P2Seg: Pointly-supervised Segmentation via Mutual Distillation
CoRR(2024)
摘要
Point-level Supervised Instance Segmentation (PSIS) aims to enhance the
applicability and scalability of instance segmentation by utilizing low-cost
yet instance-informative annotations. Existing PSIS methods usually rely on
positional information to distinguish objects, but predicting precise
boundaries remains challenging due to the lack of contour annotations.
Nevertheless, weakly supervised semantic segmentation methods are proficient in
utilizing intra-class feature consistency to capture the boundary contours of
the same semantic regions. In this paper, we design a Mutual Distillation
Module (MDM) to leverage the complementary strengths of both instance position
and semantic information and achieve accurate instance-level object perception.
The MDM consists of Semantic to Instance (S2I) and Instance to Semantic (I2S).
S2I is guided by the precise boundaries of semantic regions to learn the
association between annotated points and instance contours. I2S leverages
discriminative relationships between instances to facilitate the
differentiation of various objects within the semantic map. Extensive
experiments substantiate the efficacy of MDM in fostering the synergy between
instance and semantic information, consequently improving the quality of
instance-level object representations. Our method achieves 55.7 mAP_50 and
17.6 mAP on the PASCAL VOC and MS COCO datasets, significantly outperforming
recent PSIS methods and several box-supervised instance segmentation
competitors.
更多查看译文
关键词
Mutual Distillation,Semantic to Instance,Instance to Semantic,Point-level Supervised Instance Segmentation
AI 理解论文
溯源树
样例
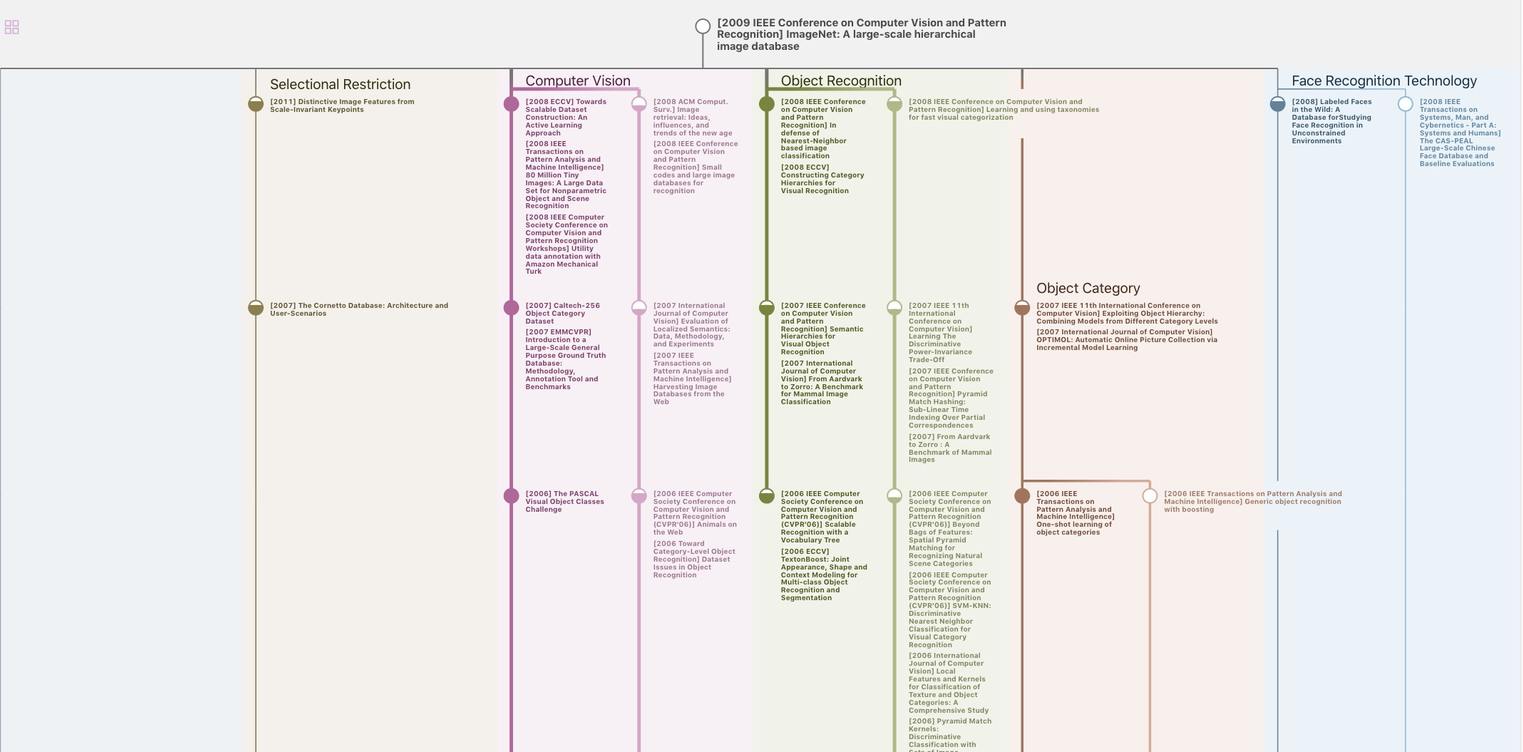
生成溯源树,研究论文发展脉络
Chat Paper
正在生成论文摘要