An Enhanced Fusion Model for Android Malware Detection Leveraging Multi-Code Fragment Features and Fuzzy Hashing
2023 20th International Computer Conference on Wavelet Active Media Technology and Information Processing (ICCWAMTIP)(2023)
摘要
Traditional methods for detecting Android malware require large-scale datasets and complex feature engineering, which are unable to adapt to complex malicious features. Additionally, feature engineering based on fuzzy hash algorithms is
w
idely used but susceptible to bypass attacks by adversaries. To address these issues, this paper proposes an Android malware detection model that multiple Android code snippet features with fuzzy hash features. Simulation experiments demonstrate that using fused fuzzy hash features improves the accuracy of Android malware detection by 7.03%, precision by 6.23%, recall by 13.78%, and F1 score by 9.88% compared to using single fuzzy hash features. The proposed detection method not only focuses on partial features of function call sequences and behaviors of malicious applications but also considers the correlation between call sequences and behaviors, enabling it to adapt to more complex Android malware detection scenarios.
更多查看译文
关键词
Fuzzy Hashing,Feature Fusion,Random Forest,Android Malware Detection
AI 理解论文
溯源树
样例
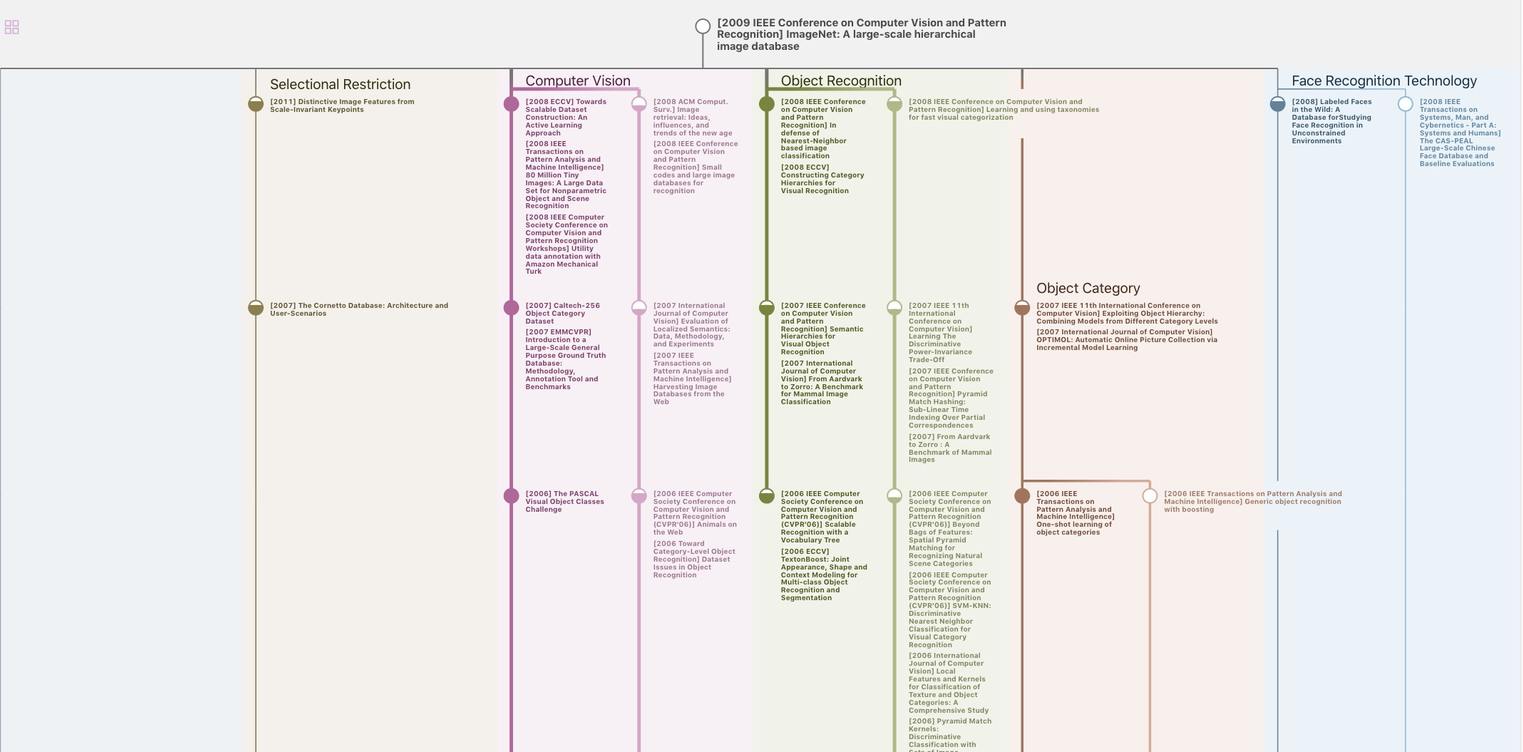
生成溯源树,研究论文发展脉络
Chat Paper
正在生成论文摘要