Enhancing Hyperspectral Image Classification: Leveraging Unsupervised Information With Guided Group Contrastive Learning
IEEE TRANSACTIONS ON GEOSCIENCE AND REMOTE SENSING(2024)
摘要
Deep learning (DL) has demonstrated remarkable performance in the classification of hyperspectral images (HSIs) by leveraging its powerful ability to automatically learn deep spectral-spatial features over the years. Nevertheless, the limited supervisory signals along with a vast number of parameters in deep models still pose critical challenges when utilizing a restricted number of samples for training deep networks. To better handle this issue, this article proposes an end-to-end framework called guided group contrastive learning (GGCL) that adaptively integrates unsupervised information into a supervised contrastive learning framework. The proposed method employs a similarity-guided module that measures the spectral-spatial similarity of unsupervised samples based on supervised signals and effectively groups them. Then, the similarity signals of both supervised and unsupervised data are combined with contrastive learning to achieve intragroup feature aggregation and intergroup feature separation with guided group contrastive loss (GGCLoss). The pivotal characteristic of the proposed method lies in the end-to-end incorporation of unsupervised information with supervised signals for contrastive learning. Experiments on three public HSI datasets demonstrate that the proposed method can achieve better performance than existing state-of-the-art (SOTA) methods. For ease of reproducibility, the code of the proposed GGCL will be publicly available at https://github.com/fanerlight/GGCL_HSI.
更多查看译文
关键词
Contrastive learning,deep learning (DL),end-to-end framework,hyperspectral image (HSI) classification,spectral-spatial similarity
AI 理解论文
溯源树
样例
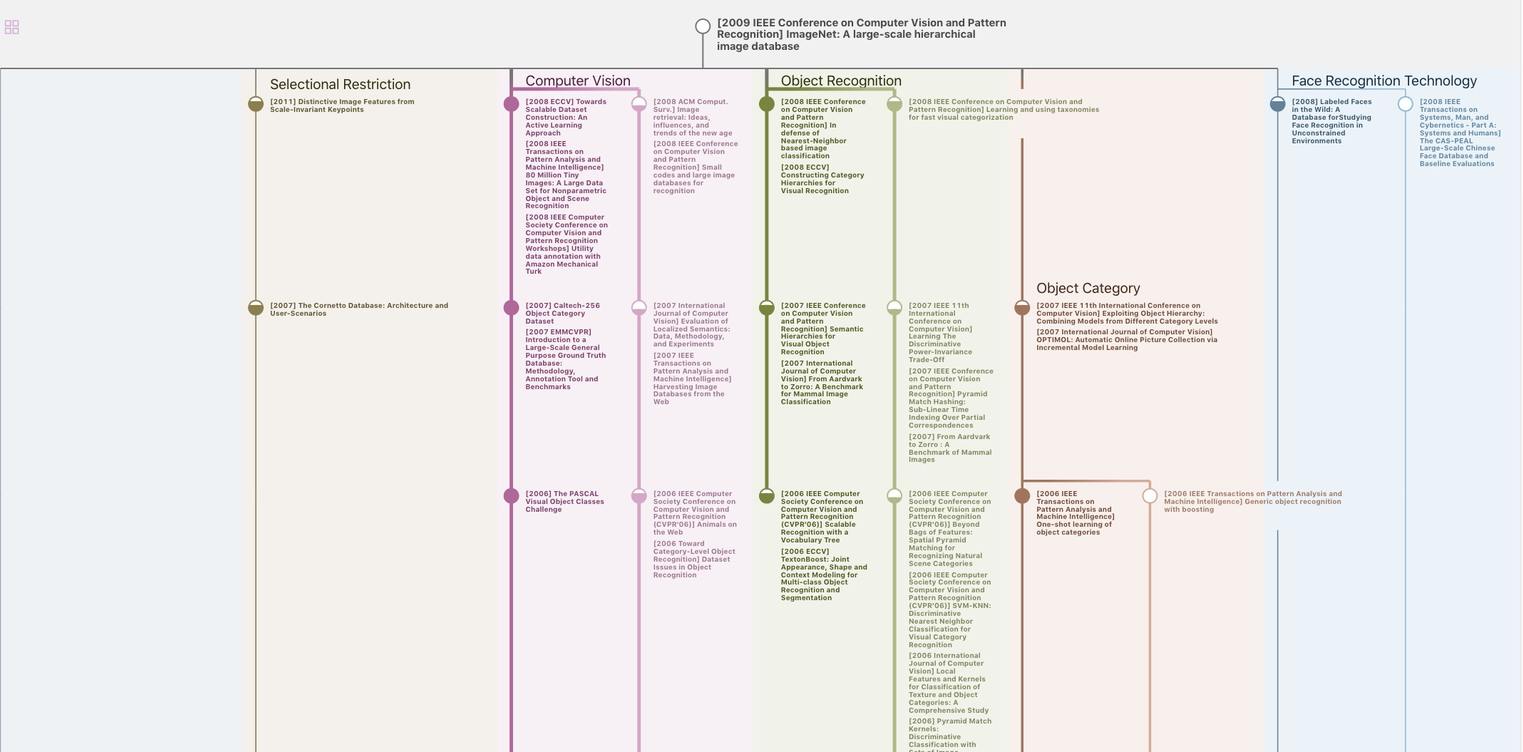
生成溯源树,研究论文发展脉络
Chat Paper
正在生成论文摘要