Deep Learning for Automatic Wafer Monitoring System
2023 Smart Systems Integration Conference and Exhibition (SSI)(2023)
摘要
Modern semiconductor manufacturing production lines include several technological processes such as diffusion, wafer cleaning and epitaxy and therefore they are subject to degradation phenomena that must be carefully monitored. The processed semiconductor wafer consequently requires a very accurate testing phase which allows to detect the presence of defects to be traced back to the production line and which can have an impact on the downstream yield. The electric wafer testing phase (EWS i.e. Electrical Wafer Sorting) is certainly one of the key stages in semiconductor wafer monitoring in both classical silicon-based technology and modern silicon carbide production lines. The EWS validation phase includes the execution of several electrical tests directly on the integrated circuits (dies) over the wafer. The EWS electrical assessment outcome can be reported in a 2D binary image-map enabling the formation of specific visual patterns in accordance with the defect statistics found in the testing phase. Specifically, from this intelligent EWS analysis it is possible to early-assess defects in the production lines, electrical defects in the devices and finally a proper wafer-yield forecasting estimation. The classification of the EWS image-map visual patterns is a very challenging and time-consuming task. In that context, ad-hoc deep learning solution has been developed in order to perform supervised/unsupervised EWS image-maps patterns recognition and tracking. The related pipeline is composed by ad-hoc convolutional deep architecture with an innovative augmentation system based on spatiotemporal preprocessing of input EWS image-maps. The implemented architecture is able to classify the input EWS binary maps into a well-known pattern-classes whose features at the production line level and the impact on yield are known. The designed deep network is able to identify new EWS patterns not included in the already known classes, so as to address the production-engineer accordingly. The proposed pipeline shows very promising results as it is able to classify the tested EWS with an average accuracy close to 97%.
更多查看译文
关键词
EWS,CNN,Clustering
AI 理解论文
溯源树
样例
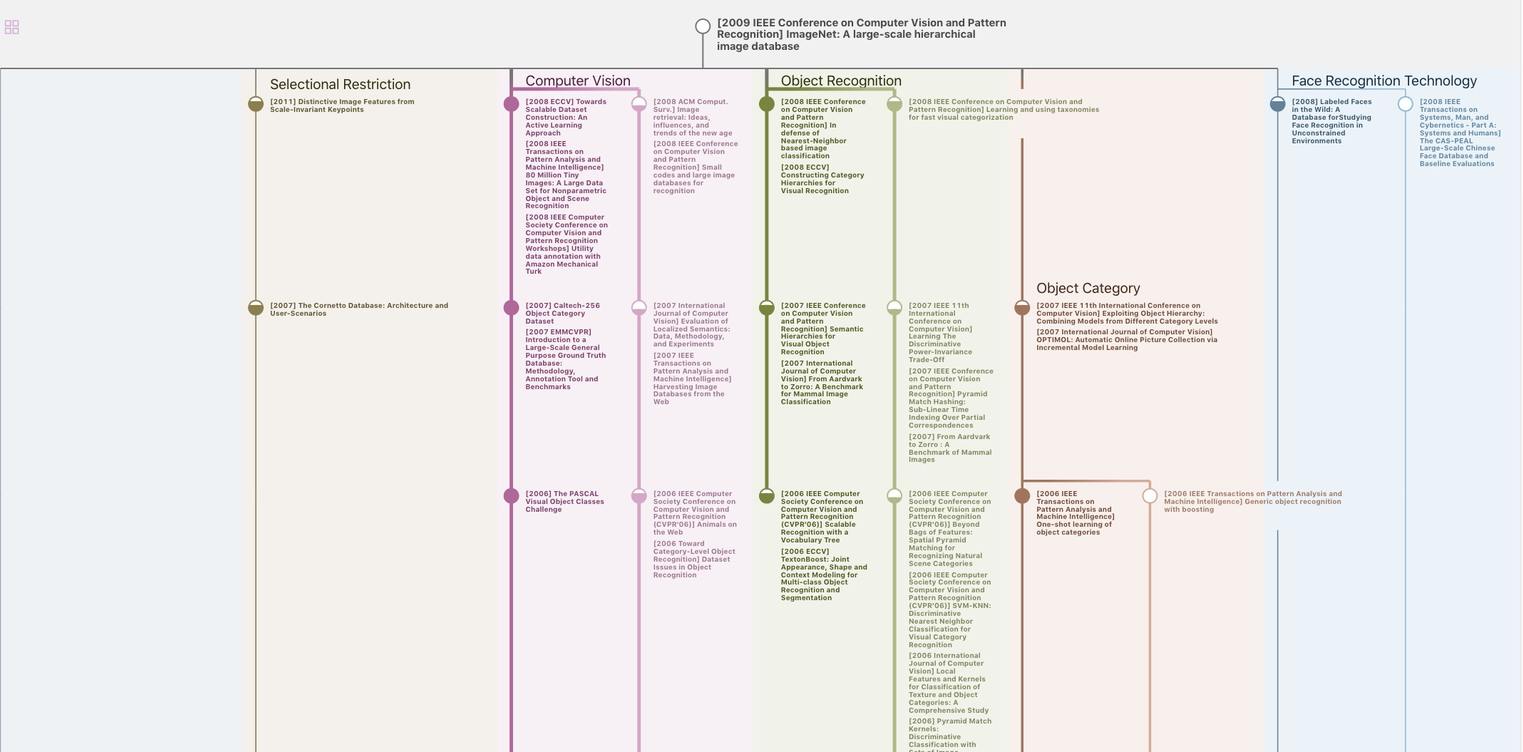
生成溯源树,研究论文发展脉络
Chat Paper
正在生成论文摘要