Text-to-Speech for Low-Resource Agglutinative Language With Morphology-Aware Language Model Pre-Training
IEEE-ACM TRANSACTIONS ON AUDIO SPEECH AND LANGUAGE PROCESSING(2024)
摘要
Text-to-Speech (TTS) aims to convert the input text to a human-like voice. With the development of deep learning, encoder-decoder based TTS models perform superior performance, in terms of naturalness, in mainstream languages such as Chinese, English, etc. Note that the linguistic information learning capability of the text encoder is the key. However, for TTS of low-resource agglutinative languages, the scale of the paired data is limited. Therefore, how to extract rich linguistic information from small-scale text data to enhance the naturalness of the synthesized speech, is an urgent issue that needs to be addressed. In this paper, we first collect a large unsupervised text data for BERT-like language model pre-training, and then adopt the trained language model to extract deep linguistic information for the input text of the TTS model to improve the naturalness of the final synthesized speech. It should be emphasized that in order to fully exploit the prosody-related linguistic information in agglutinative languages, we incorporated morphological information into the language model training and constructed a morphology-aware masking based BERT model (MAM-BERT). Experimental results based on various advanced TTS models validate the effectiveness of our approach. Further comparison of the various data scales also validates the effectiveness of our approach in low-resource scenarios.
更多查看译文
关键词
Text-to-speech (TTS),agglutinative,morphology,language modeling,pre-training
AI 理解论文
溯源树
样例
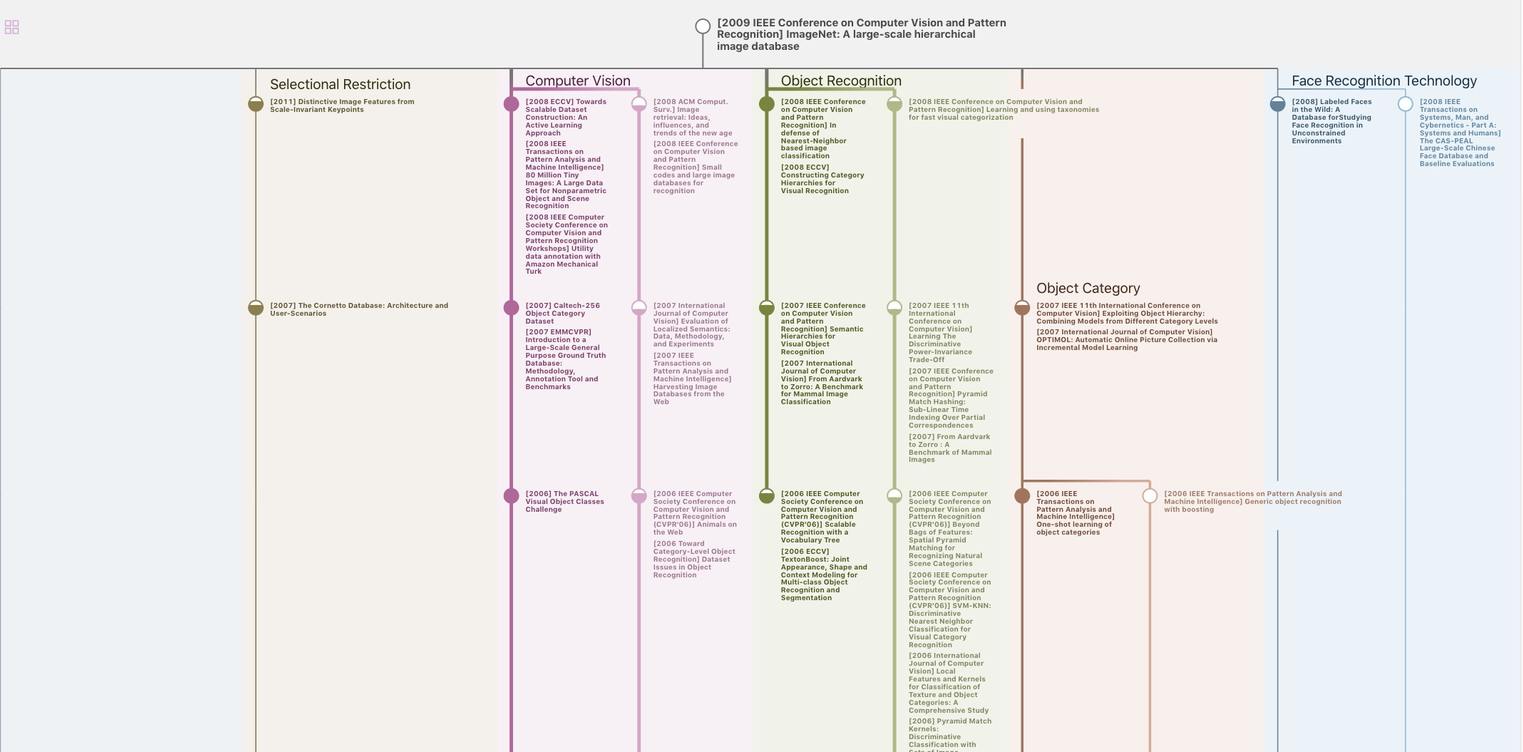
生成溯源树,研究论文发展脉络
Chat Paper
正在生成论文摘要