Population-based exploration in reinforcement learning through repulsive reward shaping using eligibility traces
Annals of Operations Research(2024)
Abstract
Efficient exploration plays a key role in accelerating the learning performance and sample efficiency of reinforcement learning tasks. In this paper we propose a framework that serves as a population-based repulsive reward shaping mechanism using eligibility traces to enhance the efficiency in exploring the state-space under the scope of tabular reinforcement learning representation. The framework contains a hierarchical structure of RL agents, where a higher level repulsive-reward-shaper agent ( RRS-Agent ) coordinates the exploration of its population of sub-agents through repulsion when necessary conditions on their eligibility traces are met. Empirical results on well-known benchmark problem domains show that the framework indeed achieves efficient exploration with a significant improvement in learning performance and state-space coverage. Furthermore, the transparency of the proposed framework enables explainable decisions made by the agents in the hierarchical structure to explore the state-space in a coordinated manner and supports the interpretability of the framework.
MoreTranslated text
Key words
Reinforcement learning,Population-based exploration,Eligibility traces,Reward shaping,Coordinated agents
AI Read Science
Must-Reading Tree
Example
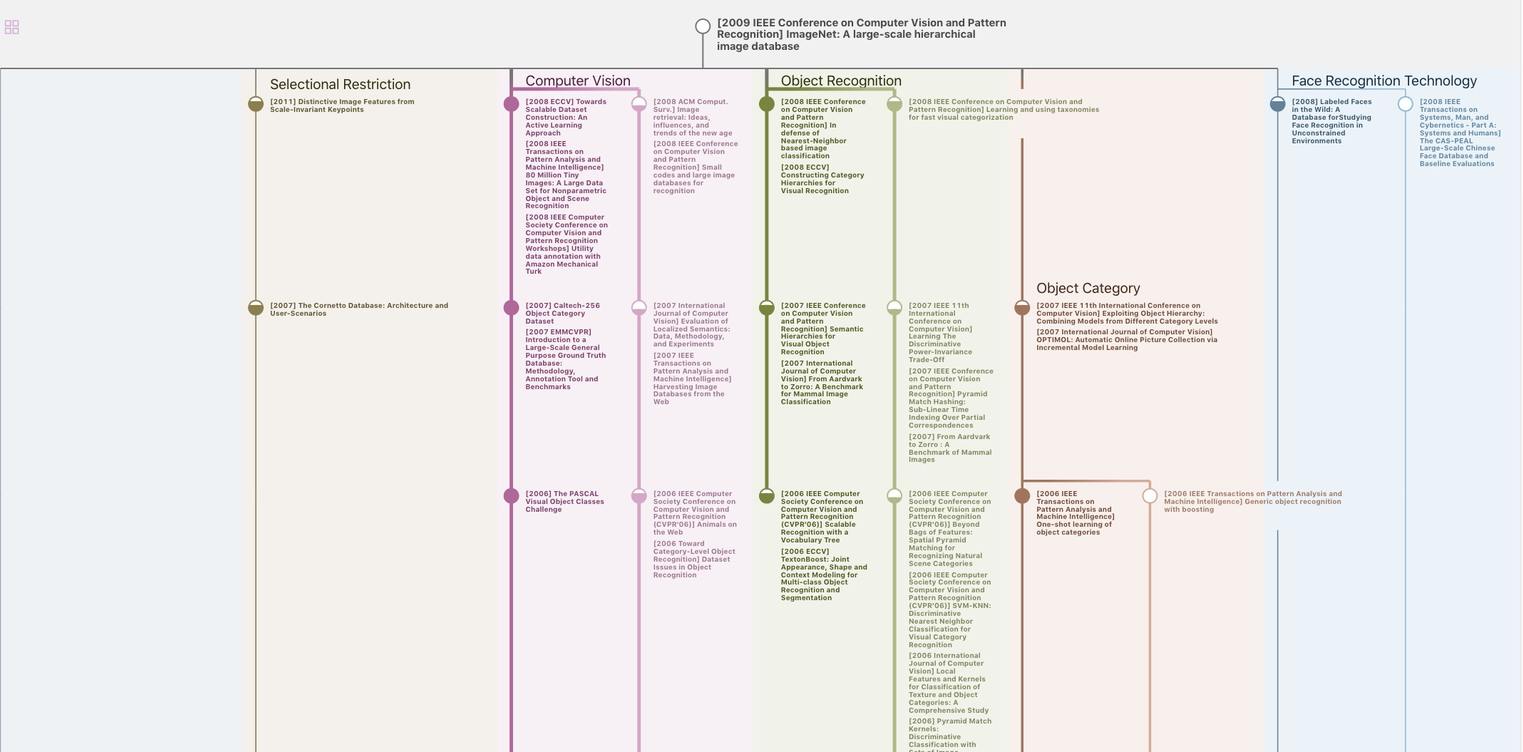
Generate MRT to find the research sequence of this paper
Chat Paper
Summary is being generated by the instructions you defined