Group Attention-based Multi-wave Graph Learning for Dementia Diagnosis.
2023 IEEE International Conference on Bioinformatics and Biomedicine (BIBM)(2023)
摘要
Dementia is a syndrome caused by various diseases and injuries to the brain. Considering the differences in brain activity among different dementias, Electroencephalogram (EEG) has been used for dementia diagnosis. EEG can be divided into different brain waves according to frequency, each of which is associated with a distinct brain function. However, current EEG-based methods rarely consider the correlation between different brain waves. To address this challenge, we propose a group attention-based multi-wave graph learning (GAMGL) framework for dementia diagnosis. In order to effectively capture the hidden complementary information between different waves, GAMGL introduces the multi-scale grouped representation module and the group attention module, which extract multi-scale features from various brain waves and adaptively fuse the multi-wave and multi-scale features by using the group attention mechanism. Then, to reveal the hidden connections among subjects, a population graph with fused features as graph nodes is constructed, and efficient dementia diagnosis is performed by graph convolution network. GAMGL is evaluated on an in-house EEG dataset containing three types of dementia, namely Alzheimer’s disease, Vascular cognitive impairment and Frontotemporal dementia. Experimental results show that GAMGL outperforms state-of-the-art methods and can be an efficient and accurate framework for dementia diagnosis.
更多查看译文
关键词
Electroencephalogram,Group attention mechanism,Multi-wave representation learning,Dementia diagnosis,Graph learning
AI 理解论文
溯源树
样例
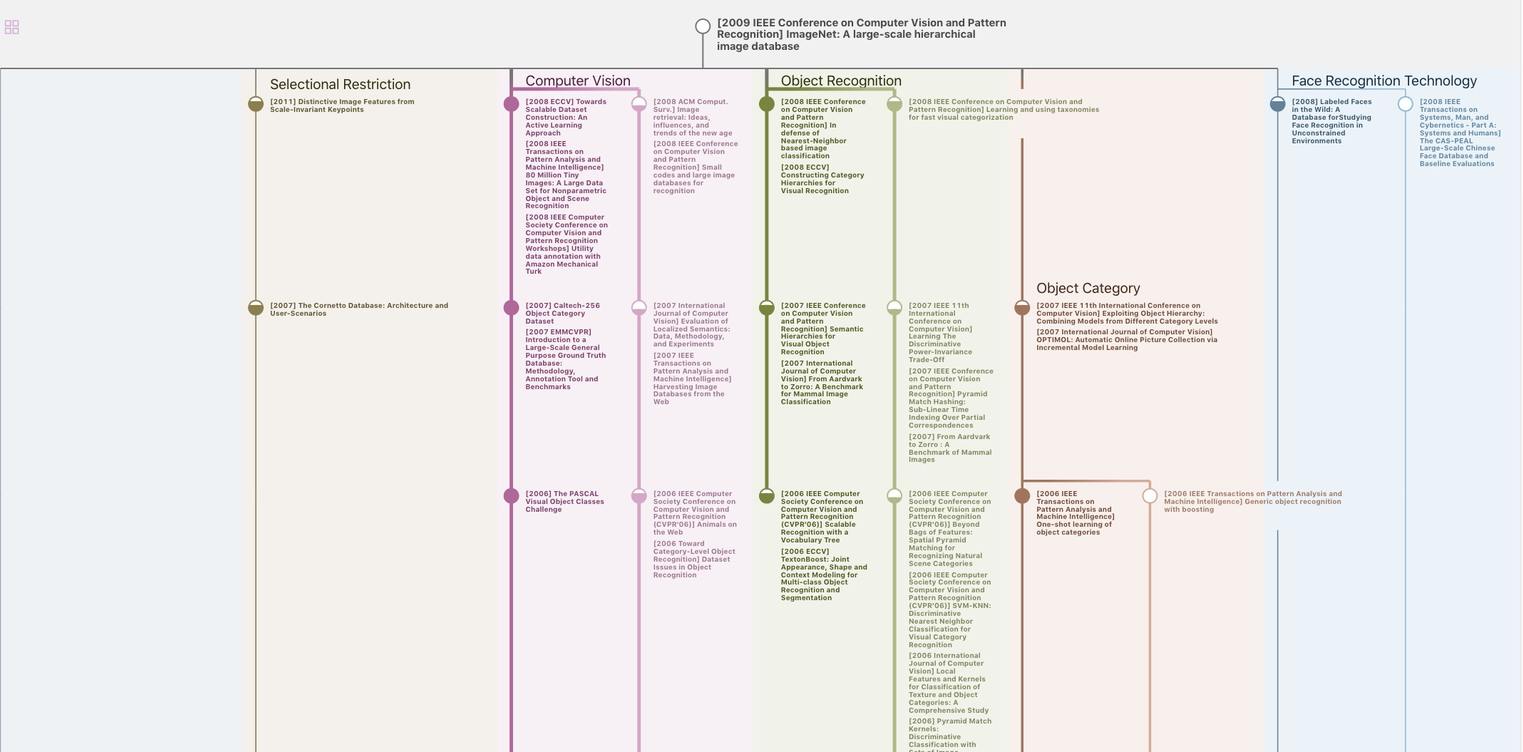
生成溯源树,研究论文发展脉络
Chat Paper
正在生成论文摘要