Promote knowledge mining towards open-world semi-supervised learning
PATTERN RECOGNITION(2024)
摘要
Deep learning models often rely on a large number of labeled data to achieve good performance. However, labeling such a large number of data requires exhaustive labor efforts. In recent years, a pivotal research direction is to generalize deep learning models to learn from not only unlabeled data of seen classes but also data of novel classes which are not predefined, known as open -world semi -supervised learning (openworld SSL). Existing works tackled this challenging task by manually designing different optimizations for labeled/unlabeled data and seen/novel classes. In this paper, we propose a simple unified framework that can be applied to all images and all classes in the same form. In this framework, we exploit the Sinkhorn-Knopp algorithm to overcome the overconfidence issue of pseudo labels on seen classes and thus lead to a more balanced distribution of seen and novel classes. To reduce the intra-class variance and avoid model collapse, we take as input two different views of an image and regard one's prediction as the other's pseudo label. However, in a unified framework, the model converges much faster on the seen classes than those novel classes. To balance them and encourage knowledge transfer from seen classes to novel classes, we further propose mixing up any two training images during our unified optimization. Extensive experiments on three benchmarks (i.e., CIFAR-10, CIFAR-100, and ImageNet-100) show that our unified framework achieved comparable performance with existing state-of-the-art methods. Our code is available on https://github.com/happytianhao/OWSSL.
更多查看译文
关键词
Open world semi-supervised learning,Representation learning,Novel class discovery
AI 理解论文
溯源树
样例
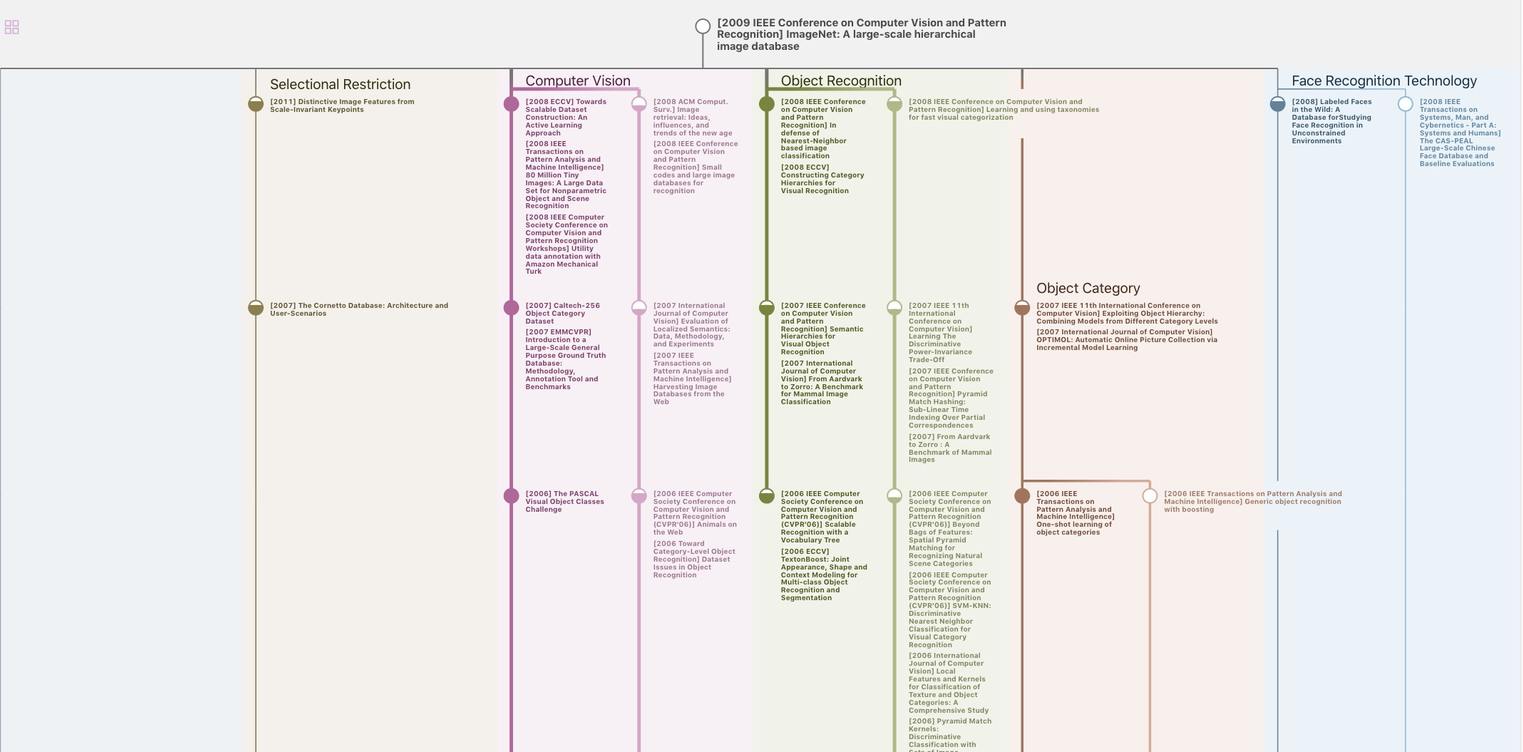
生成溯源树,研究论文发展脉络
Chat Paper
正在生成论文摘要