Self-Supervised Convolutional Clustering for Picking the First Break of Microseismic Recording
IEEE GEOSCIENCE AND REMOTE SENSING LETTERS(2024)
摘要
Accurate first break picking is essential for tunnel microseismic monitoring. Here, we propose a self-supervised convolutional clustering picking (SCCP) method for automatically picking the first break of microseismic recordings. The time-frequency features are decomposed and reconstructed using accurate convolutional encoding and decoding under self-supervision. Then, the autoencoder output is unsupervisedly clustered into useful and invalid waveform sections employing the fuzzy c -means (FCMs) algorithm under long short-term memories, global attention, and self-attention constraints. Furthermore, the first point of the useful waveform is determined as the first break. Our results demonstrate that the proposed SCCP method outperforms the short-term average/long-term average (STA/LTA) and Akaike information criterion (AIC). Compared with PhaseNet, a supervised deep-learning method, the SCCP, produces similar performance without using human-labeled data. Practically, when the signal-to-noise ratio (SNR) is reduced to -6 dB, the average mean absolute error and standard deviation of the picking results remain at 1.12 and 9.19 ms, respectively.
更多查看译文
关键词
Convolutional clustering,first break picking,fuzzy c-means (FCMs) algorithm,microseismic recording,self-supervised deep learning
AI 理解论文
溯源树
样例
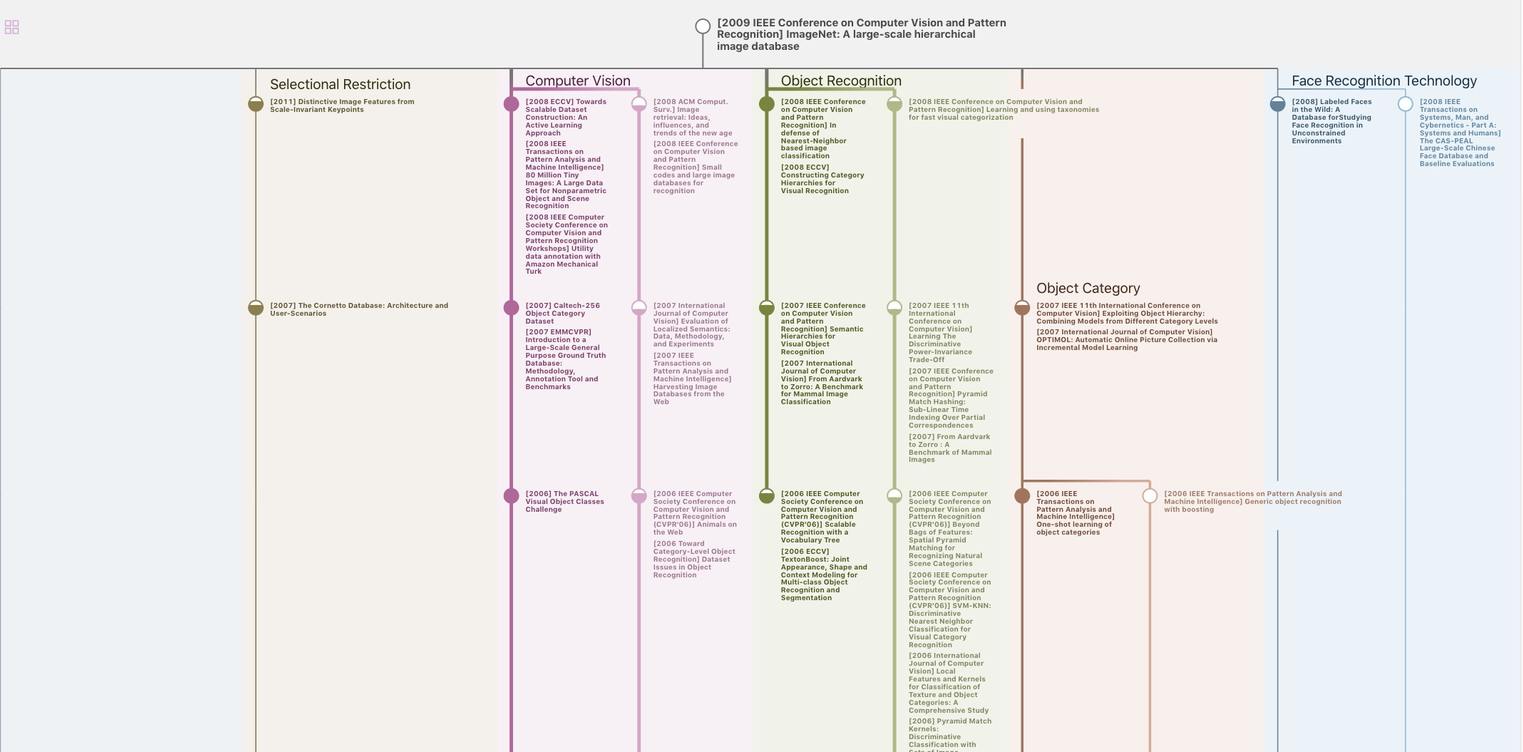
生成溯源树,研究论文发展脉络
Chat Paper
正在生成论文摘要