Explainable Multiscale Kernel Depth-wise Separable Convolutional Framework with Attention for COVID-19 Prediction from Chest X-ray
2023 7th International Symposium on Innovative Approaches in Smart Technologies (ISAS)(2023)
摘要
There is an urgent demand for lightweight deep learning models applicable to real-world scenarios. This research proposes an innovative XAI model that integrates an attention mechanism with multiscale kernel depth-wise separable convolution designed for COVID-19 classification using chest X-rays. The model consists of four sequential blocks, incorporating multiscale kernel attention depth-wise separable convolution (MKnADSC) modules. Notably, this model achieves an impressive accuracy of 96.50%, boasting a compact structure with 2.56 million parameters and FLOPs with 0.41 G. These findings suggest its significant practicality for real-world implementation, addressing the pressing need for efficient and accessible diagnostic tools during pandemics.
更多查看译文
关键词
Attention mechanism,COVID-19 diagnosis,Depth-wise separable convolution,Artificial intelligence
AI 理解论文
溯源树
样例
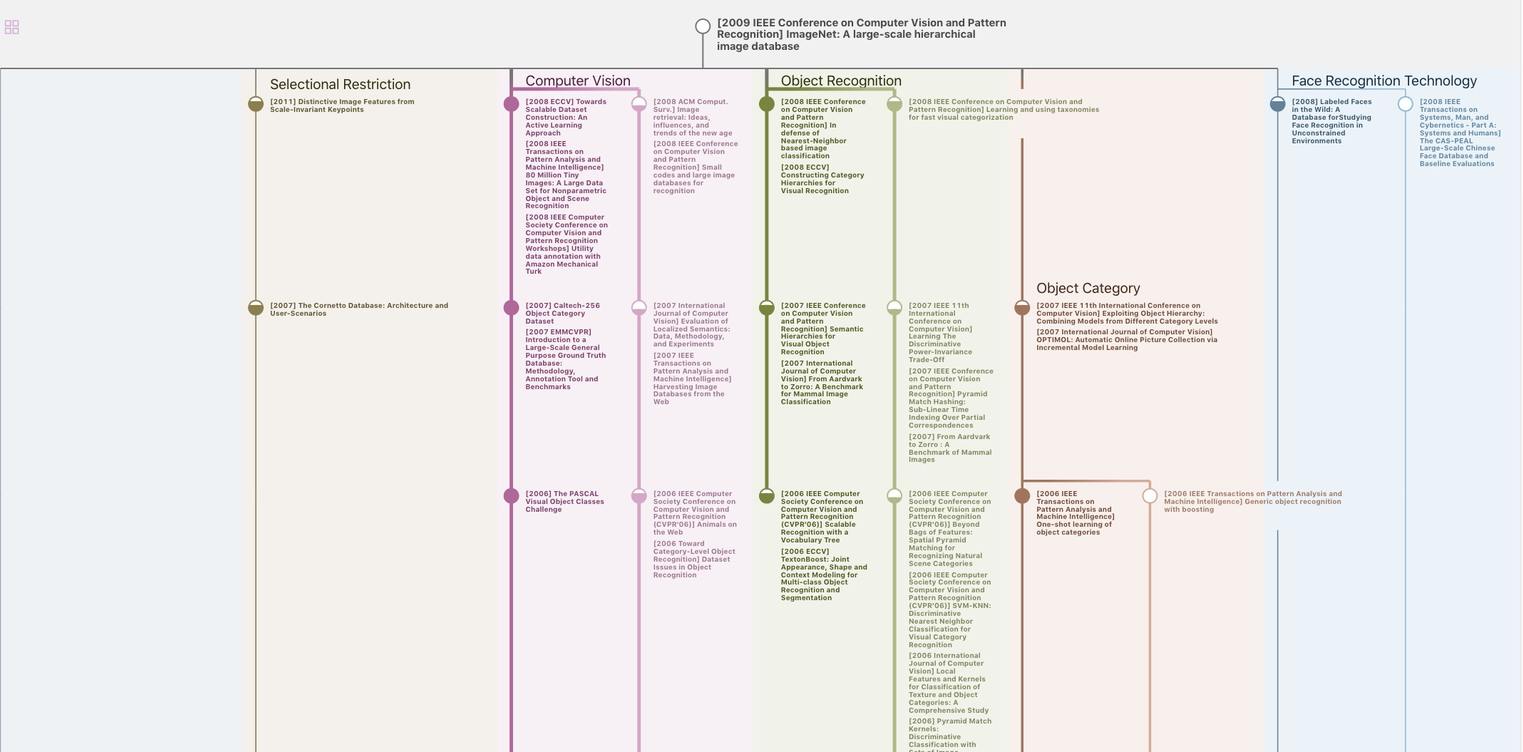
生成溯源树,研究论文发展脉络
Chat Paper
正在生成论文摘要