HRL-TSCH: A Hierarchical Reinforcement Learning-based TSCH Scheduler for IIoT
CoRR(2024)
Abstract
The Industrial Internet of Things (IIoT) demands adaptable Networked Embedded Systems (NES) for optimal performance. Combined with recent advances in Artificial Intelligence (AI), tailored solutions can be developed to meet specific application requirements. This study introduces HRL-TSCH, an approach rooted in Hierarchical Reinforcement Learning (HRL), to devise Time Slotted Channel Hopping (TSCH) schedules provisioning IIoT demand. HRL-TSCH employs dual policies: one at a higher level for TSCH schedule link management, and another at a lower level for timeslot and channel assignments. The proposed RL agents address a multi-objective problem, optimizing throughput, power efficiency, and network delay based on predefined application requirements. Simulation experiments demonstrate HRL-TSCH superiority over existing state-of-art approaches, effectively achieving an optimal balance between throughput, power consumption, and delay, thereby enhancing IIoT network performance.
MoreTranslated text
Key words
Industrial Internet of Things (IIoT),Networked Embedded Systems (NES),Sleep Scheduling,Time Slotted Channel Hopping (TSCH),Reinforcement Learning (RL),Software-Defined Wireless Sensor Networks (SDWSNs)
AI Read Science
Must-Reading Tree
Example
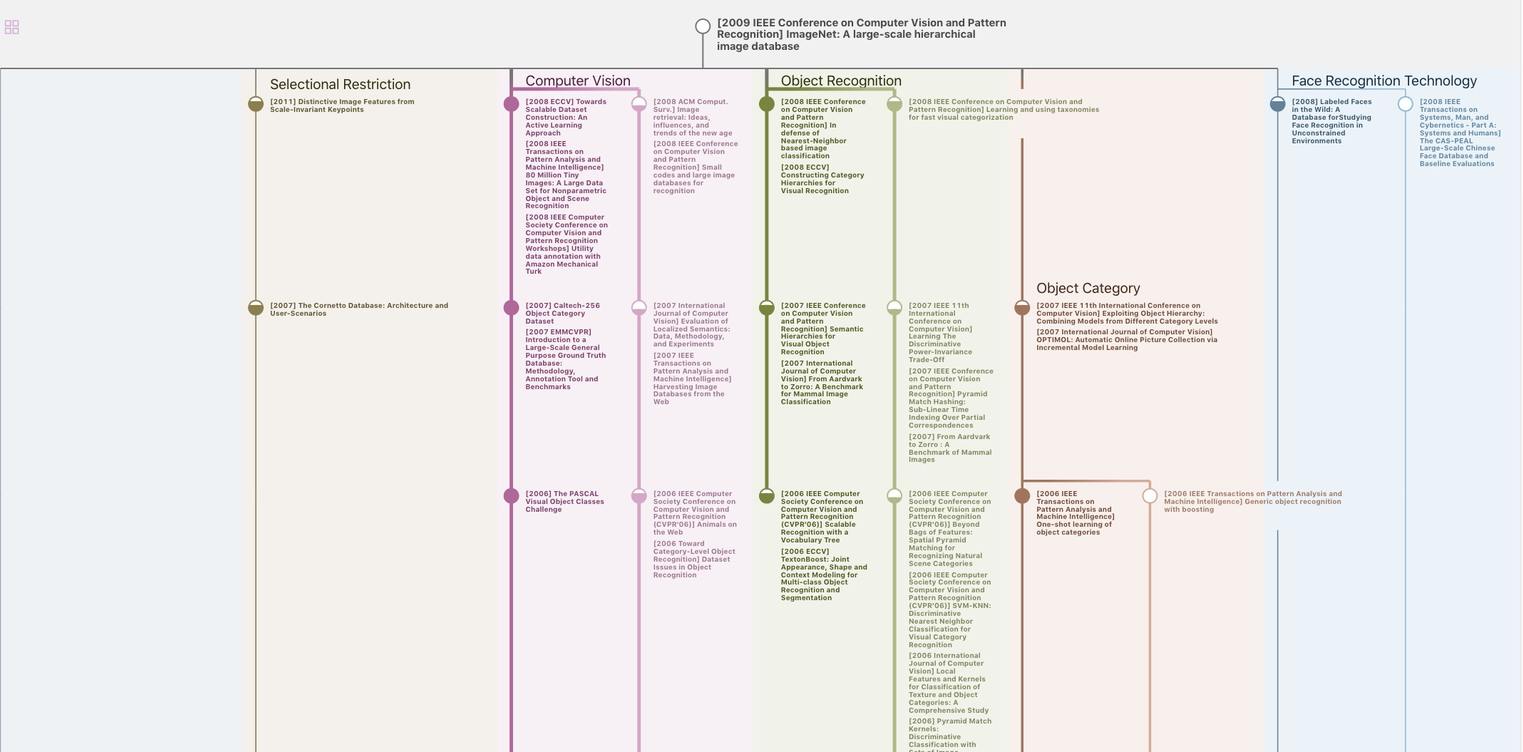
Generate MRT to find the research sequence of this paper
Chat Paper
Summary is being generated by the instructions you defined