MOSER: Scalable Network Motif Discovery using Serial Test
PROCEEDINGS OF THE VLDB ENDOWMENT(2023)
摘要
Given a graph G, a motif (e.g., 3-node clique) is a fundamental building block for... Recently, motif-based graph analysis has attracted much attention due to its efficacy in tasks such as clustering, ranking, and link prediction. These tasks require Network Motif Discovery (NMD) at the early stage to identify the motifs of... However, existing NMD solutions have two drawbacks: (1) Lack of theoretical guarantees on the quality of the samples generated using the existing methods, and (2) inefficient algorithms, which are not scalable for large graphs. These limitations hinder the exploration of motifs for analyzing large graphs. To address the above issues, we propose a novel solution named MOSER (MOtif Discovery using SERial Test). This novel NMD framework leverages a significance testing method known as the serial test, which differs from the existing solutions. We further propose two fast incremental subgraph counting algorithms, allowing MOSER to scale to larger graphs than ever possible before. Extensive experimental results show that using MOSER can improve the state-of-the-art up to 5 orders of magnitude in efficiency and that the motifs found by MOSER facilitate downstream tasks such as link prediction.
更多查看译文
AI 理解论文
溯源树
样例
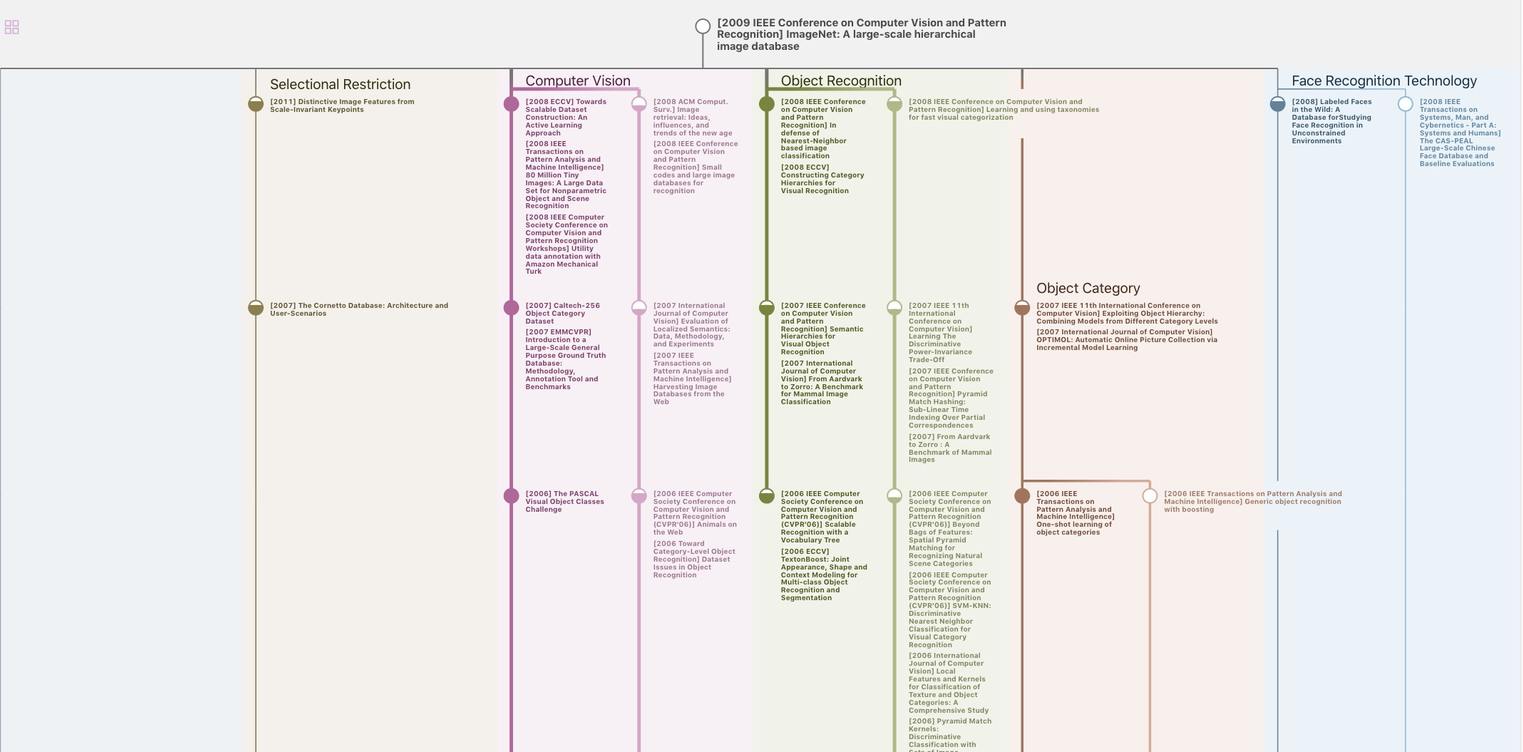
生成溯源树,研究论文发展脉络
Chat Paper
正在生成论文摘要