Entity-Relation Extraction as Full Shallow Semantic Dependency Parsing
IEEE-ACM TRANSACTIONS ON AUDIO SPEECH AND LANGUAGE PROCESSING(2024)
摘要
Entity-relation extraction is the essential information extraction task and can be decomposed into Named Entity Recognition (NER) and Relation Extraction (RE) subtasks. This paper proposes a novel joint entity-relation extraction method that models the entity-relation extraction task as full shallow semantic dependency graph parsing. Specifically, it jointly and simultaneously converts the entities and relation mentions as the edges of the semantic dependency graph to be parsed and their types as the labels. This model also integrates the advantages of multiple feature tagging methods and enriches the token representation. Furthermore, second-order scoring is introduced to exploit the relationships between entities and relations, which improves the model performance. Our work is the first time to fully model entities and relations into a graph and uses higher-order modules to address their interaction problems. Compared with state-of-the-art scores on five benchmarks (ACE04, ACE05, CoNLL04, ADE, and SciERC), empirical results show that our proposed model makes significant improvements and demonstrates its effectiveness and practicability.
更多查看译文
关键词
Named entity recognition,relation extraction,semantic dependency parsing,second-order parsing
AI 理解论文
溯源树
样例
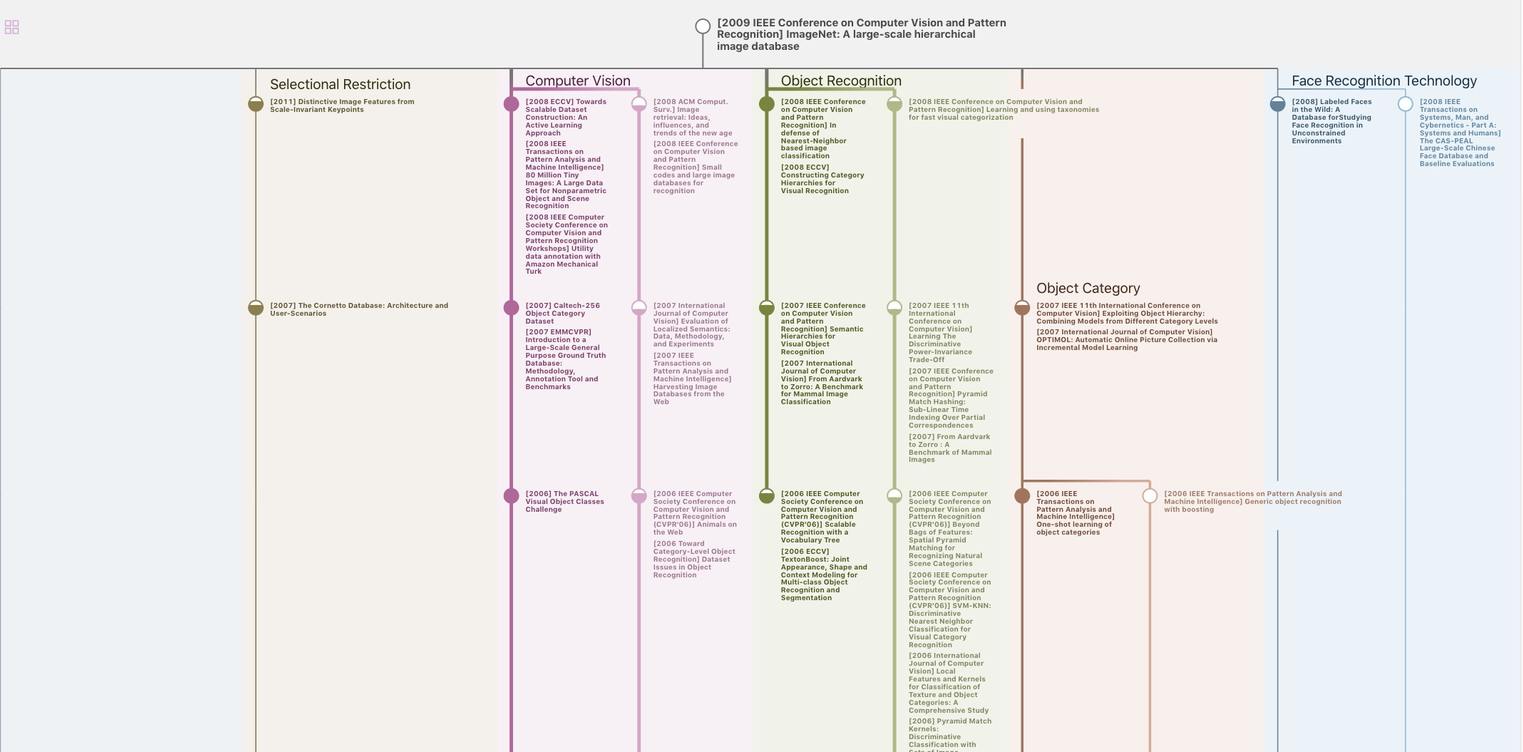
生成溯源树,研究论文发展脉络
Chat Paper
正在生成论文摘要