BSRU: boosting semi-supervised regressor through ramp-up unsupervised loss
Knowledge and Information Systems(2024)
摘要
Semi-supervised regression aims to improve the performance of the learner with the help of unlabeled data. Popular approaches select some unlabeled data with high-quality pseudo labels to enrich the training set. In this paper, we propose a new approach with a semi-supervised regressor, a learner, and a respective loss function. First, an off-the-shelf semi-supervised regressor is trained to provide pseudo labels for all unlabeled data. These labels are often reliable enough to guide the learning process. Second, we design a neural network with dropout to train data with Gaussian noise added. In this way, the robustness of our learners is enhanced. Third, we design a weighted sum combining the supervised and unsupervised loss. The weight for pseudo-labels ramp-up over time, indicating more attention to the pseudo-labels. Six state-of-the-art algorithms are employed as the base model of our framework. Results on 15 real-world data sets show that our model has a significant improvement over the respective base regressor on most data sets.
更多查看译文
关键词
Semi-supervised regression,Unsupervised loss,Ramp-up
AI 理解论文
溯源树
样例
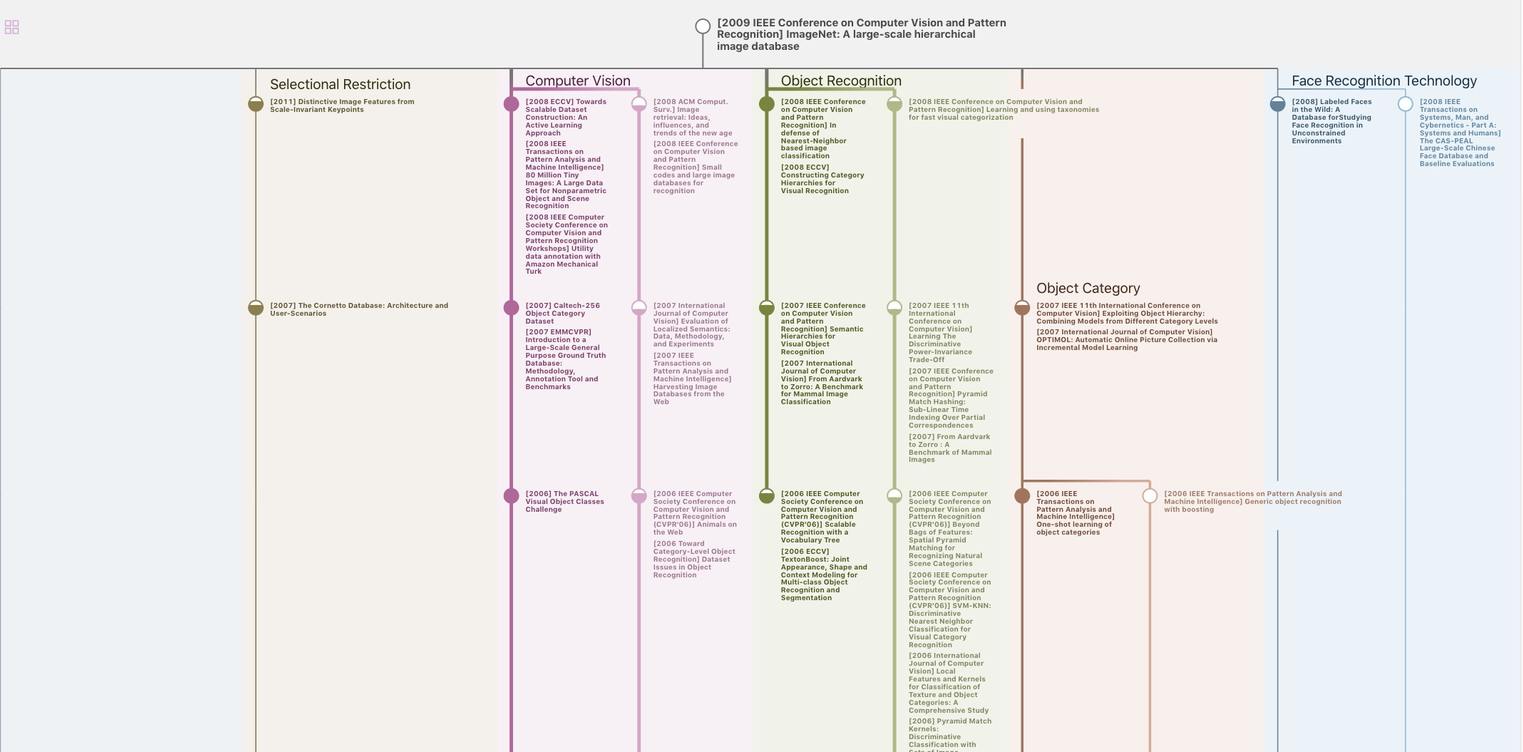
生成溯源树,研究论文发展脉络
Chat Paper
正在生成论文摘要