Model-Based Fairness Metric for Speaker Verification.
2023 IEEE Automatic Speech Recognition and Understanding Workshop (ASRU)(2023)
摘要
Ensuring that technological advancements benefit all groups of people equally is crucial. The first step towards fairness is identifying existing inequalities. The naive comparison of group error rates may lead to wrong conclusions. We introduce a new method to determine whether a speaker verification system is fair toward several population subgroups. We propose to model miss and false alarm probabilities as a function of multiple factors, including the population group effects, e.g., male and female, and a series of confounding variables, e.g., speaker effects, language, nationality, etc. This model can estimate error rates related to a group effect without the influence of confounding effects. We experiment with a synthetic dataset where we control group and confounding effects. Our metric achieves significantly lower false positive and false negative rates w.r.t. baseline. We also experiment with VoxCeleb and NIST SRE21 datasets on different ASV systems and present our conclusions.
更多查看译文
关键词
Fairness,Speaker verification
AI 理解论文
溯源树
样例
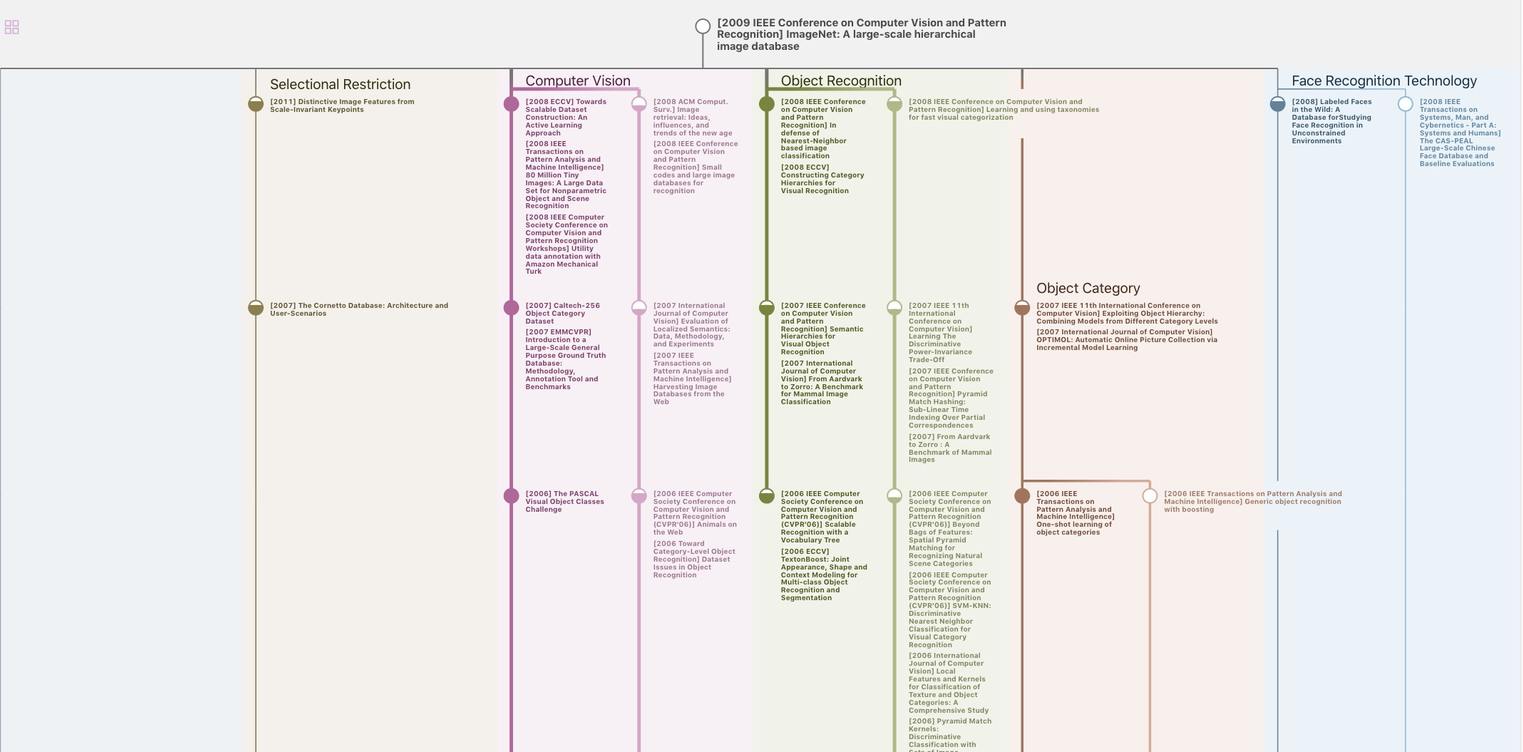
生成溯源树,研究论文发展脉络
Chat Paper
正在生成论文摘要