OPIT: A Simple but Effective Method for Sparse Subspace Tracking in High-Dimension and Low-Sample-Size Context
IEEE Transactions on Signal Processing(2024)
摘要
In recent years, sparse subspace tracking has attracted increasing attention in the signal processing community. In this paper, we propose a new provable effective method called OPIT (which stands for Online Power Iteration via Thresholding) for tracking the sparse principal subspace of data streams over time. Particularly, OPIT introduces a new adaptive variant of power iteration with space and computational complexity linear to the data dimension. In addition, a new column-based thresholding operator is developed to regularize the subspace sparsity. Utilizing both advantages of power iteration and thresholding operation, OPIT is capable of tracking the underlying subspace in both the classical regime and high dimensional regime. We also present a theoretical result on its convergence to verify its consistency in high dimensions. Several experiments are carried out on both synthetic and real data to demonstrate the performance of OPIT.
更多查看译文
关键词
Sparse subspace tracking,data streams,high dimensions,thresholding,power iteration
AI 理解论文
溯源树
样例
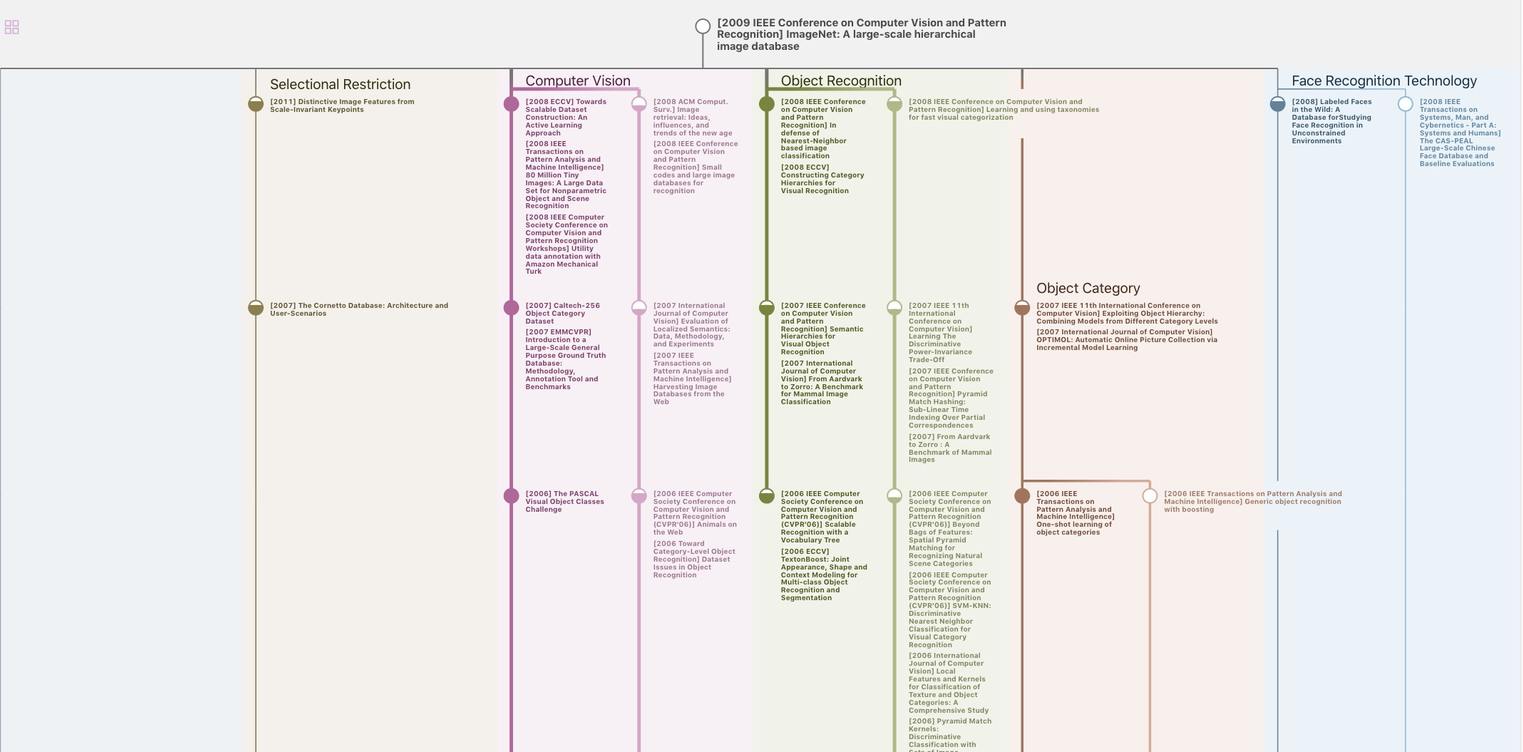
生成溯源树,研究论文发展脉络
Chat Paper
正在生成论文摘要