Improving the Accuracy of Analog-Based In-Memory Computing Accelerators Post-Training
CoRR(2024)
摘要
Analog-Based In-Memory Computing (AIMC) inference accelerators can be used to
efficiently execute Deep Neural Network (DNN) inference workloads. However, to
mitigate accuracy losses, due to circuit and device non-idealities,
Hardware-Aware (HWA) training methodologies must be employed. These typically
require significant information about the underlying hardware. In this paper,
we propose two Post-Training (PT) optimization methods to improve accuracy
after training is performed. For each crossbar, the first optimizes the
conductance range of each column, and the second optimizes the input, i.e,
Digital-to-Analog Converter (DAC), range. It is demonstrated that, when these
methods are employed, the complexity during training, and the amount of
information about the underlying hardware can be reduced, with no notable
change in accuracy (≤0.1
transformer model for all General Language Understanding Evaluation (GLUE)
benchmark tasks. Additionally, it is demonstrated that further optimizing
learned parameters PT improves accuracy.
更多查看译文
AI 理解论文
溯源树
样例
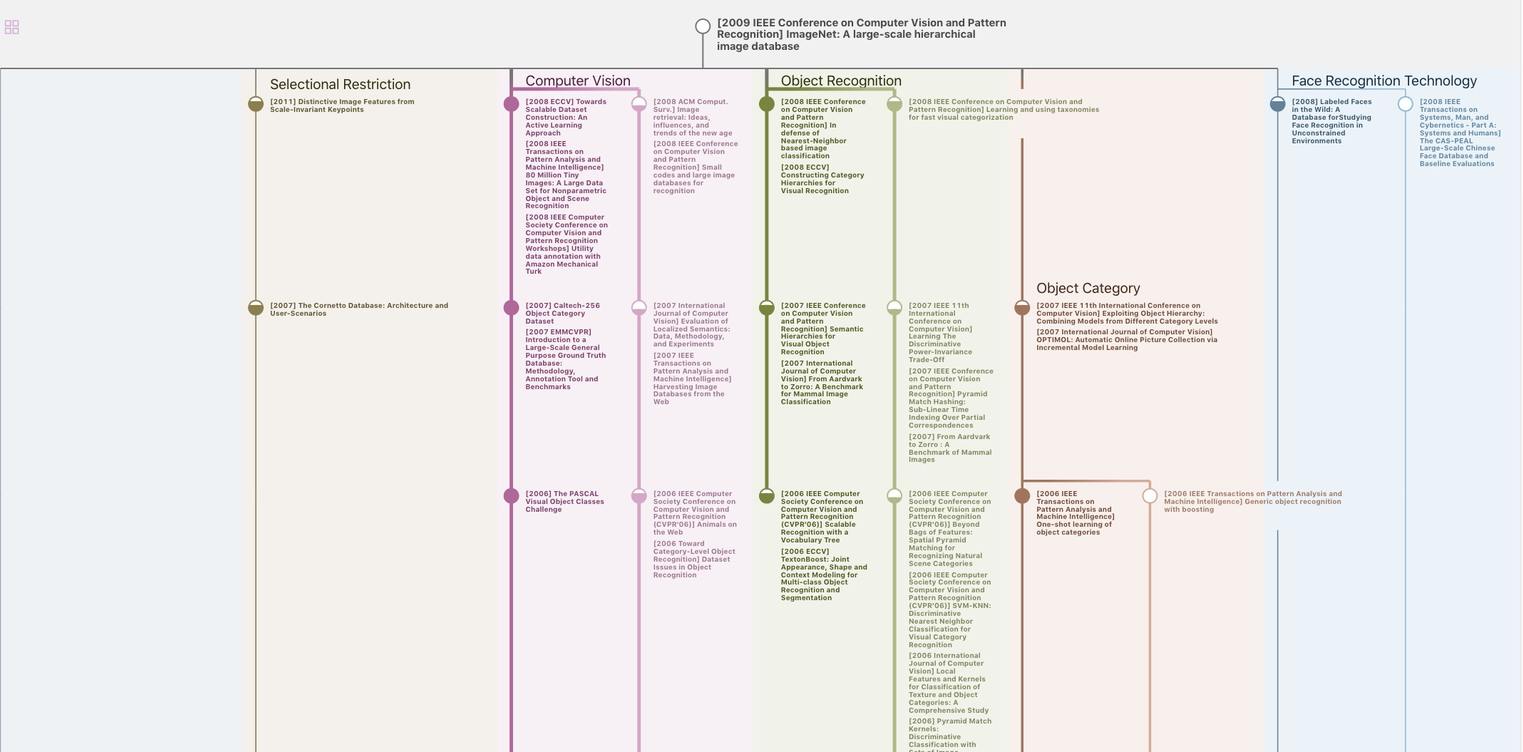
生成溯源树,研究论文发展脉络
Chat Paper
正在生成论文摘要