Rolling bearing fault diagnosis based on Gramian angular difference field and improved channel attention model
PEERJ COMPUTER SCIENCE(2024)
Abstract
Fault diagnosis of rolling bearings is a critical task, and in previous research, convolutional neural networks (CNN) have been used to process vibration signals and perform fault diagnosis. However, traditional CNN models have certain limitations in terms of accuracy. To improve accuracy, we propose a method that combines the Gramian angular difference field (GADF) with residual networks (ResNet) and embeds frequency channel attention module (Fca) in the ResNet to diagnose rolling bearing fault. Firstly, we used GADF to convert the signals into RGB three-channel fault images during data preprocessing. Secondly, to further enhance the performance of the model, on the foundation of the ResNet we embedded the frequency channel attention module with discrete cosine transform (DCT) to form Fca, to effectively explores the channel information of fault images and identifies the corresponding fault characteristics. Finally, the experiment validated that the accuracy of the new model reaches 99.3% and the accuracy reaches 98.6% even under an unbalanced data set, which significantly improves the accuracy of fault diagnosis and the generalization of the model.
MoreTranslated text
Key words
Fault diagnosis,Rolling bearings,Gramian Angular Difference Field,Channel attention module,Residual networks
AI Read Science
Must-Reading Tree
Example
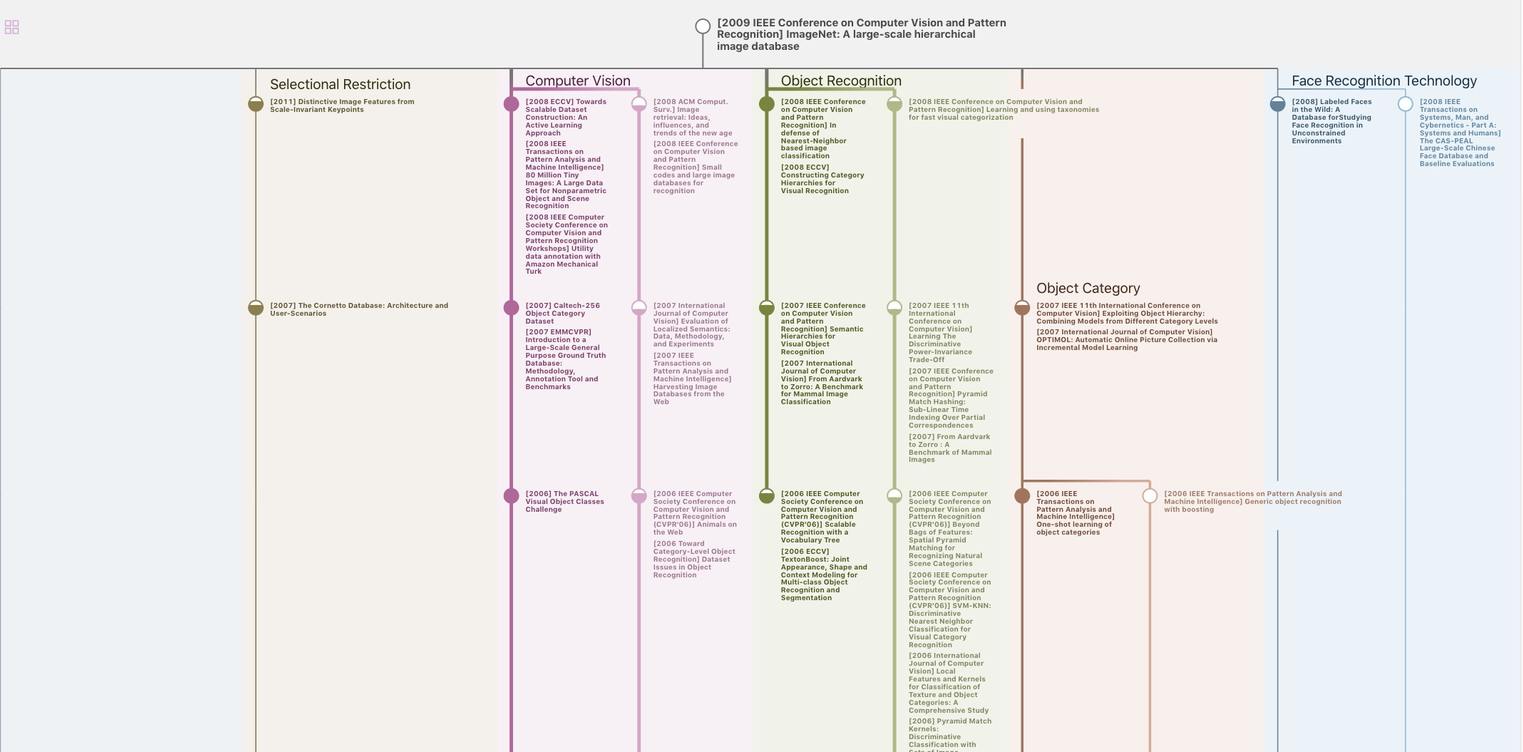
Generate MRT to find the research sequence of this paper
Chat Paper
Summary is being generated by the instructions you defined