Squeezing adaptive deep learning methods with knowledge distillation for on-board cloud detection
ENGINEERING APPLICATIONS OF ARTIFICIAL INTELLIGENCE(2024)
摘要
Cloud detection is a pivotal satellite image pre-processing step that can be performed on board a satellite to tag useful images. It can reduce the amount of data to downlink by pruning the cloudy areas, or to make a satellite more autonomous through data-driven acquisition re-scheduling. This study approaches this task with a no-new-Net (nnU-Net), an adaptive framework to perform meta-learning of a segmentation network over various datasets. Unfortunately, such models are commonly memory-inefficient due to their (very) large architectures which renders them infeasible to be deployed in hardware-constrained edge devices. To benefit from such automatically-elaborated models, nnU-Nets are compressed with knowledge distillation into much smaller U-Nets that can fit the target hardware. The experiments, performed over Sentinel-2 and Landsat-8 images, and over the images simulating the imagery expected on board a new mission (Phi-Sat-2), revealed that nnU-Nets deliver state-of-the-art performance without any manual design. The proposed approach was ranked within the top 7% best solutions (across 847 teams) in the On Cloud N: Cloud Cover Detection Challenge, where it reached the Jaccard index of 0.882 over more than 10 thousand unseen Sentinel-2 images (the winners obtained Jaccard of 0.897, the baseline U-Net with the residual network backbone: 0.817, and the classic Sentinel-2 image thresholding: 0.652). The experimental study showed that knowledge distillation enables to elaborate dramatically smaller (almost 280x) U-Nets while maintaining the segmentation capabilities of nnU-Nets. Such compact U-Nets offer fast inference, as segmenting a 19.4 x 19.4 km scene took approximately 12 s on the Intel Movidius Myriad-2 processing unit.
更多查看译文
关键词
Cloud segmentation,Multispectral images,On-board processing,Knowledge distillation,Deep model compression
AI 理解论文
溯源树
样例
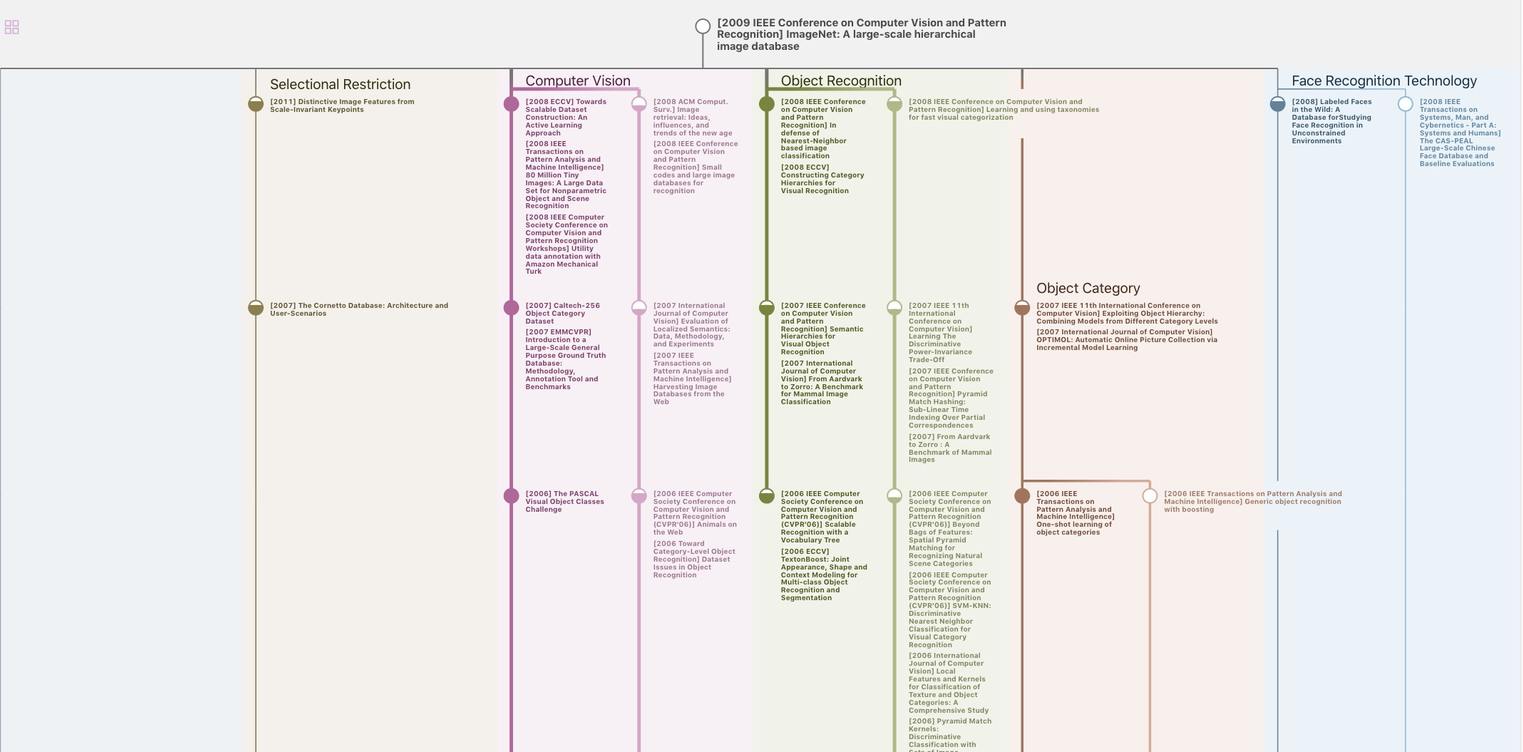
生成溯源树,研究论文发展脉络
Chat Paper
正在生成论文摘要