Learning to Robustly Reconstruct Low-light Dynamic Scenes from Spike Streams
CoRR(2024)
Abstract
As a neuromorphic sensor with high temporal resolution, spike camera can generate continuous binary spike streams to capture per-pixel light intensity. We can use reconstruction methods to restore scene details in high-speed scenarios. However, due to limited information in spike streams, low-light scenes are difficult to effectively reconstruct. In this paper, we propose a bidirectional recurrent-based reconstruction framework, including a Light-Robust Representation (LR-Rep) and a fusion module, to better handle such extreme conditions. LR-Rep is designed to aggregate temporal information in spike streams, and a fusion module is utilized to extract temporal features. Additionally, we have developed a reconstruction benchmark for high-speed low-light scenes. Light sources in the scenes are carefully aligned to real-world conditions. Experimental results demonstrate the superiority of our method, which also generalizes well to real spike streams. Related codes and proposed datasets will be released after publication.
MoreTranslated text
AI Read Science
Must-Reading Tree
Example
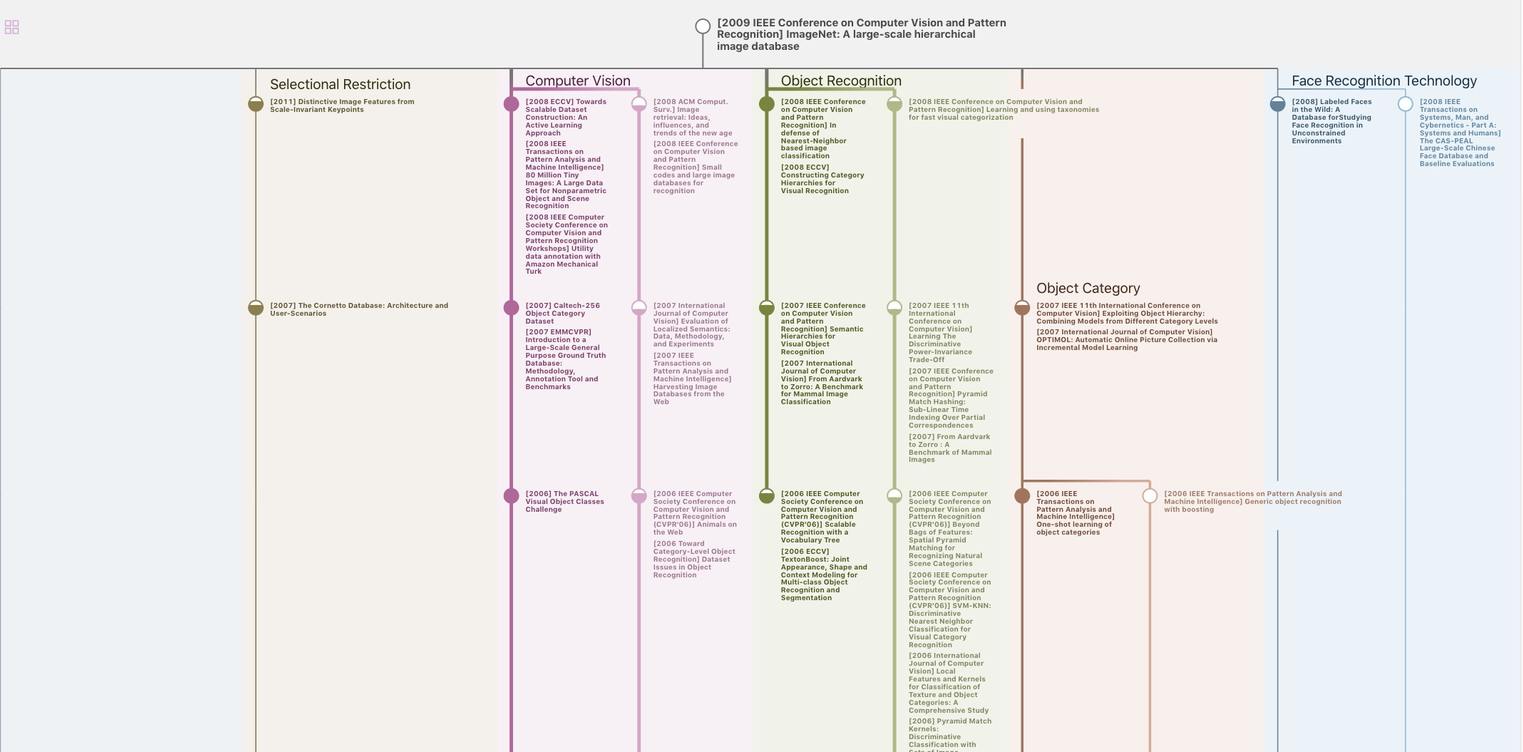
Generate MRT to find the research sequence of this paper
Chat Paper
Summary is being generated by the instructions you defined