A new approach to nonparametric estimation of multivariate spectral density function using basis expansion
Computational Statistics(2024)
摘要
This paper develops a nonparametric method for estimating the spectral density of multivariate stationary time series using basis expansion. A likelihood-based approach is used to fit the model through the minimization of a penalized Whittle negative log-likelihood. Then, a Newton-type algorithm is developed for the computation. In this method, we smooth the Cholesky factors of the multivariate spectral density matrix in a way that the reconstructed estimate based on the smoothed Cholesky components is consistent and positive-definite. In a simulation study, we have illustrated and compared our proposed method with other competitive approaches. Finally, we apply our approach to two real-world problems, Electroencephalogram signals analysis, El Niño Cycle.
更多查看译文
关键词
Multivariate time series,Spectral analysis,Smoothing spline,Whittle likelihood,Regularization
AI 理解论文
溯源树
样例
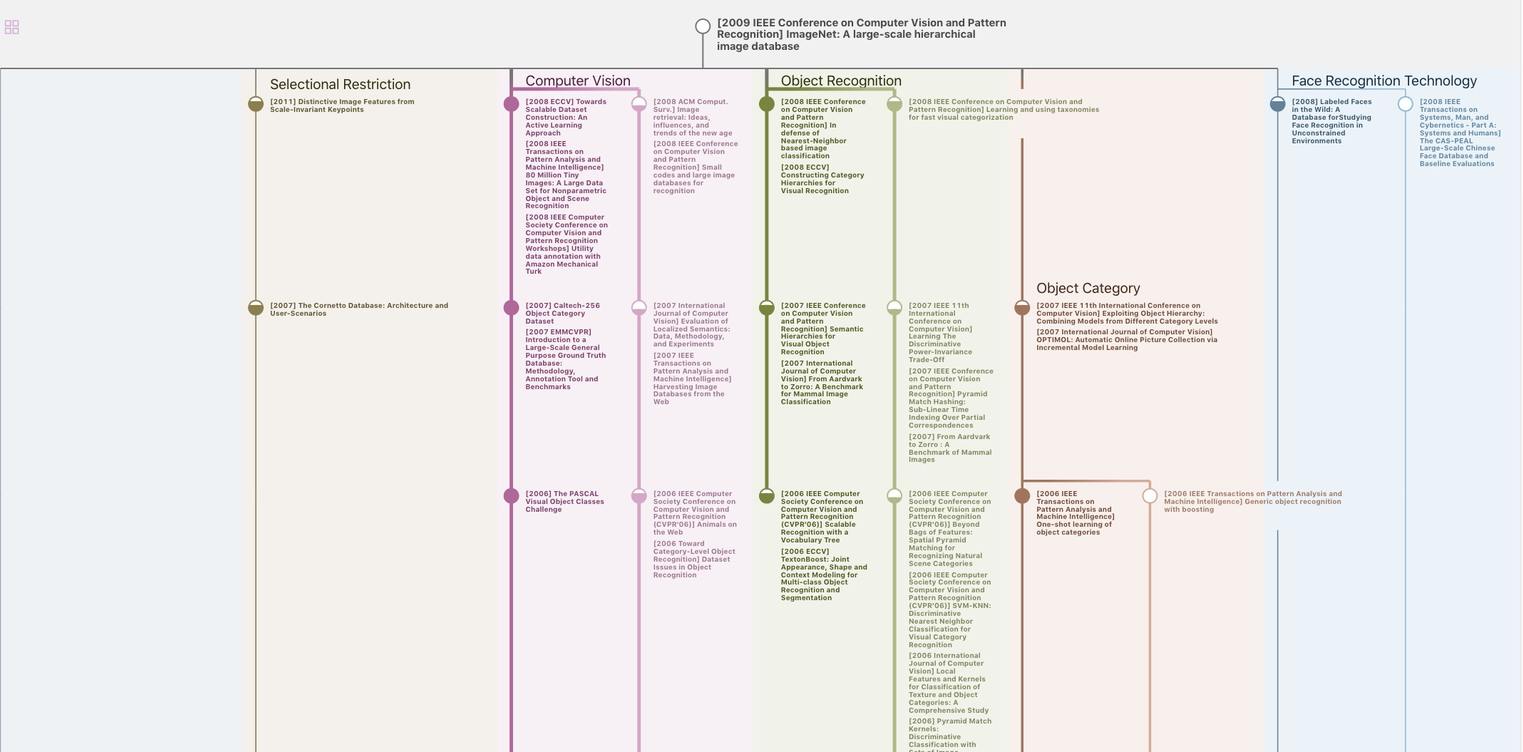
生成溯源树,研究论文发展脉络
Chat Paper
正在生成论文摘要