A Combined ELM and CNN Model Architecture for Accurate Viral Family DNA Classification
2023 Eleventh International Conference on Intelligent Computing and Information Systems (ICICIS)(2023)
摘要
Viruses are an important group of pathogens that can cause a wide range of diseases in humans and other organisms. Accurate and efficient classification of viruses is crucial for the development of diagnostic tools and therapies, as well as for understanding the evolutionary relationships and diversity of these viruses. There has been a surge of interest in the application of machine learning and deep learning approaches for DNA viral classification in recent years. These methods can effectively handle the large and complex datasets that are often encountered in this context. A deep learning architecture is proposed in this study for the classification of DNA virus into its respective family. The model was trained on a newly collected dataset that covers a wide variety of viral family genomes. The architecture utilizes Convolutional Neural Networks (CNN) as a feature extraction layer and Extreme Learning Machines (ELMs) as a classification layer, using k-mer encoding. The use of CNN as a feature extraction layer and ELMs for classification allows for the effective and efficient classification of DNA virus into their diverse virus families. The proposed architecture was tested on a dataset obtained in FASTA format, as provided by the National Center for Biotechnology Information (NCBI) . The dataset consists of the major 10 virus families. The architecture achieved an accuracy of 94.54% on the test set, with a confusion matrix showing strong performance across all 10 virus families. A comparative analysis was also performed with other k-mer based machine learning algorithms and the proposed architecture achieved the highest accuracy amongst them.
更多查看译文
关键词
Convolutional neural networks,Extreme learning machines,encoding techniques,Deep neural networks
AI 理解论文
溯源树
样例
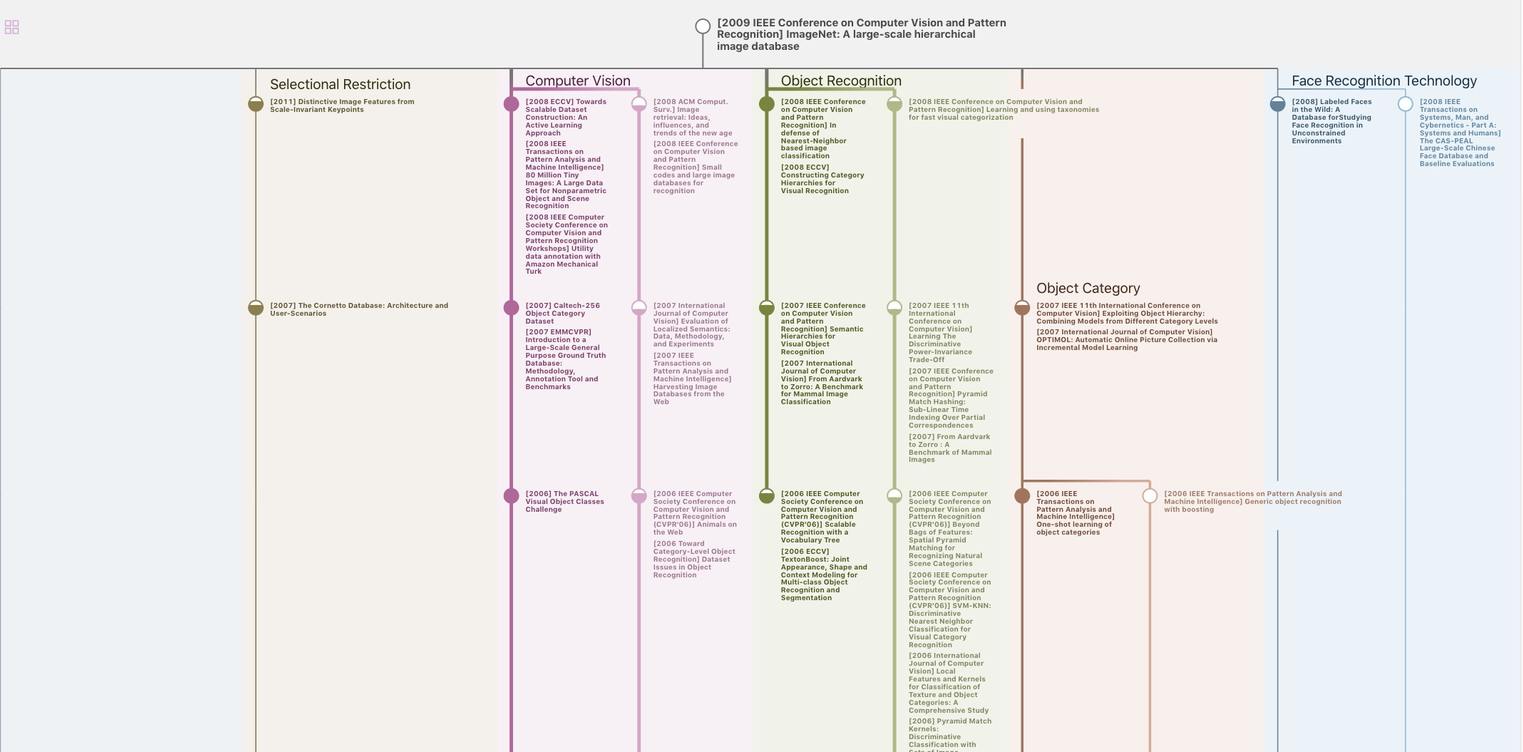
生成溯源树,研究论文发展脉络
Chat Paper
正在生成论文摘要