Unsupervised Discriminative Feature Selection via Contrastive Graph Learning
IEEE TRANSACTIONS ON IMAGE PROCESSING(2024)
摘要
Due to many unmarked data, there has been tremendous interest in developing unsupervised feature selection methods, among which graph-guided feature selection is one of the most representative techniques. However, the existing feature selection methods have the following limitations: (1) All of them only remove redundant features shared by all classes and neglect the class-specific properties; thus, the selected features cannot well characterize the discriminative structure of the data. (2) The existing methods only consider the relationship between the data and the corresponding neighbor points by Euclidean distance while neglecting the differences with other samples. Thus, existing methods cannot encode discriminative information well. (3) They adaptively learn the graph in the original or embedding space. Thus, the learned graph cannot characterize the data's cluster structure. To solve these limitations, we present a novel unsupervised discriminative feature selection via contrastive graph learning, which integrates feature selection and graph learning into a uniform framework. Specifically, our model adaptively learns the affinity matrix, which helps characterize the data's intrinsic and cluster structures in the original space and the contrastive learning. We minimize & ell;(1,2) -norm regularization on the projection matrix to preserve class-specific features and remove redundant features shared by all classes. Thus, the selected features encode discriminative information well and characterize the discriminative structure of the data. Generous experiments indicate that our proposed model has state-of-the-art performance.
更多查看译文
关键词
Feature extraction,Sparse matrices,Self-supervised learning,Data models,Telecommunications,Spatial databases,Redundancy,Contrastive learning,classification,self-representation,sparse learning,unsupervised feature selection
AI 理解论文
溯源树
样例
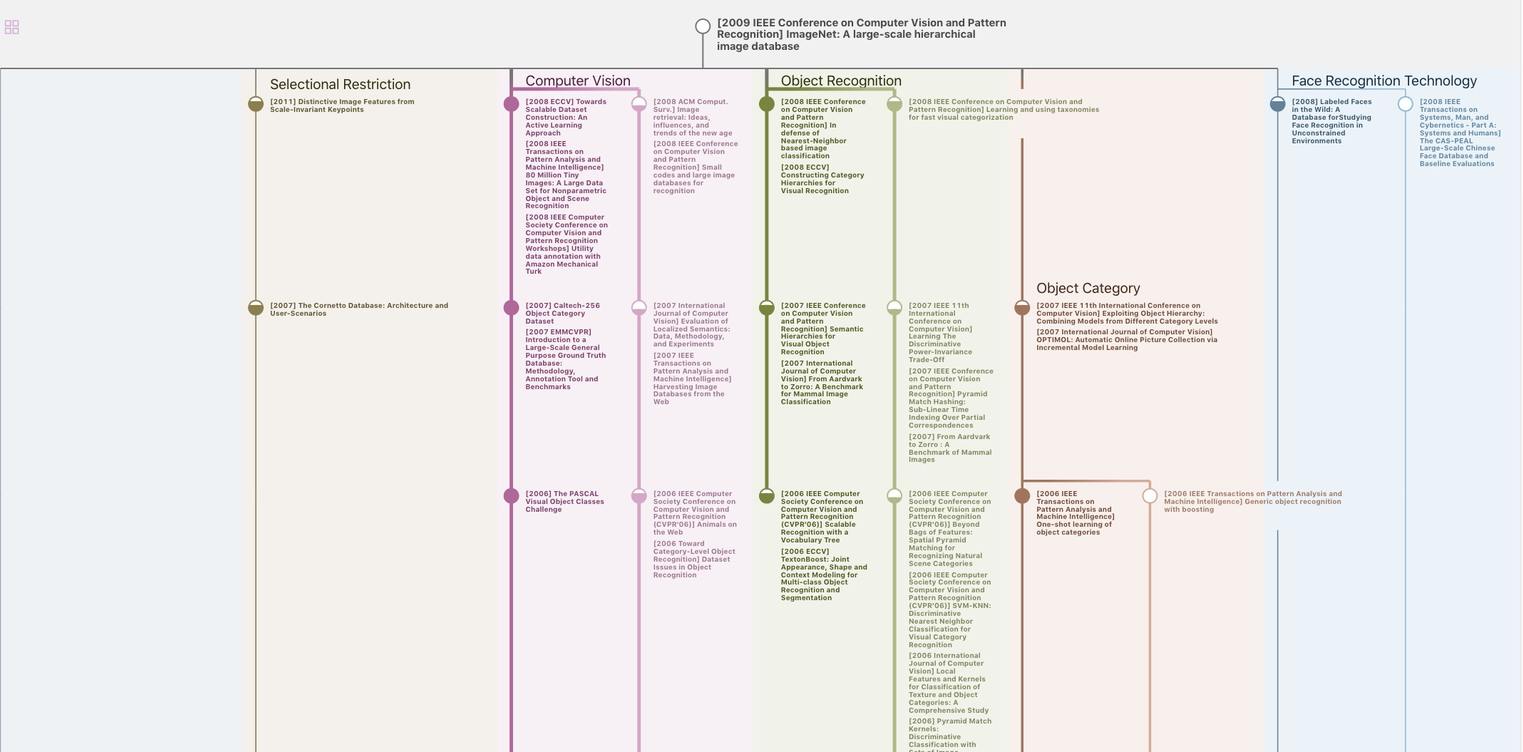
生成溯源树,研究论文发展脉络
Chat Paper
正在生成论文摘要