A deep learning approach for health monitoring in rotating machineries using vibrations and thermal features
Decision Analytics Journal(2024)
摘要
Gearbox failures can lead to substantial damage, significant financial losses due to maintenance downtimes, and, in some instances, fatalities. This study introduces an intelligent gear fault diagnosis system employing a convolutional neural network (CNN), utilizing vibration and thermal features extracted from healthy, chipped, and broken tooth gear health categories. The performance of the CNN is compared with conventional machine learning models, including Naïve Bayes (NB), random forest (RF), and support vector machine (SVM) classifiers. Experimental investigations highlight CNN’s remarkable performance. With vibration features, the CNN achieved 96.78% accuracy, surpassing SVM (84.89%), NB (81.56%), and RF (85.11%). The CNN attained 100% accuracy when utilizing thermal features, while SVM, NB, and RF achieved 91.11%, 88.89%, and 96.51% accuracies, respectively.
更多查看译文
关键词
Convolutional neural network,Gearbox,Machine learning,Fault diagnosis,Thermal image,Vibrational signal
AI 理解论文
溯源树
样例
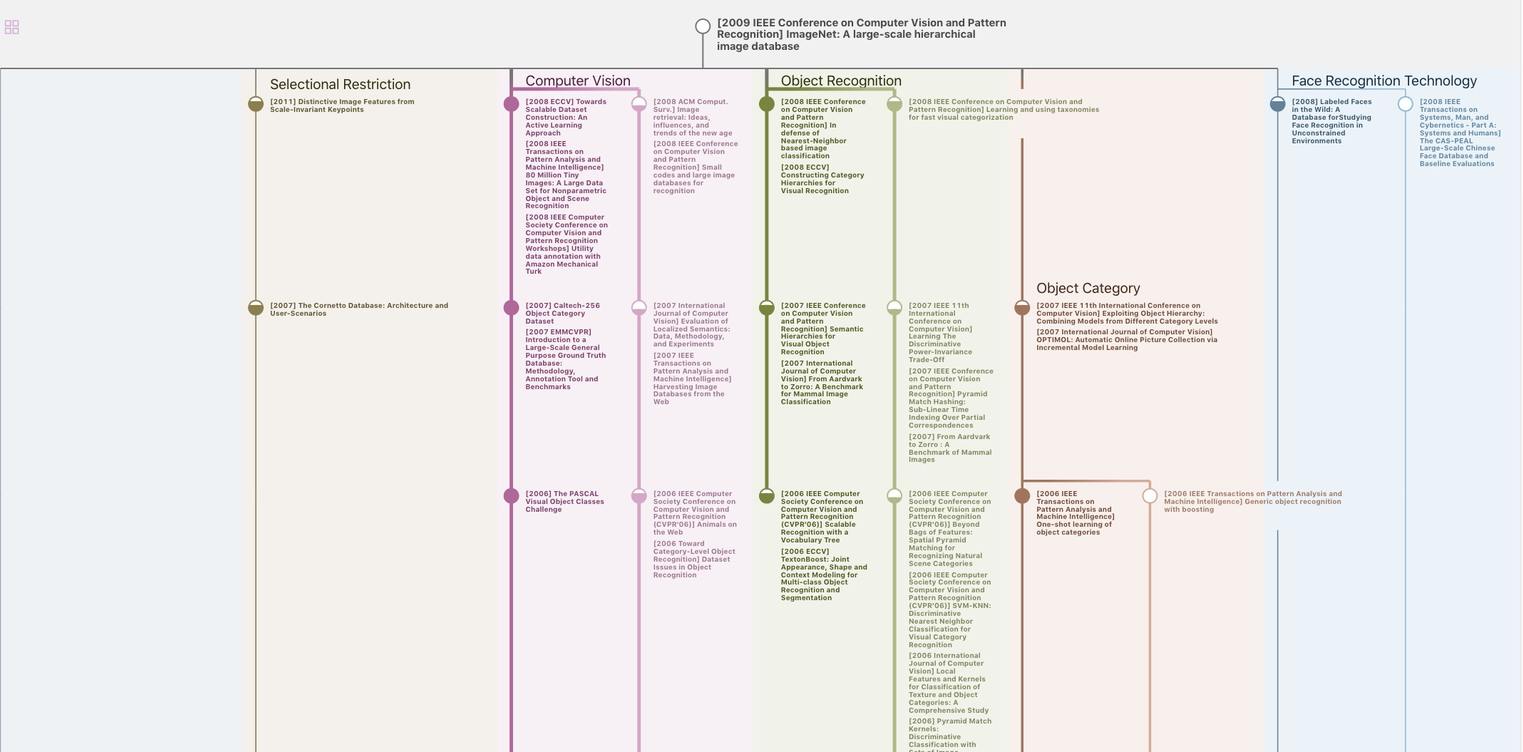
生成溯源树,研究论文发展脉络
Chat Paper
正在生成论文摘要