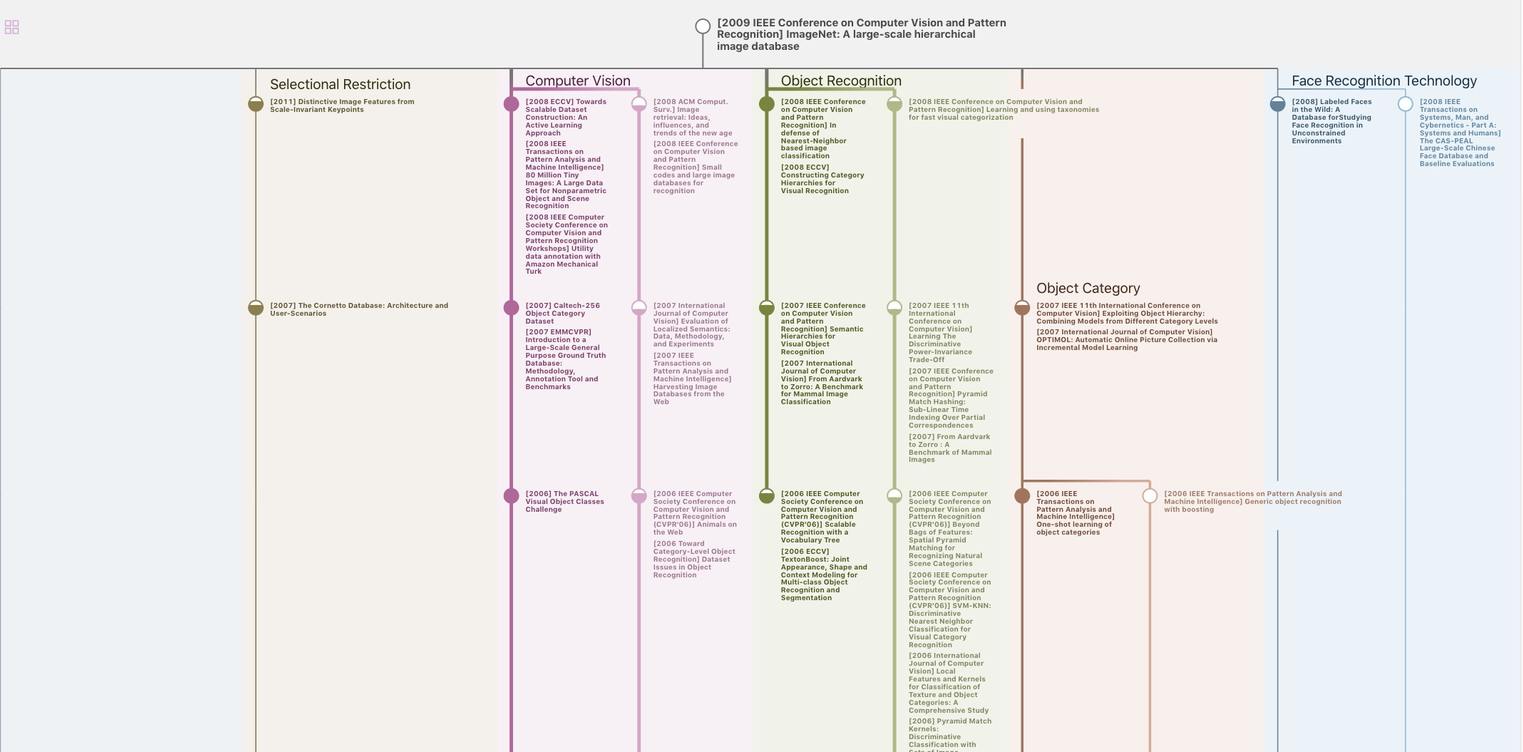
Generate MRT to find the research sequence of this paper
Chat Paper
Summary is being generated by the instructions you defined