QGFORMER: Quantum-Classical Hybrid Transformer Architecture for Gravitational Wave Detection
2023 20th International Computer Conference on Wavelet Active Media Technology and Information Processing (ICCWAMTIP)(2023)
摘要
Gravitational Wave (GW) detection is a pivotal field in astrophysics, gaining prominence for its role in deciphering cosmic phenomena. Traditional machine learning algorithms in GW detection, while effective, grapple with challenges in parallelism, scalability, efficiency in handling high-dimensional data and noise interference. To mitigate these limitations, this paper proposes the Quantum-Gravitational Transformer (QGFormer), a novel quantum-classical hybrid transformer for gravitational wave detection. The QGFormer consists of a vision transformer encoder and a quantum classifier following the autoencoder architecture, which first segments the original input signal into small patches and maps them to higher dimensions, and then inputs the higher-dimensional feature map into the quantum classifier for prediction. The excellent parallelism inherent in the multi-head self-attention mechanism and quantum computing makes QGFormer expected to achieve high computational efficiency and scalability on quantum computing devices. Additionally, the super-density coding and quantum state compression techniques that could be used in these devices help mitigate the effects of noise. In experiments on the Gravitational Spy dataset, which utilizes data collected by the LIGO telescope, our results show that QGFormer outperforms the comparison models in accuracy and F1-score, demonstrating the promising future of quantum machine learning approaches in this field.
更多查看译文
关键词
Quantum machine learning,Gravitational wave detection,Glitch classification
AI 理解论文
溯源树
样例
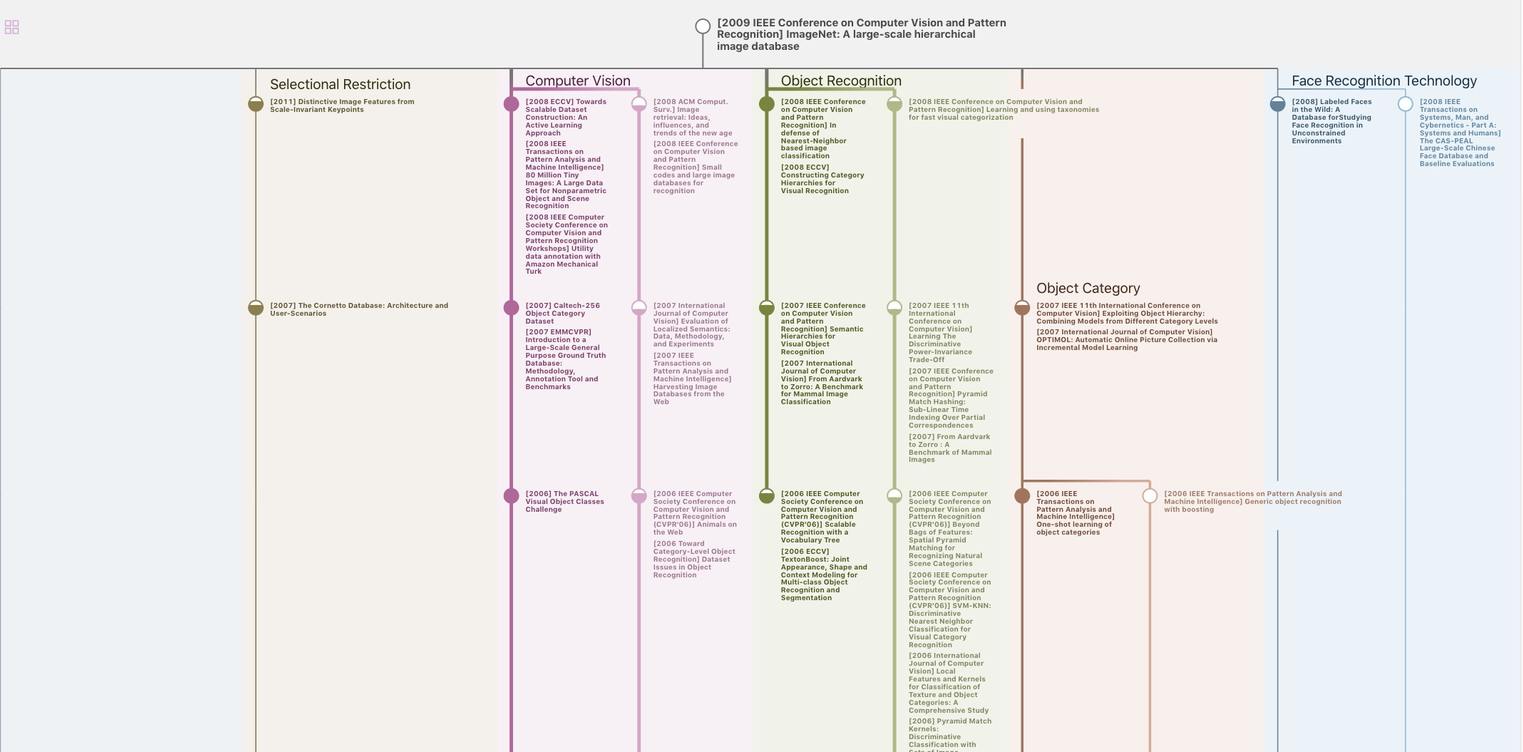
生成溯源树,研究论文发展脉络
Chat Paper
正在生成论文摘要