Double-space environmental change detection and response strategy for dynamic multi-objective optimize problem
SWARM AND EVOLUTIONARY COMPUTATION(2024)
摘要
Dynamic multi-objective optimization problems (DMOPs) which contain various Pareto-optimal front (PF) and Pareto-optimal set (PS) have gained much attention. Accurate environmental change detection reveals the change degree of DMOPs and contributes the algorithm to quickly respond to the environment changes. In order to fully detect environmental changes and efficiently track front, a double-space environmental change detection and response strategy (DSDRS) is proposed. It could detect whether the environment has changed while explore the change intensity of PF and PS, respectively. Moreover, different response strategies are implemented for PF and PS. For PF environmental changes, a multiple knee points-guided evolutionary strategy (MKGES) is proposed, which is driven by front shape information and adaptively responds to different PF change intensities. For PS environmental changes, a knowledge guided memory strategy (KGMS) is proposed, which guides population evolution based on environmental information. The effectiveness of DSDRS is confirmed by comparison with five evolutionary algorithms on 20 dynamic multiobjective benchmark functions. Simulation results demonstrate that the performance of proposed algorithm is outstanding on test functions with complex changing PF and PS.
更多查看译文
关键词
Dynamic optimization,Multi-objective optimization,Evolutionary algorithm,Double-space change detection
AI 理解论文
溯源树
样例
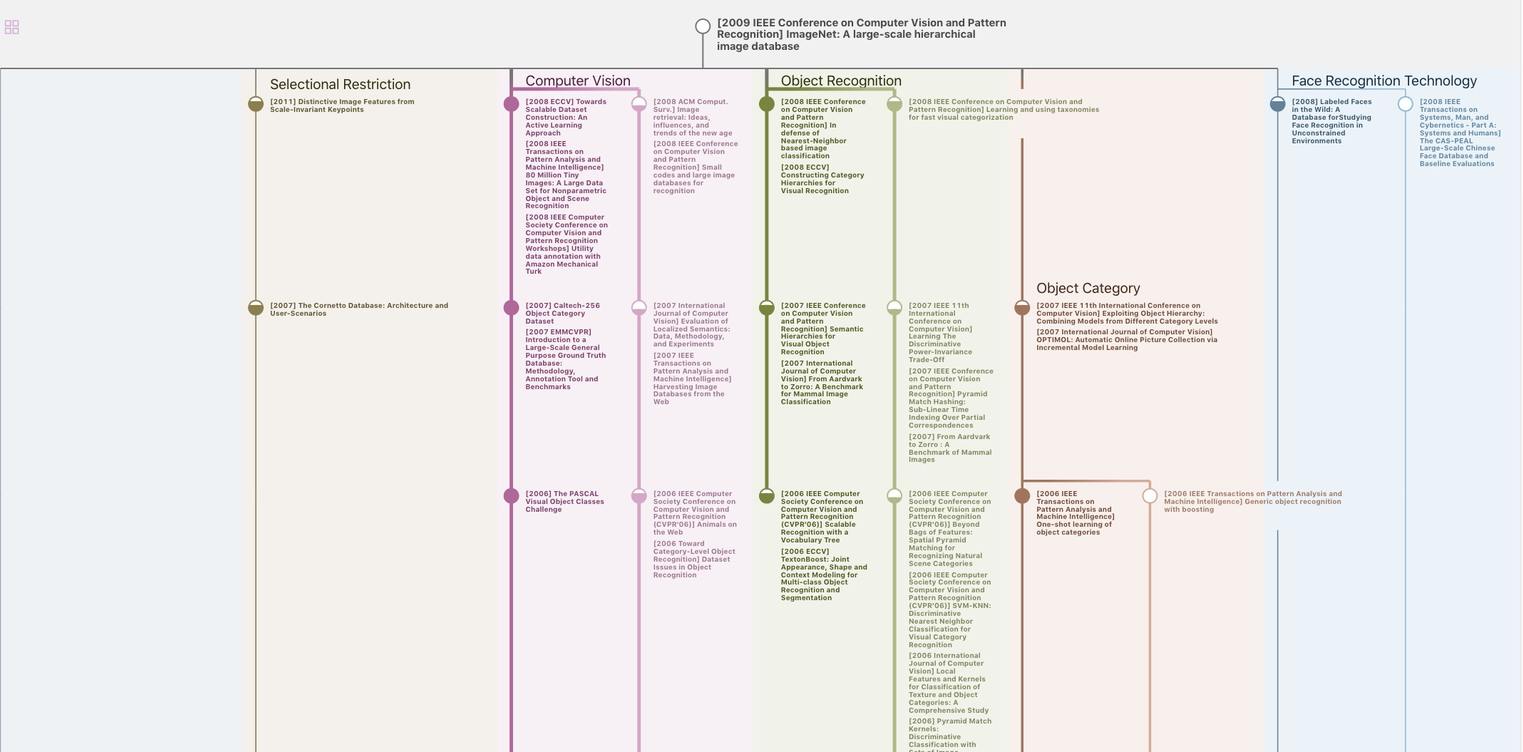
生成溯源树,研究论文发展脉络
Chat Paper
正在生成论文摘要