RevAP: A bankruptcy-based algorithm to solve the multi-agent credit assignment problem in task start threshold-based multi-agent systems
ROBOTICS AND AUTONOMOUS SYSTEMS(2024)
Abstract
Multi -Agent Systems (MASs) are the prominent symbol of Distributed Artificial Intelligence (DAI). Learning in MAS, which is commonly based on Reinforcement Learning (RL), is one of the problems that play an essential role in unknown environments. In this study aimed at solving the Multi -agent Credit Assignment (MCA) problem, we introduce the Task Start Threshold (TST) of agents as a new constraint in a multi -score operational environment, transforming the MCA into a bankruptcy problem. In the following, considering the bankruptcy concept, a new base algorithm, which is called Reverse Adjusted Proportional (RevAP), is introduced. Based on this algorithm, three methods PTST, T-MAS, and T-KAg, were presented to solve the MCA with different strategies. The proposed methods were evaluated in terms of group learning rate, confidence, expertness, certainty, efficiency, correctness, and density in comparison to the state-of-the-art methods such as ranking methods, dynamic, history -based as knowledge -based methods, Counterfactual Multi -Agent Policy Gradient (COMA) as an example of policy -based methods, Value -Decomposition Network (VDN) as an example of value -based methods, and Shapley Q- value Deep Deterministic Policy Gradient (SQDDPG) as a game theorybased method. The results reveal the better performance of the proposed approach compared to the existing methods based on the majority of the parameters.
MoreTranslated text
Key words
Multi-agent system,Credit assignment problem,Bankruptcy problem,Multi-agent reinforcement learning,Cooperative game theory
AI Read Science
Must-Reading Tree
Example
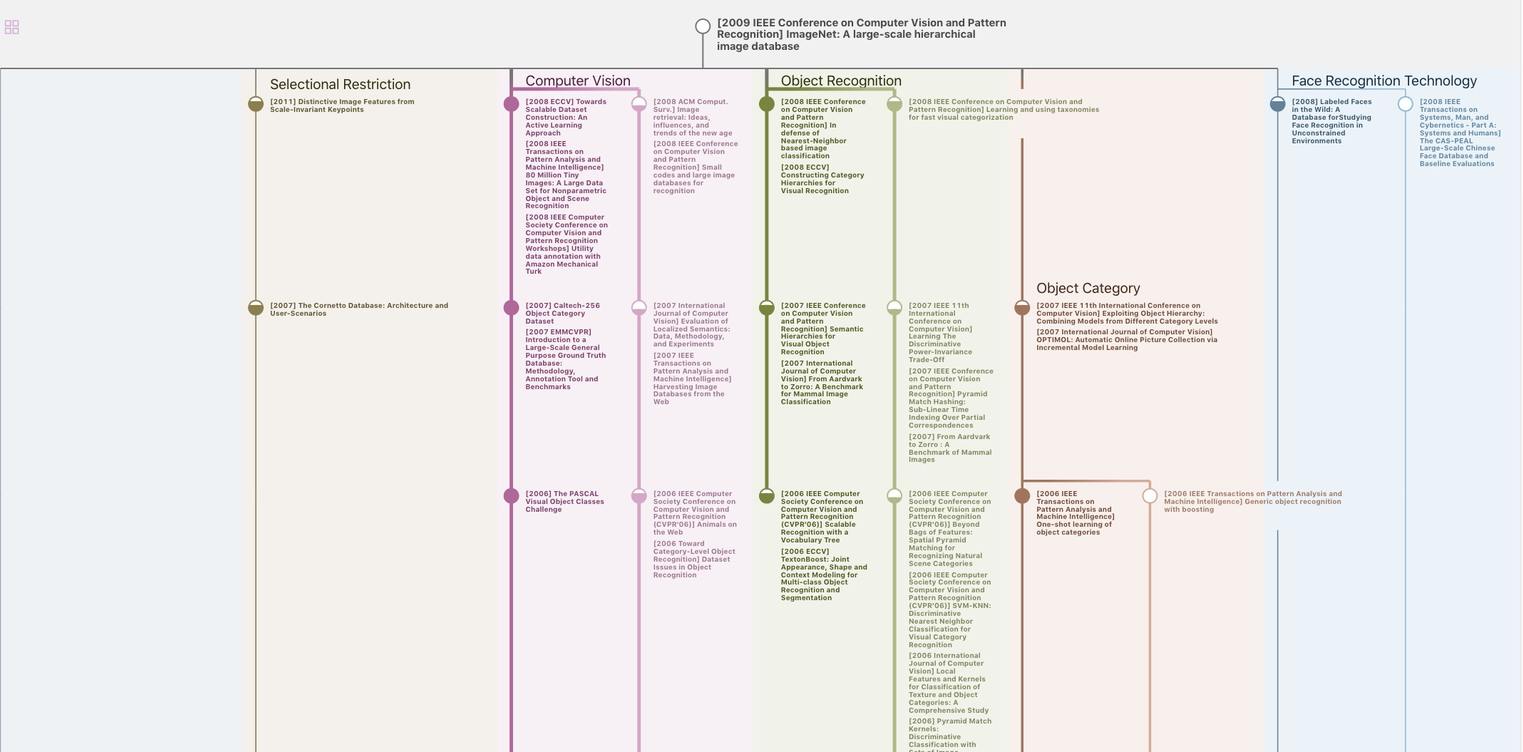
Generate MRT to find the research sequence of this paper
Chat Paper
Summary is being generated by the instructions you defined