Detection of Voltage Fault in Lithium-Ion Battery Based on Equivalent Circuit Model-Informed Neural Network
IEEE TRANSACTIONS ON INSTRUMENTATION AND MEASUREMENT(2024)
Abstract
Voltage fault diagnosis is critical for detecting and identifying the lithium (Li)-ion battery failure. This article proposes a voltage fault diagnosis algorithm based on an equivalent circuit model-informed neural network (ECMINN) method for Li-ion batteries, which aims to learn the voltage fault observer by embedding the equivalent circuit model (ECM) into neural network structures. This method directly embeds the deterministic mechanism part in the ECM and designs the uncertain part into neural networks, which takes advantage of the high precision of the physical model and the strong nonlinear processing ability of the neural network to improve the fault diagnosis effect. In this method, the voltage prediction module and residual-based parameter calibration module are developed to describe state space equations and automatically learn the state parameters and model parameters, respectively. Moreover, a fault score specifically designed by combining the identified model parameters is presented, which is proved to be effectively in detecting different fault types. Finally, experiments on two public datasets are conducted, by which the proposed ECMINN method is demonstrated to be effective and accurate in predicting voltage data and detecting faults. Comparison with three other state-of-the-art methods also reveals the advantages of the proposed ECMINN method.
MoreTranslated text
Key words
Fault diagnosis,lithium (Li)-ion battery,physics-informed neural networks,voltage fault
AI Read Science
Must-Reading Tree
Example
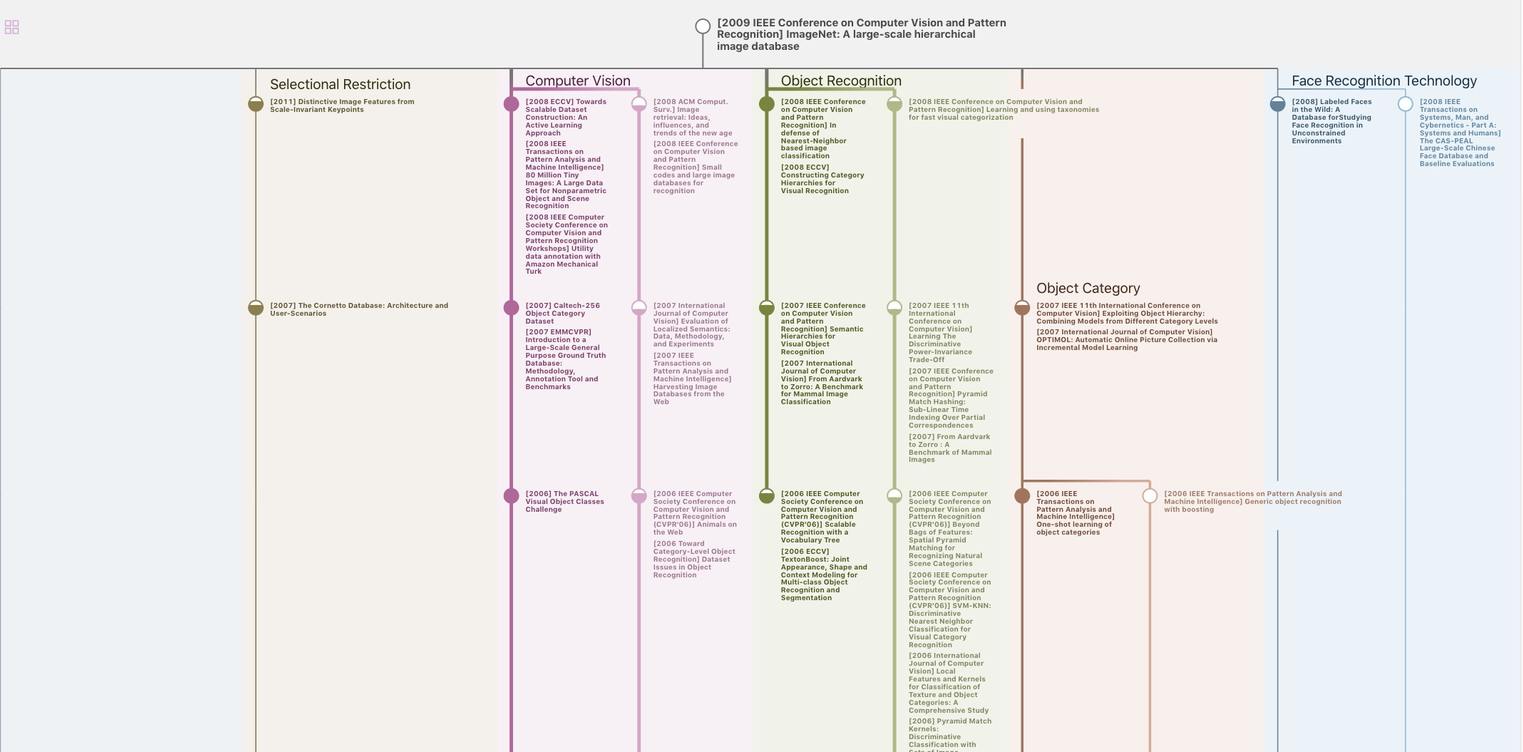
Generate MRT to find the research sequence of this paper
Chat Paper
Summary is being generated by the instructions you defined