Deep k-Space Partition-Based Convolutional Networks for Fast Multimodal MRI Reconstruction.
2023 IEEE International Conference on Bioinformatics and Biomedicine (BIBM)(2023)
摘要
Magnetic Resonance Imaging (MRI) with multiple modalities is commonly used for diagnosis, but it is associated with an inherently slow acquisition process. To accelerate multi-modal MRI, recent studies explored the merits of using a fully-sampled reference modality (RM) as a guidance to reconstruct the query modalities (QMs) from their undersampled k-space data via convolutional neural networks (CNNs). However, even aided by the RM, the reconstruction of highly undersampled QM data is still suffering from aliasing artifacts. To enhance reconstruction quality, we suggest to further release the guiding power of the RM data via generating its multiscale variants. To this end, we simultaneously partition the k-space of the RM and QM into several subregions with gradually increasing sizes. We then proposed a k-Space Partition-based Convolutional Network (kSPCN) to fully use the partitioned RM and QM data to perform QM reconstruction subregion by subregion. Extensive experiments on different query modalities and acceleration rates demonstrate that kSPCN consistently outperforms state-of-the-art methods and can preserve anatomical structure faithfully up to 12-fold undersampling.
更多查看译文
关键词
MRI reconstruction,k-space partition,convolutional networks,multimodal MRI
AI 理解论文
溯源树
样例
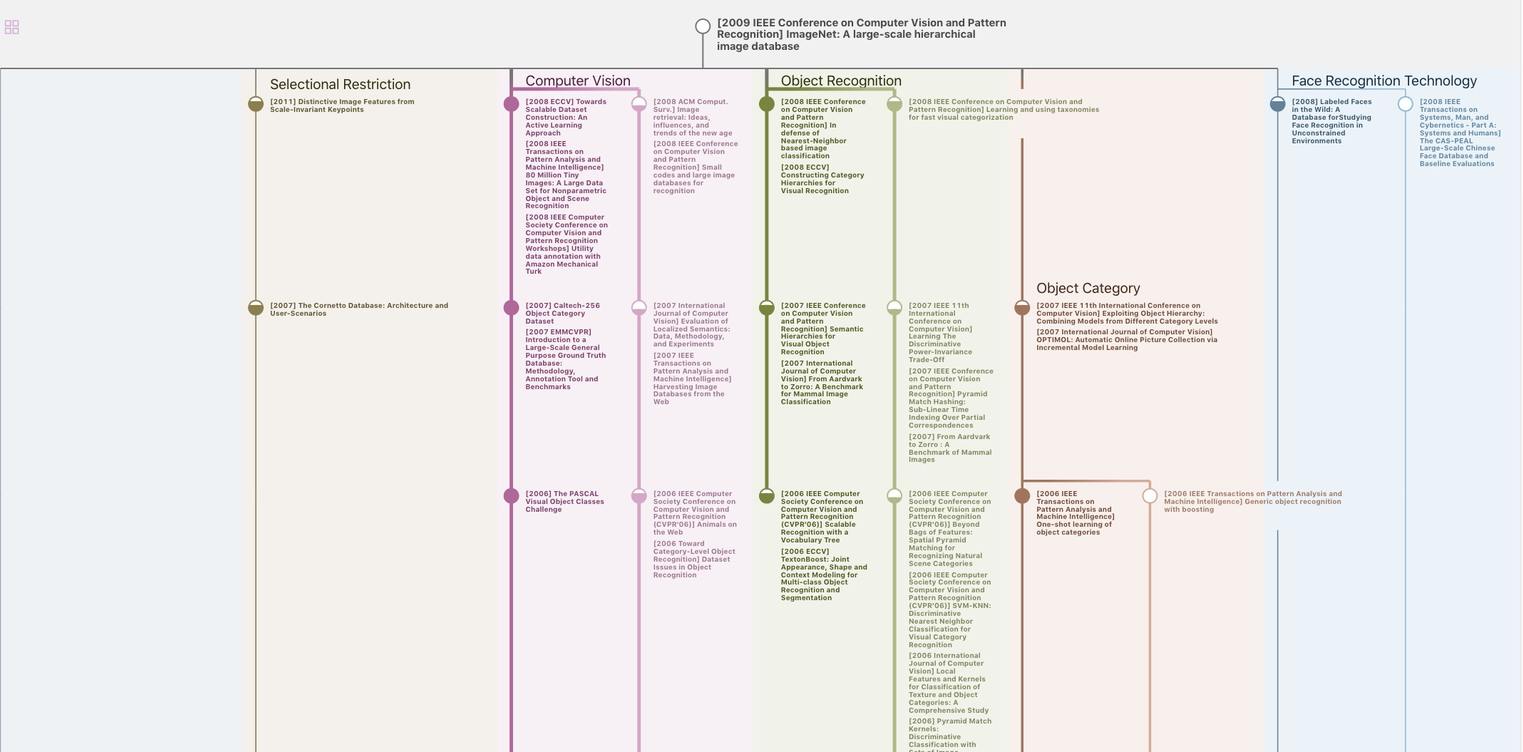
生成溯源树,研究论文发展脉络
Chat Paper
正在生成论文摘要