Learning sample-aware threshold for semi-supervised learning
Machine Learning(2024)
摘要
Pseudo-labeling methods are popular in semi-supervised learning (SSL). Their performance heavily relies on a proper threshold to generate hard labels for unlabeled data. To this end, most existing studies resort to a manually pre-specified function to adjust the threshold, which, however, requires prior knowledge and suffers from the scalability issue. In this paper, we propose a novel method named Meta-Threshold, which learns a dynamic confidence threshold for each unlabeled instance and does not require extra hyperparameters except a learning rate. Specifically, the instance-level confidence threshold is automatically learned by an extra network in a meta-learning manner. Considering limited labeled data as meta-data, the overall training objective of the classifier network and the meta-net can be formulated as a nested optimization problem that can be solved by a bi-level optimization scheme. Furthermore, by replacing the indicator function existed in the pseudo-labeling with a surrogate function, we theoretically provide the convergence of our training procedure, while discussing the training complexity and proposing a strategy to reduce its time cost. Extensive experiments and analyses demonstrate the effectiveness of our method on both typical and imbalanced SSL tasks.
更多查看译文
关键词
Semi-supervised learning,Confidence thresholds,Meta-learning,Bi-level optimization
AI 理解论文
溯源树
样例
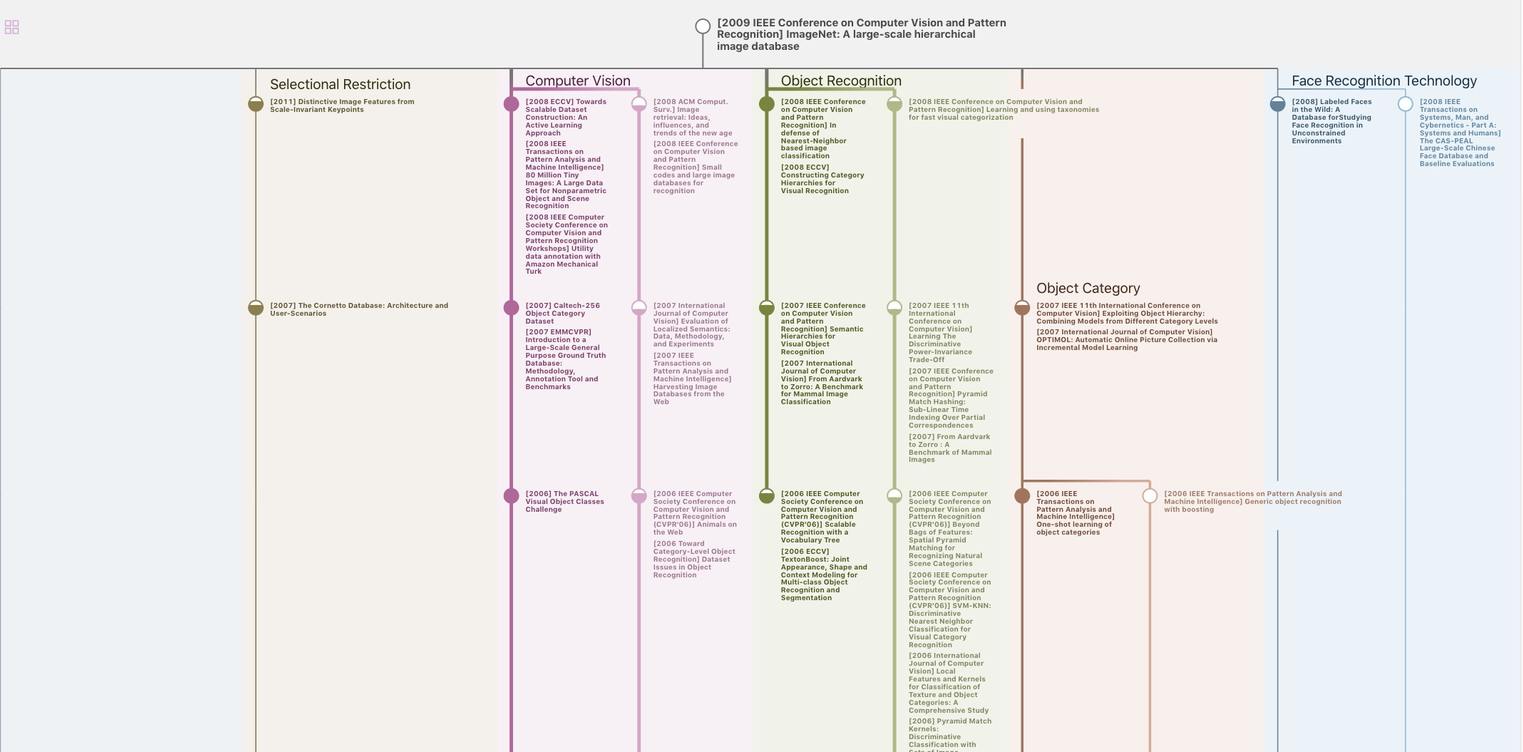
生成溯源树,研究论文发展脉络
Chat Paper
正在生成论文摘要