TSM: Three-Stream Mix For Unsupervised Medical Image Registration.
2023 IEEE International Conference on Bioinformatics and Biomedicine (BIBM)(2023)
摘要
Medical image registration is a crucial preprocessing step in medical image processing. Due to the potential impact of treatments and disease progression on patients' organ geometry, such as in magnetic resonance imaging (MRI) and computed tomography (CT) images, medical image registration is of significant importance for cancer diagnosis, treatment planning, and therapy. Existing methods usually adopt convolutional neural networks and Transformer frameworks, but still face challenges to effectively solve both tissue robustness and registration accuracy. Therefore, we propose TSM, a hybrid Transformer-Convolution model, for non-rigid registration of volumetric medical multi-tissue images. We perform dynamic global filtering convolution in the frequency domain and multi-scale parallel convolution, capturing local tissue structure information; meanwhile, we use a special attention combination mechanism to obtain semantic associations among tissue structures. We evaluate our method on the publicly available LPBA40 and EMPIRE10 challenge datasets. With comparison of the state-of-the-arts, we improve the dice score by 1.2% on the LPBA40 dataset and by 3% on the EMPIRE10 dataset, achieving the best registration results.
更多查看译文
关键词
Medical image registration,Transformer,Convolutional neural network
AI 理解论文
溯源树
样例
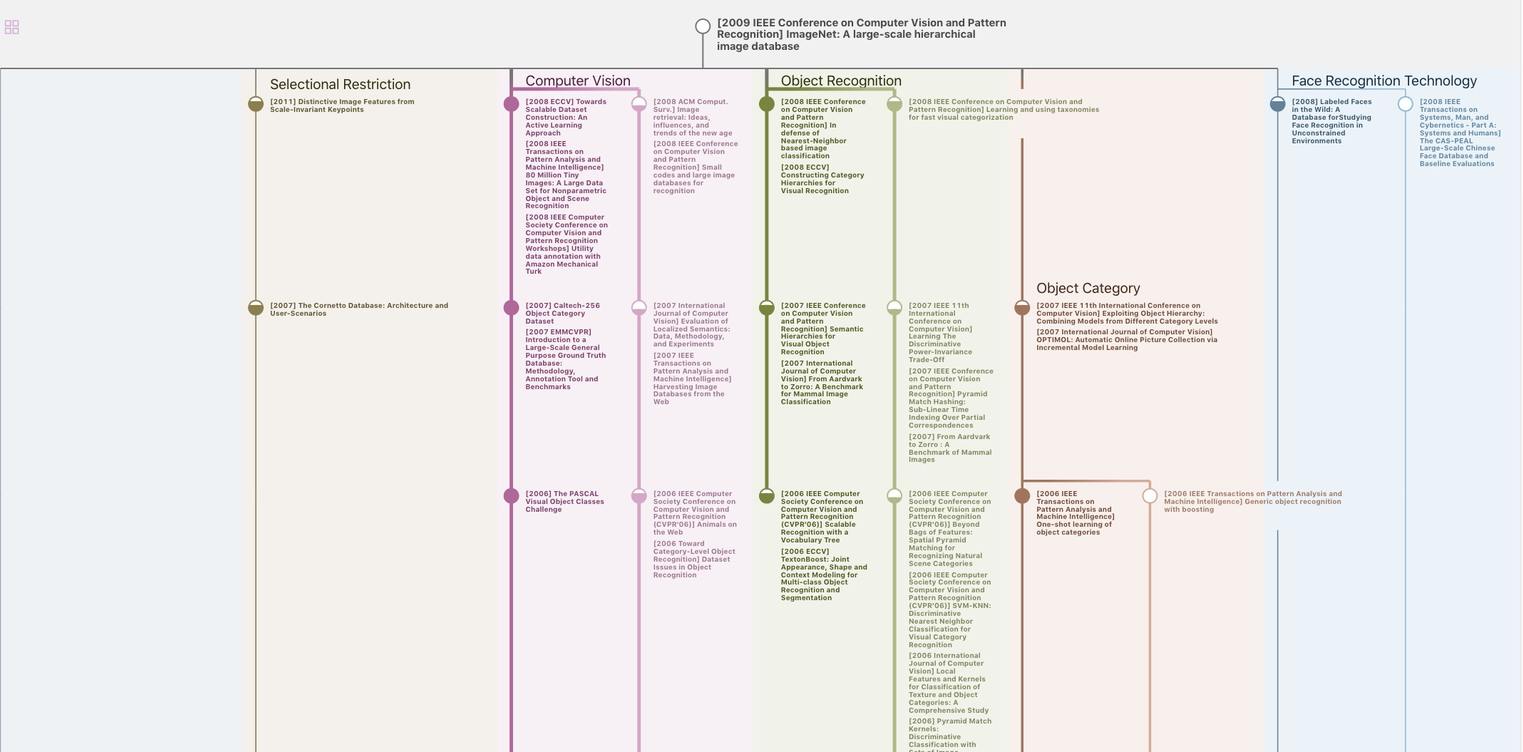
生成溯源树,研究论文发展脉络
Chat Paper
正在生成论文摘要