Forecasting resource usage pattern changes in clouds via contrast graph-evolution learning
FUTURE GENERATION COMPUTER SYSTEMS-THE INTERNATIONAL JOURNAL OF ESCIENCE(2024)
摘要
The increasing large-scale workload trace collected by datacenter monitors has provided great potential for workload prediction. However, resource usage pattern changes of workloads often exist, which are usually caused by user demand changes, workload re -locations or other reasons. If the patterns of resource usage change, the accuracy of prediction may degrade significantly. Hence, how to forecast such changes brings an open and challenging issue. In this paper, we present a novel method named CEGWP. CEGWP uses shapelets to explicitly identify resource usage patterns in a fine-grained level. Specifically. a two -level importance based shapelet extraction mechanism is designed to mine new usage pattern changes in temporal dimension, and a multi -view contrast graph -evolution learning algorithm is designed to fuse the interference among resource usage patterns of different workloads in spatial dimension. With both temporal extraction of shapelets from each single workload and spatial interference of different workloads, a spatio-temporal GNN-based TCN encoder is developed to forecast the resource usage pattern changes. CEGWP is jointly trained in an end -toend manner and is evaluated using real trace data. Experimental results show that CEGWP achieves notable improvements by up to 62.15% against existing prediction methods and indicate the potential to be deployed into real -world cloud datacenters.
更多查看译文
关键词
Workload prediction,Cloud computing,Graph neural network,Temporal convolutional network,Resource management,Datacenter
AI 理解论文
溯源树
样例
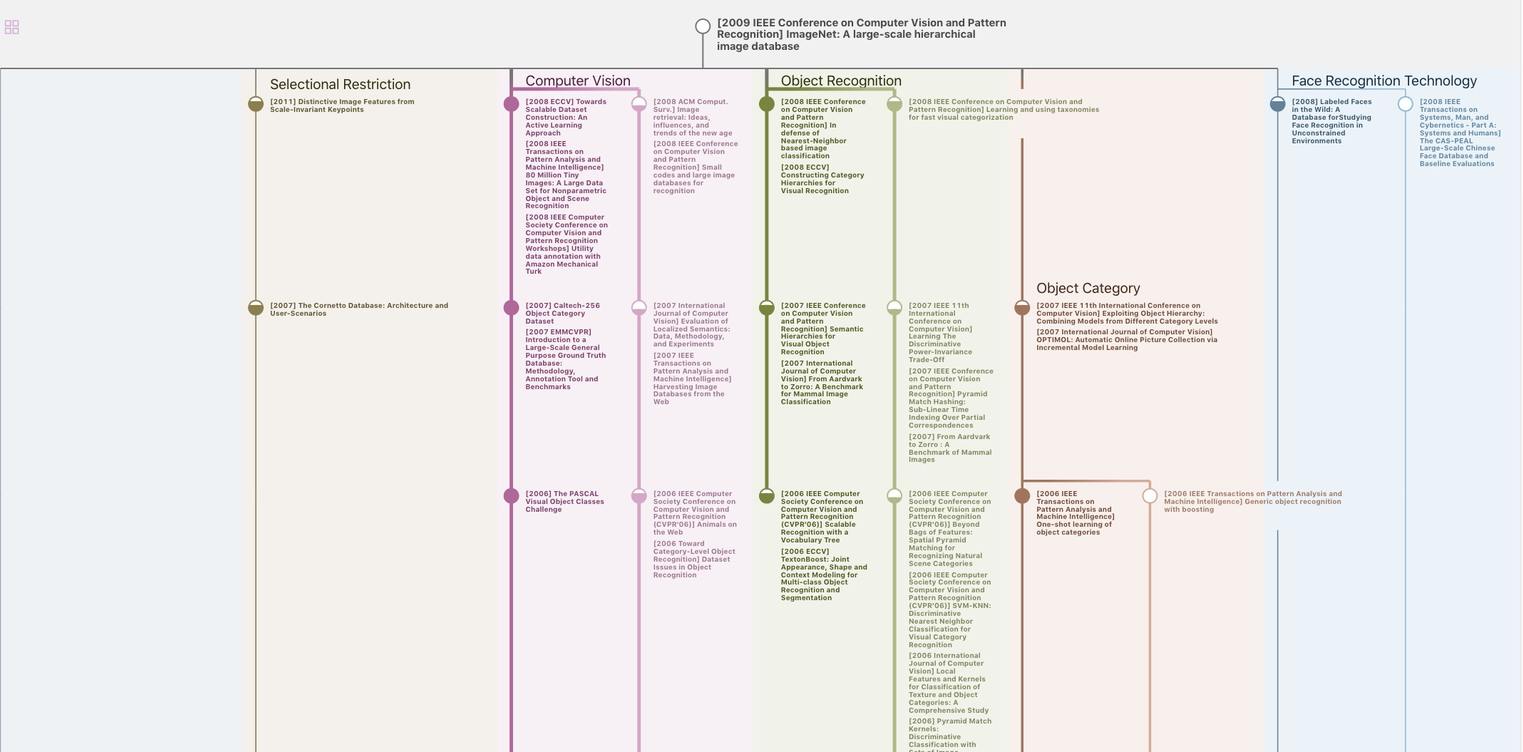
生成溯源树,研究论文发展脉络
Chat Paper
正在生成论文摘要