A-XAI: adversarial machine learning for trustable explainability
AI and Ethics(2024)
Abstract
With the recent advancements in the usage of Artificial Intelligence (AI)-based systems in the healthcare and medical domain, it has become necessary to monitor whether these systems make predictions using the correct features or not. For this purpose, many different types of model interpretability and explainability methods are proposed in the literature. However, with the rising number of adversarial attacks against these AI-based systems, it also becomes necessary to make those systems more robust to adversarial attacks and validate the correctness of the generated model explainability. In this work, we first demonstrate how an adversarial attack can affect the model explainability even after robust training. Along with this, we present two different types of attack classifiers: one that can detect whether the given input is benign or adversarial and the other classifier that can identify the type of attack. We also identify the regions affected by the adversarial attack using model explainability. Finally, we demonstrate how the correctness of the generated explainability can be verified using model interpretability methods.
MoreTranslated text
Key words
Deep learning,Adversarial machine learning,Convolution neural network (CNN),Explainable AI,Model interpretability
AI Read Science
Must-Reading Tree
Example
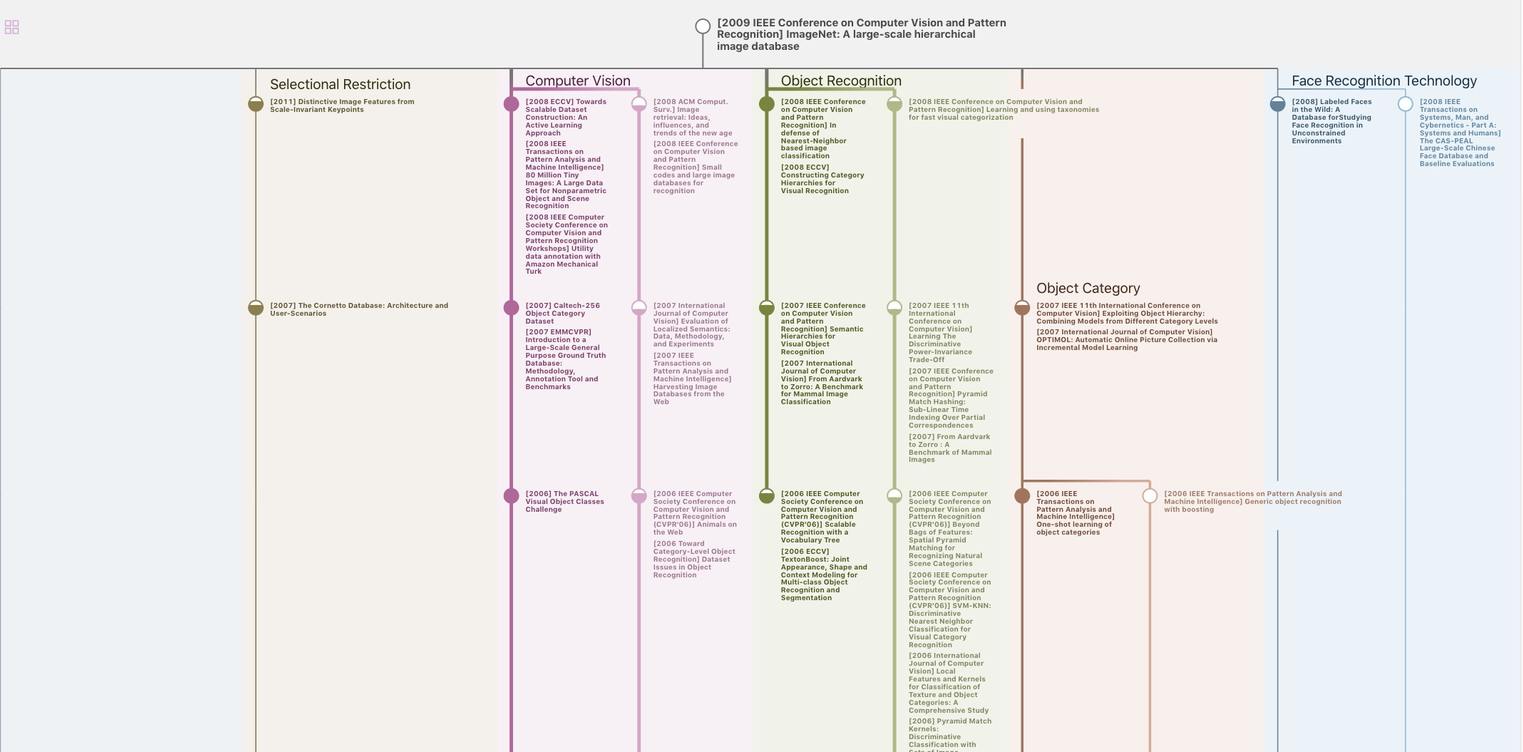
Generate MRT to find the research sequence of this paper
Chat Paper
Summary is being generated by the instructions you defined