Optimal scale selection approach for classification based on generalized multi-scale formal context
APPLIED SOFT COMPUTING(2024)
摘要
The classification of multi-scale data is an important research topic in granular computing. Its research goal is to determine the most appropriate scale and achieve better classification performance. However, determining the optimal scale is often a difficult problem due to lacking better metrics and optimization methods. In order to solve this problem, this paper proposes the optimal scale selection criteria for generalized multi-scale formal contexts. That is, the optimal scale uses the coarsest conditional attributes and finest decision attributes to optimize the combination of granularities of attributes. We combine these criteria with multi-objective optimization methods for developing an algorithm to fast compute the optimal scale. Experiments show that for the selected 14 data sets and 11 comparative classification methods, there are 9 classification methods with higher classification accuracies on more than 9 data sets. Therefore, the optimal scale selection method proposed in this paper is feasible and can effectively improve the performance of the classification method.
更多查看译文
关键词
Generalized multi-scale formal context,Optimal scale selection criteria,Multi-objective optimization methods,Classification
AI 理解论文
溯源树
样例
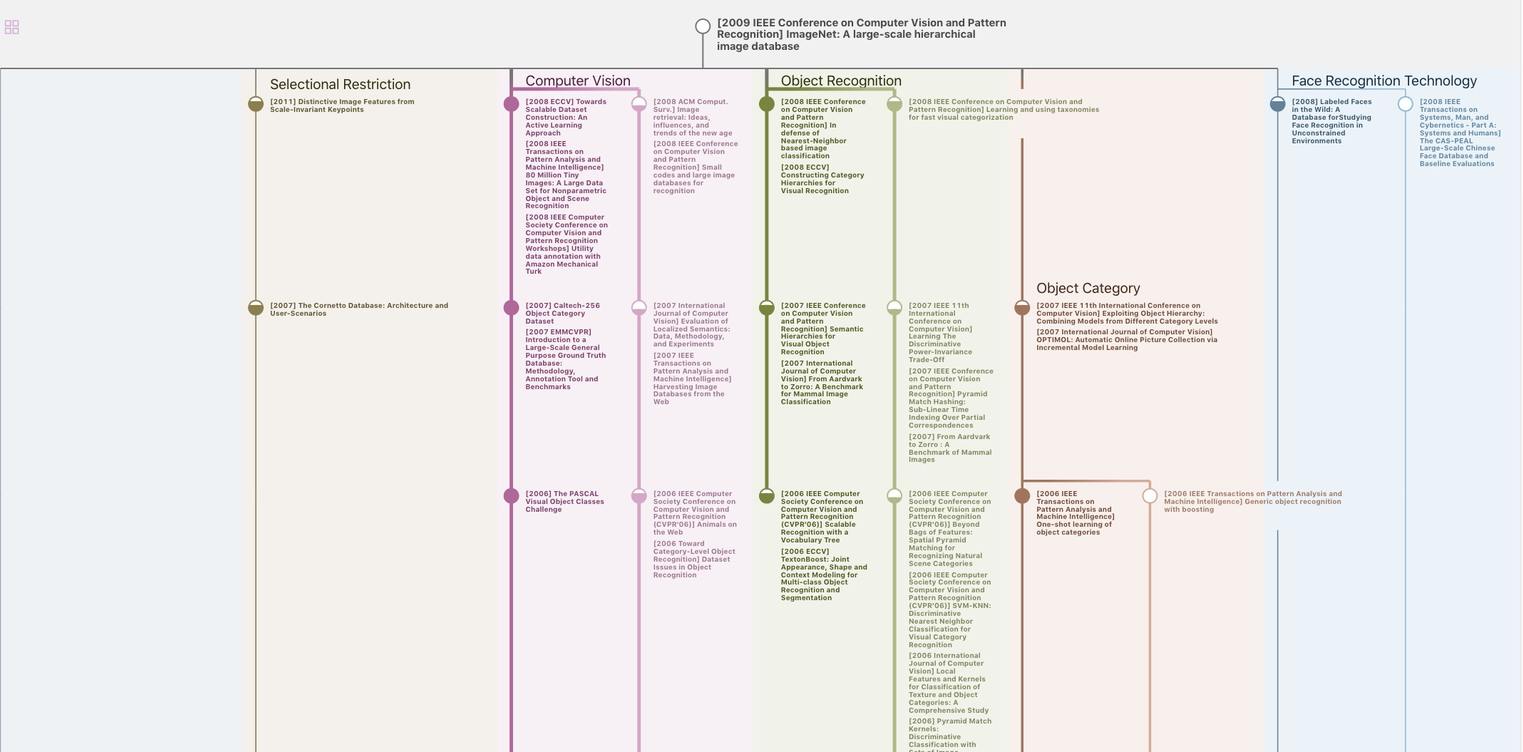
生成溯源树,研究论文发展脉络
Chat Paper
正在生成论文摘要