Large-scale Point Cloud Semantic Segmentation Via Local Perception and Global Descriptor Vector
EXPERT SYSTEMS WITH APPLICATIONS(2024)
Key words
Point cloud,Semantic segmentation,Large-scale scene,Local and global feature
AI Read Science
Must-Reading Tree
Example
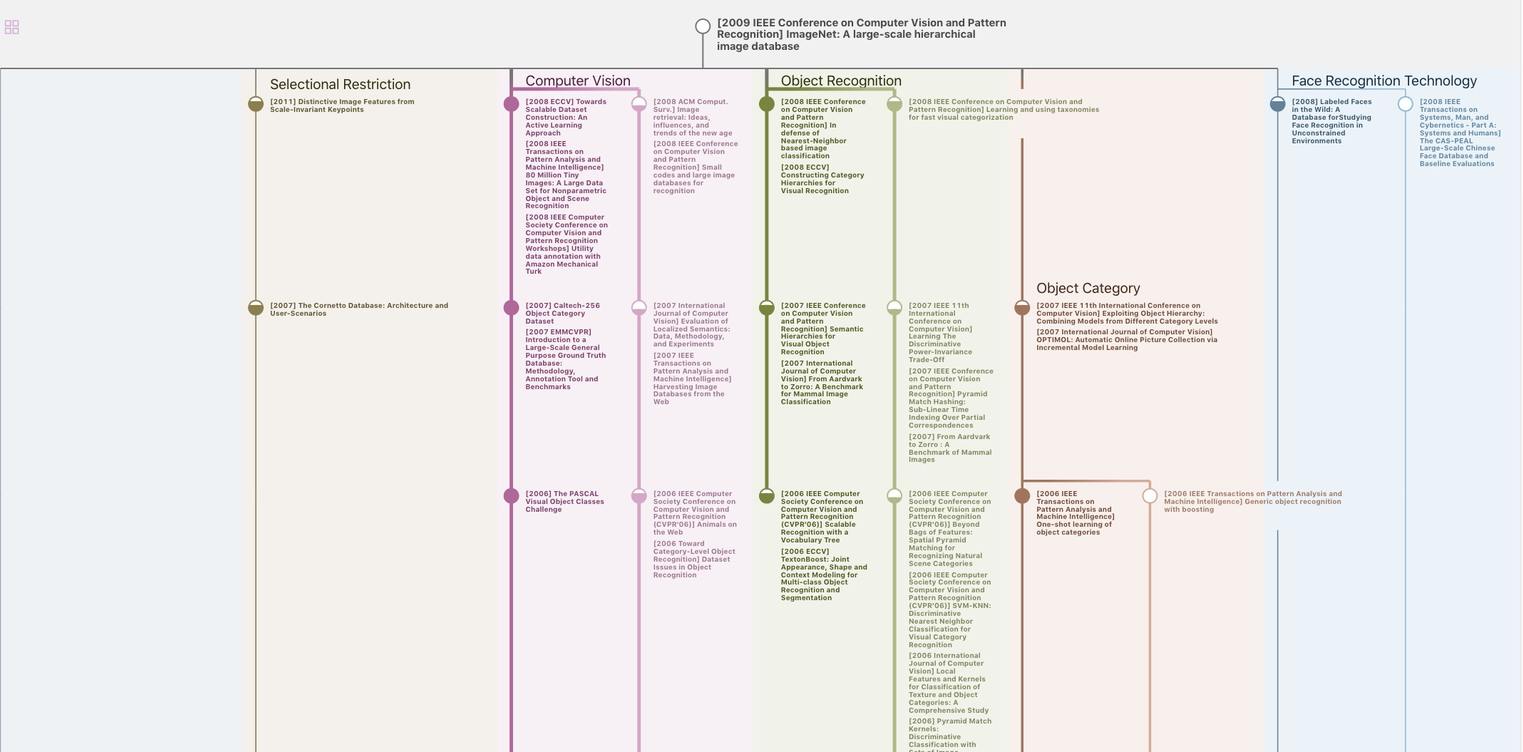
Generate MRT to find the research sequence of this paper
Chat Paper
Summary is being generated by the instructions you defined