Singular spectrum analysis based sleeping stage classification via electrooculogram
Multimedia Tools and Applications(2024)
摘要
The sleeping stage classification plays an important role in the medical science because it helps the diagnosis of the mental health diseases. The conventional approach for performing the sleeping stage classification is based on the electroencephalograms (EEGs). However, it is worth noting that the EEGs reflect the brain activities. Nevertheless, the brain activities are very complicated even though the subjects are sleeping. Hence, performing the sleeping stage classification via the EEGs may yield the low classification accuracy. On the other hand, the electrooculograms (EOGs) are the voltages between the front eyes and the back eyes which are related to the eye ball movement. As it can directly reflect the various sleeping stages, it can achieve a higher classification accuracy. Therefore, this paper employs the two channel EOGs for performing the sleeping stage classification. The major contribution of this paper is to 1) employ the singular spectrum analysis (SSA) to exploit the latent intrinsic high dimensional dynamics of the one dimensional EOGs for performing the sleeping stage classification, 2) employ the approximate entropy as the features for performing the sleeping stage classification, and 3) assign the same features of different SSA components of different channels of the epochs of the EOGs into the same group and perform the principal component analysis (PCA) on each group of the feature vectors so that the properties of each type of the features are preserved. The results show that our proposed method yields the five sleeping stage classification accuracy at 93.73% and the sensitivity of the stage one non-rapid eye movement (S1) at 78.44%, which achieves the significant improvements compared to the existing methods. Therefore, our proposed method could be used to reduce the workload of the medical officers.
更多查看译文
关键词
Electrooculograms,Singular spectrum analysis,one way variance analysis,Principal component analysis,random forest,Sleeping stage classification
AI 理解论文
溯源树
样例
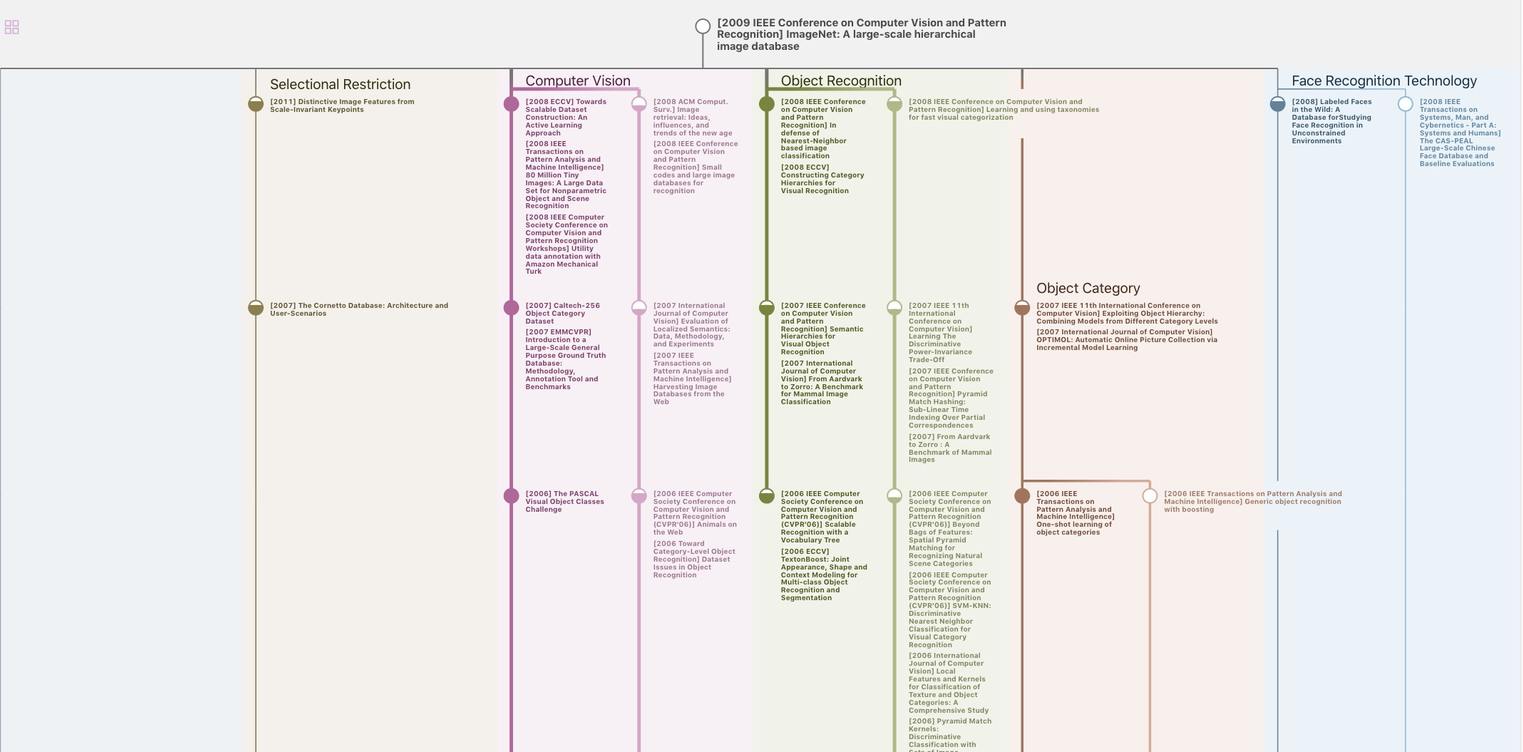
生成溯源树,研究论文发展脉络
Chat Paper
正在生成论文摘要