An optimized CNN-BiLSTM network for bearing fault diagnosis under multiple working conditions with limited training samples
NEUROCOMPUTING(2024)
Abstract
Aiming at limitations in fully exploiting the temporal correlation features of the original signals, expensive cost in parameter tuning, and difficulties in obtaining sufficient training data under multiple working conditions, this paper proposes an optimized Convolutional Neural Network (CNN) with Bi-directional Long Short -Term Memory (BiLSTM) scheme for bearing fault diagnosis under multiple working conditions with limited training samples. A CNN-BiLSTM network is developed to obtain precise fault features and high detection accuracy by extracting high -dimensional and temporal correlation features of raw vibration signals. An improved particle swarm optimization (PSO) algorithm is leveraged to optimize the training hyperparameters of the CNN-BiLSTM network for further advances in fault diagnosis performance. The optimized CNN-BiLSTM network is regarded as a pre -trained model and transferred to new working conditions to achieve satisfactory fault diagnosis results based on limited training samples. Several comprehensive experiments are implemented to confirm the excellent performance of the proposed schemes, especially efficiently addressing the challenges of model training and fault diagnosis in new working conditions with scarce samples.
MoreTranslated text
Key words
Bearing,Fault diagnosis,CNN,BiLSTM,PSO,Transfer learning
AI Read Science
Must-Reading Tree
Example
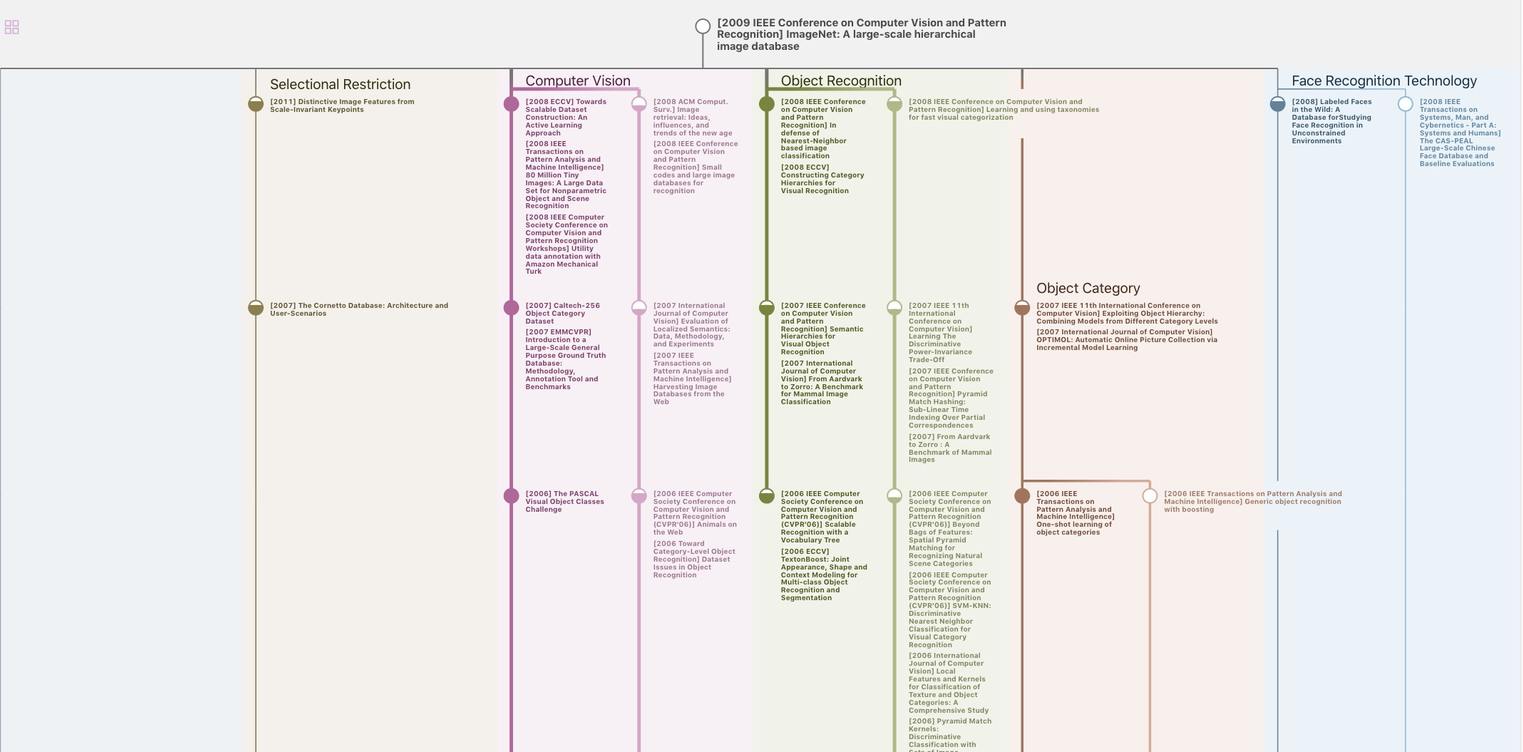
Generate MRT to find the research sequence of this paper
Chat Paper
Summary is being generated by the instructions you defined