Predicting Treatment Outcomes in Obstructive Sleep Apnea: A Distribution-based Spectral Analysis of Low-Sampled Snoring Vibrations.
2023 IEEE Biomedical Circuits and Systems Conference (BioCAS)(2023)
摘要
Obstructive sleep apnea (OSA) is a prevalent condition characterized by complete (apnea) or partial (hypopnea) reductions in airflow due to a collapsed upper airway during sleep. Oral appliances, which reposition the lower jaw or tongue forward to maintain an open airway, are commonly used for OSA treatment. A major advantage of oral appliances over other treatment options such as positive airway pressure machines is a higher adherence to treatment. However, sleep physicians are hesitant to prescribe oral appliances since it is hard to predict who would respond to this treatment. Previous studies have explored invasive techniques like drug-induced sleep endoscopy (DISE) and awake nasendoscopy, as well as clinical tools such as polysomnography-based sleep phenotyping, to predict oral appliance treatment success. However, these methods require clinical expertise and are challenging for patients. In this study, we investigated the feasibility of using snoring vibrations recorded by a portable at-home OSA test device to predict the effectiveness of mandibular advancement devices, a common type of oral appliance. Snoring vibrations were recorded in 20 patients over a 5-month follow-up period. Our findings highlight that by utilizing distribution-based spectral features extracted solely from snoring vibrations and employing machine learning techniques along with feature selection methods, we can predict the efficacy of mandibular advancement device with an accuracy of 90% using leave-one-subject-out cross-validation. This approach offers a non-invasive and patient-friendly alternative for assessing the efficacy of oral appliances, which can aid sleep physicians in making informed treatment decisions.
更多查看译文
关键词
Sleep apnea,Oral appliances,Acoustic analysis,Snoring signal,Machine learning
AI 理解论文
溯源树
样例
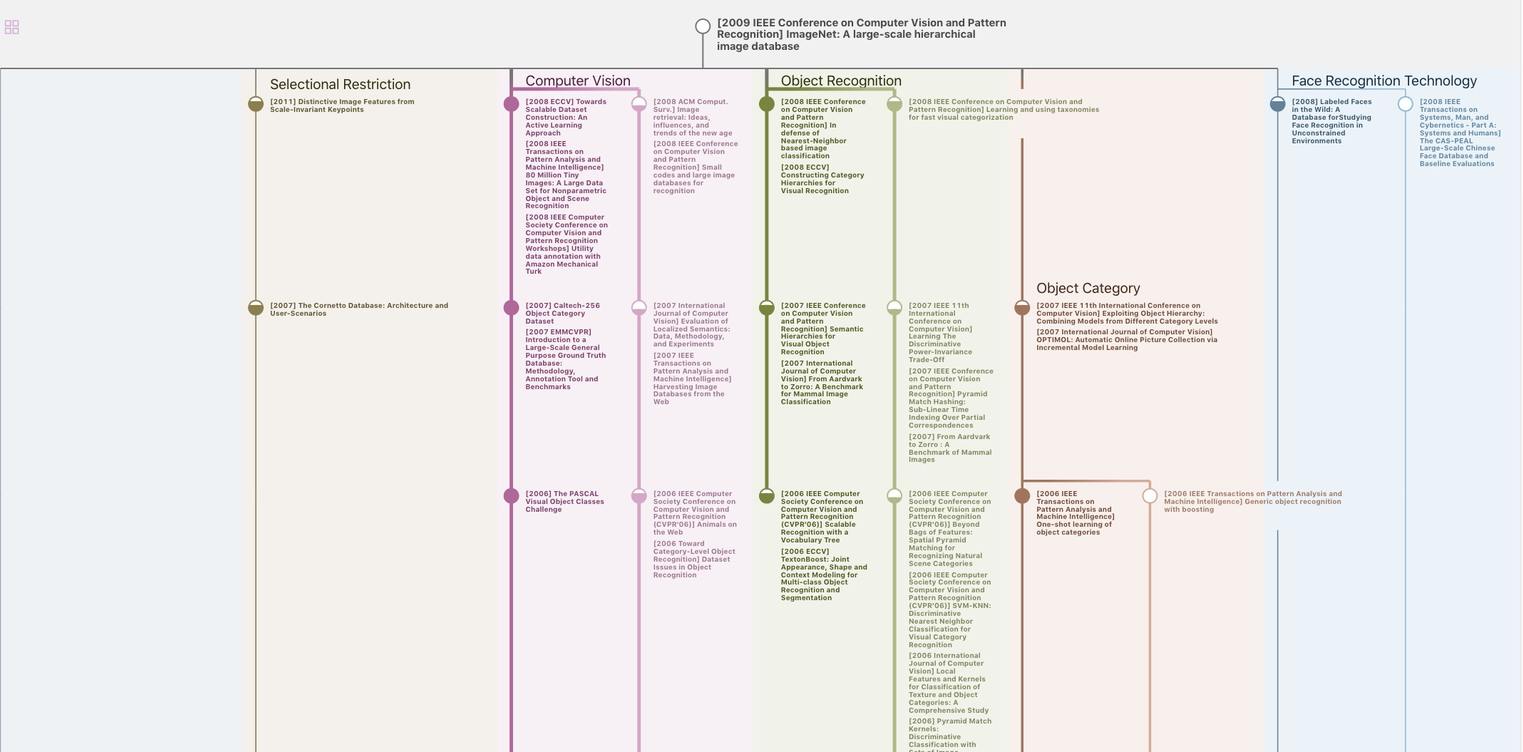
生成溯源树,研究论文发展脉络
Chat Paper
正在生成论文摘要