Through the Dual-Prism: A Spectral Perspective on Graph Data Augmentation for Graph Classification
CoRR(2024)
Abstract
Graph Neural Networks (GNNs) have become the preferred tool to process graph
data, with their efficacy being boosted through graph data augmentation
techniques. Despite the evolution of augmentation methods, issues like graph
property distortions and restricted structural changes persist. This leads to
the question: Is it possible to develop more property-conserving and
structure-sensitive augmentation methods? Through a spectral lens, we
investigate the interplay between graph properties, their augmentation, and
their spectral behavior, and found that keeping the low-frequency eigenvalues
unchanged can preserve the critical properties at a large scale when generating
augmented graphs. These observations inform our introduction of the Dual-Prism
(DP) augmentation method, comprising DP-Noise and DP-Mask, which adeptly
retains essential graph properties while diversifying augmented graphs.
Extensive experiments validate the efficiency of our approach, providing a new
and promising direction for graph data augmentation.
MoreTranslated text
AI Read Science
Must-Reading Tree
Example
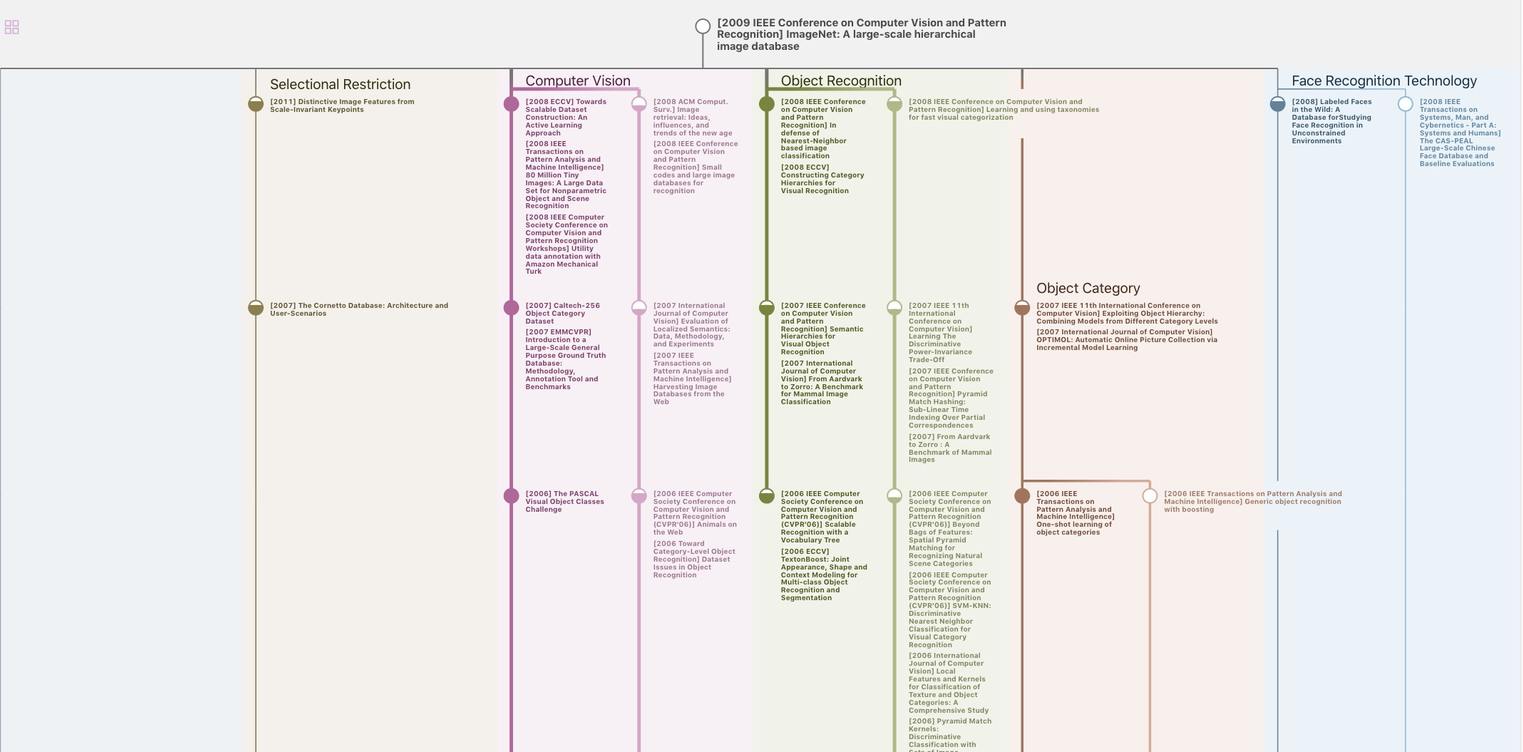
Generate MRT to find the research sequence of this paper
Chat Paper
Summary is being generated by the instructions you defined