Dynamic real-time forecasting technique for reclaimed water volumes in urban river environmental management
ENVIRONMENTAL RESEARCH(2024)
摘要
In recent years, the utilization of wastewater recycling as an alternative water source has gained significant traction in addressing urban water shortages. Accurate prediction of wastewater quantity is paramount for effective urban river water resource management. There is an urgent need to develop advanced forecasting technologies to further enhance the accuracy and efficiency of water quantity predictions. Decomposition ensemble models have shown excellent predictive capabilities but are challenged by boundary effects when decomposing the original data sequence. To address this, a rolling forecast decomposition ensemble scheme was developed. It involves using a machine learning (ML) model for prediction and progressively integrating prediction outcomes into the original sequence using complementary ensemble empirical mode decomposition with adaptive noise (CEEMDAN). Long short-term memory (LSTM) is then applied for sub -signal prediction and ensemble. The ML-CEEMDAN-LSTM model was introduced for wastewater quantity prediction, compared with non -decomposed ML models, CEEMDAN-based LSTM models, and ML-CEEMDAN-based LSTM models. Three ML algorithms-linear regression (LR), gradient boosting regression (GBR), and LSTM-were examined, using realtime prediction data and historical monitoring data, with historical data selected using the decision tree method. The study used daily water volumes data from two reclaimed water plants, CH and WQ, in Beijing. The results indicate that (1) ML models varied in their selection of real-time factors, with LR performing best among ML models during testing; (2) the ML-CEEMDAN-LSTM model consistently outperformed ML models; (3) the MLCEEMDAN-LSTM hybrid model performed better than the CEEMDAN-LSTM model across different seasons. This study offers a reliable and accurate approach for reclaimed water volumes forecasting, critical for effective water environment management.
更多查看译文
关键词
Reclaimed water volumes,Dynamic real-time forecasting,Machine learning,Forecast decomposition and ensemble,technique
AI 理解论文
溯源树
样例
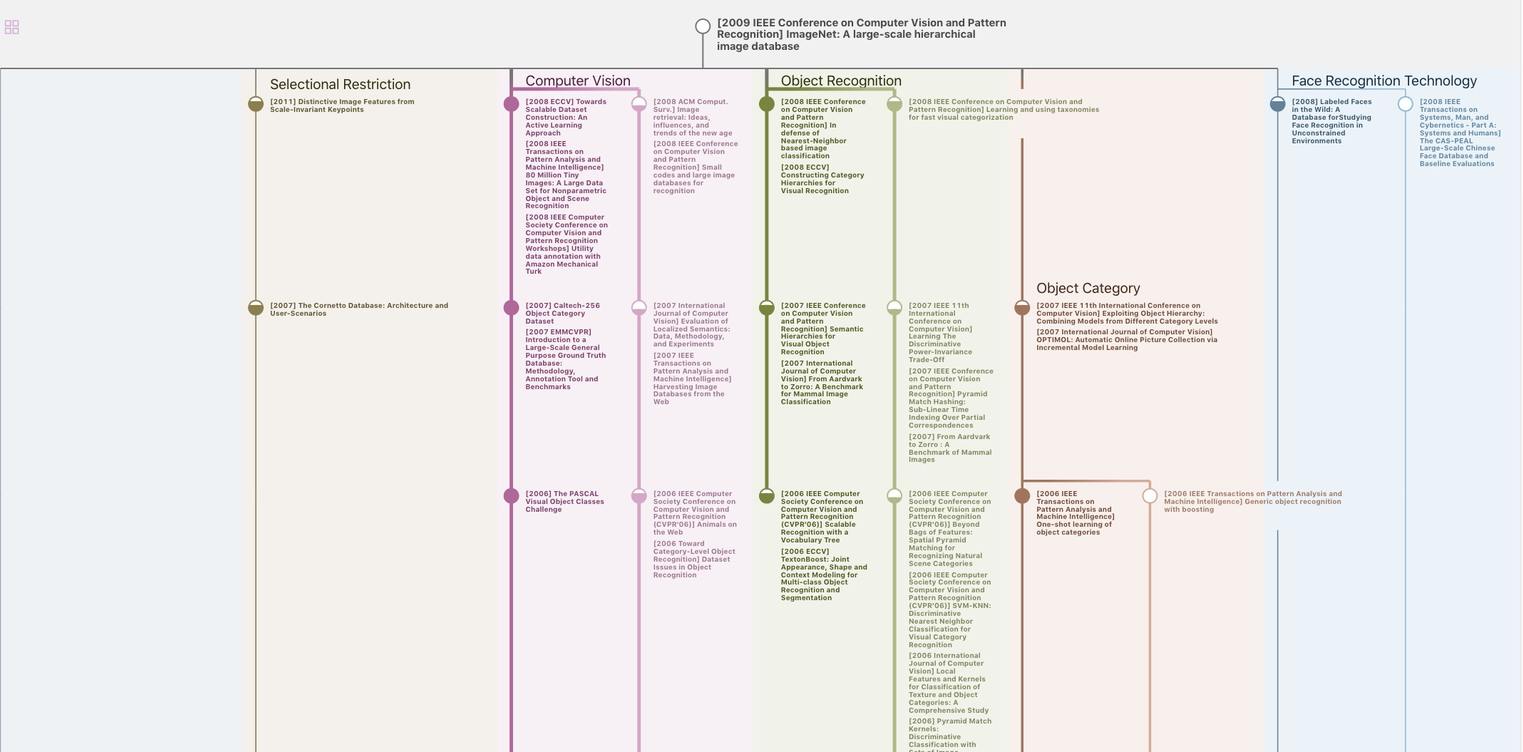
生成溯源树,研究论文发展脉络
Chat Paper
正在生成论文摘要