Joint Network Combining Dual-Attention Fusion Modality and Two Specific Modalities for Land Cover Classification Using Optical and SAR Images
IEEE JOURNAL OF SELECTED TOPICS IN APPLIED EARTH OBSERVATIONS AND REMOTE SENSING(2024)
摘要
Optical and synthetic aperture radar (SAR) images provide various complementary information on land properties, which can substantially improve the accuracy of land cover classification because of the fusion of their multisource information. However, excellent extraction of the discriminative information of optical and SAR images and effective integration of the interpretation information of multisource data remain challenging issues. In this study, we have proposed a novel joint network that combines a dual-attention fusion modality and two specific modalities to achieve superior land cover classification maps, which has two modes of encoding-level fusion (JoiTriNet-e) and decoding-level fusion (JoiTriNet-d). We first proposed a strategy for the fusion modality and specific modalities joint learning, the goal of which was to simultaneously find three mapping functions that project optical and SAR images separately and together into semantic maps. Two architectures were constructed using the proposed strategy, which improved the performance of multisource and single-source land cover classification. To aggregate the heterogeneous features of optical and SAR images more reasonably, we designed a multimodal dual-attention fusion module. Experiments were conducted on two multisource land cover datasets, among which comparison experiments highlighted the superiority and robustness of our model, and ablation experiments verified the effectiveness of the proposed architecture and module.
更多查看译文
关键词
Convolutional neural network (CNN),data fusion,deep learning (DL),land cover classification,multimodal semantic segmentation,optical images,synthetic aperture radar (SAR) images
AI 理解论文
溯源树
样例
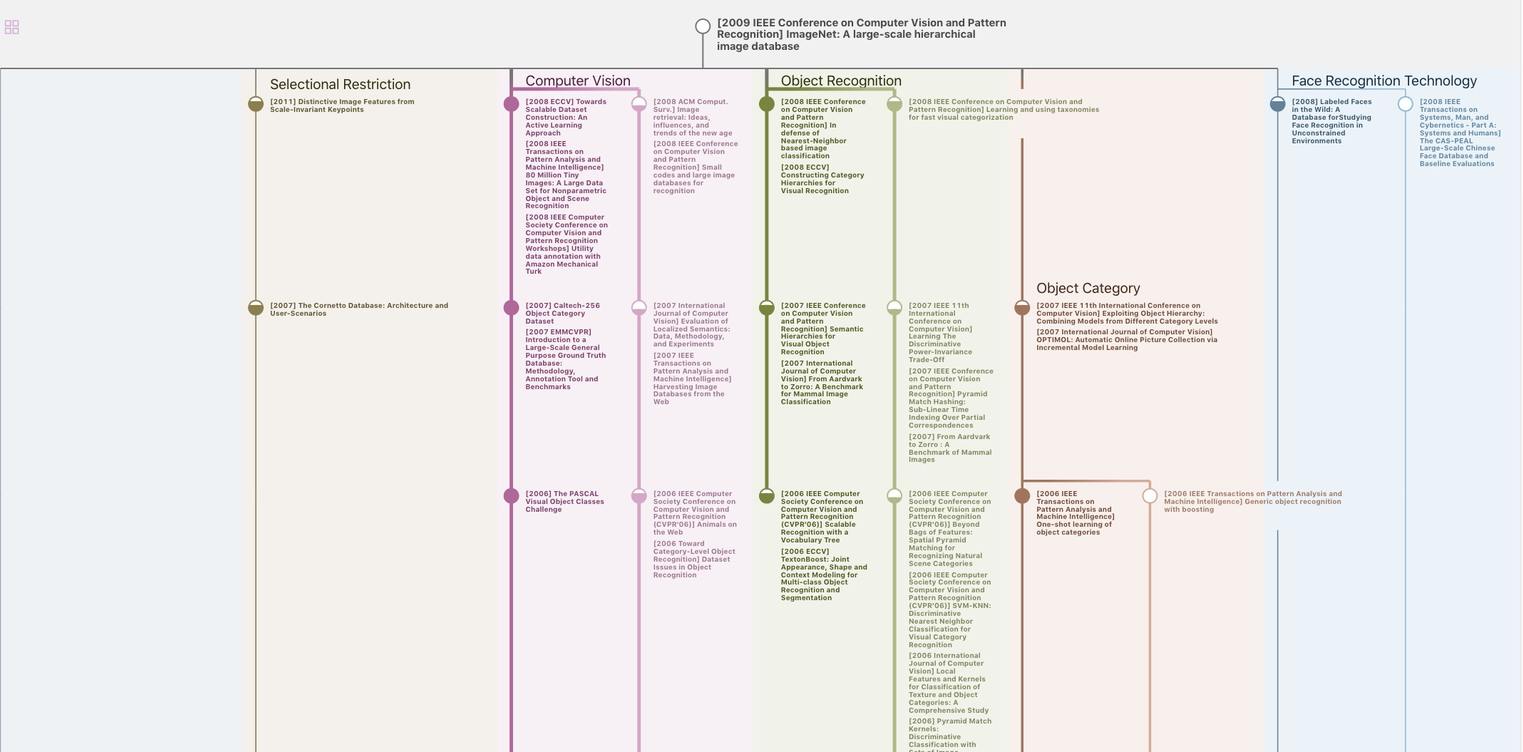
生成溯源树,研究论文发展脉络
Chat Paper
正在生成论文摘要