GeMP-BNN: High-Performance Sampling-Free Bayesian Neural Network Accelerator with Gaussian Error Moment Propagation
2023 IEEE 16TH INTERNATIONAL SYMPOSIUM ON EMBEDDED MULTICORE/MANY-CORE SYSTEMS-ON-CHIP, MCSOC(2023)
摘要
Bayesian neural networks (BNNs), which can represent predictive uncertainty, have been an essential framework for reliable machine learning. Unfortunately, BNNs are difficult to use in applications requiring high-throughput processing, such as automated driving systems. This difficulty arises from the large amount of computation required, compared to that required for non-Bayesian neural networks. For example, Monte Carlo sampling-based BNNs require tens of forward propagations for prediction. Another approach, known as moment propagation, requires a lot of computations in the nonlinear layers, including exponential and square root functions, which are difficult to execute on hardware. Edge AI in IoT systems requires inference with limited computational resources, but the computation of such expensive functions results in increased circuit resources and increased computation. To realize high-throughput uncertainty estimation with limited computational resources, a hardware-friendly nonlinear layer and a dedicated hardware accelerator that can efficiently execute moment propagation are necessary. This study proposes a novel nonlinear layer, Gaussian error moment propagation (GeMP), which introduces nonlinearity and perturbations with less computational cost and no additional parameters and develops a dedicated FPGA-based accelerator utilizing the proposed nonlinear layer. Through evaluations with software and FPGA implementation, we showed that GeMP-BNN achieved up to 4.9 times higher throughput and 0.20 times lower energy consumption without compromising the uncertainty quality compared with BNN accelerators having previous nonlinear layers.
更多查看译文
关键词
Bayesian neural network,FPGA accelerator,Hardware-algorithm co-design,Moment propagation,Nonlinear layer
AI 理解论文
溯源树
样例
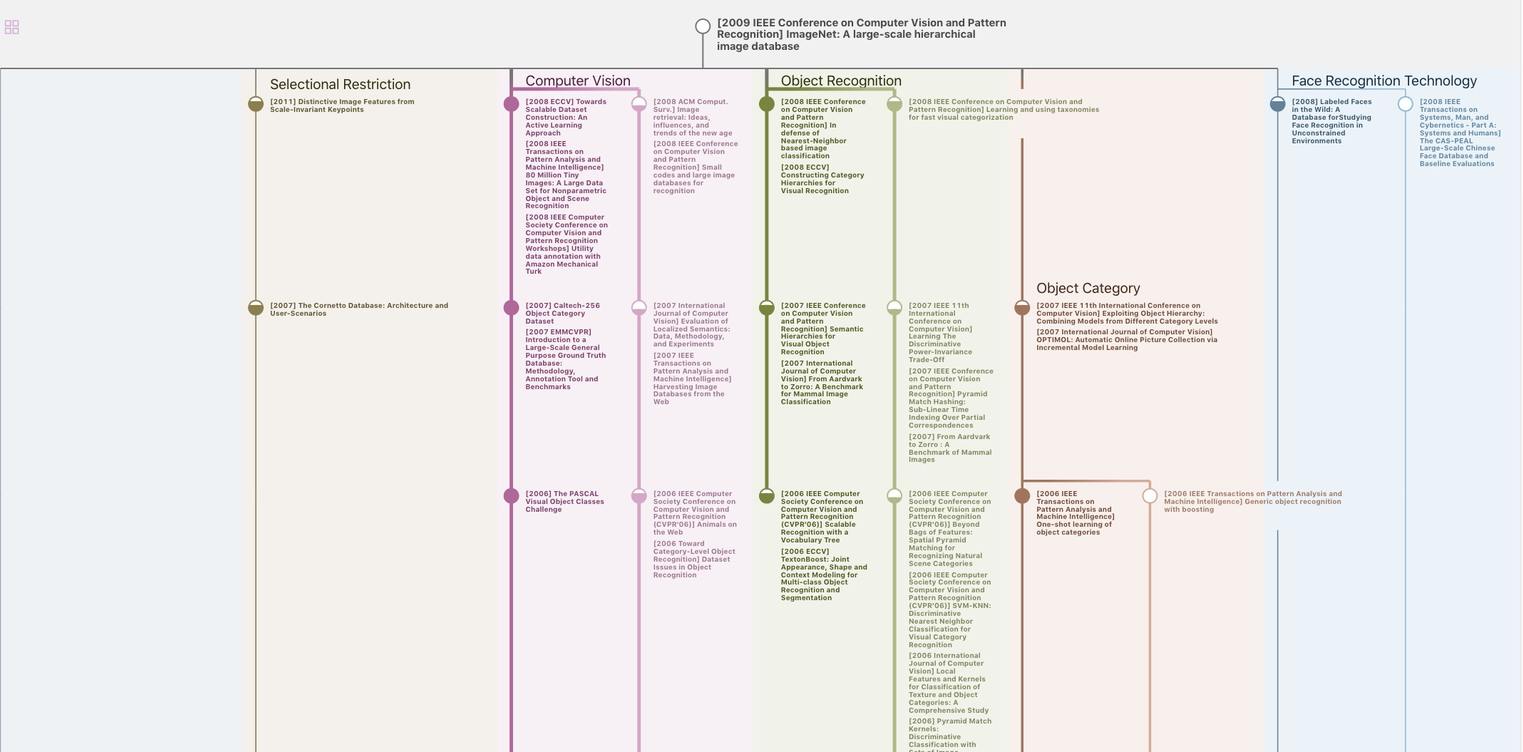
生成溯源树,研究论文发展脉络
Chat Paper
正在生成论文摘要